Learning From Architectural Redundancy: Enhanced Deep Supervision in Deep Multipath Encoder–Decoder Networks
IEEE Transactions on Neural Networks and Learning Systems(2022)
摘要
Deep encoder–decoders are the model of choice for pixel-level estimation due to their redundant deep architectures. Yet they still suffer from the vanishing supervision information issue that affects convergence because of their overly deep architectures. In this work, we propose and theoretically derive an enhanced deep supervision (EDS) method which improves on conventional deep supervision (DS) by incorporating variance minimization into the optimization. A new structure variance loss is introduced to build a bridge between deep encoder–decoders and variance minimization, and provides a new way to minimize the variance by forcing different intermediate decoding outputs (paths) to reach an agreement. We also design a focal weighting strategy to effectively combine multiple losses in a scale-balanced way, so that the supervision information is sufficiently enforced throughout the encoder–decoders. To evaluate the proposed method on the pixel-level estimation task, a novel multipath residual encoder is proposed and extensive experiments are conducted on four challenging density estimation and crowd counting benchmarks. The experimental results demonstrate the superiority of our EDS over other paradigms, and improved estimation performance is reported using our deeply supervised encoder–decoder.
更多查看译文
关键词
Convolutional neural networks (CNN),crowd counting,deep supervised learning,encoder–decoder networks
AI 理解论文
溯源树
样例
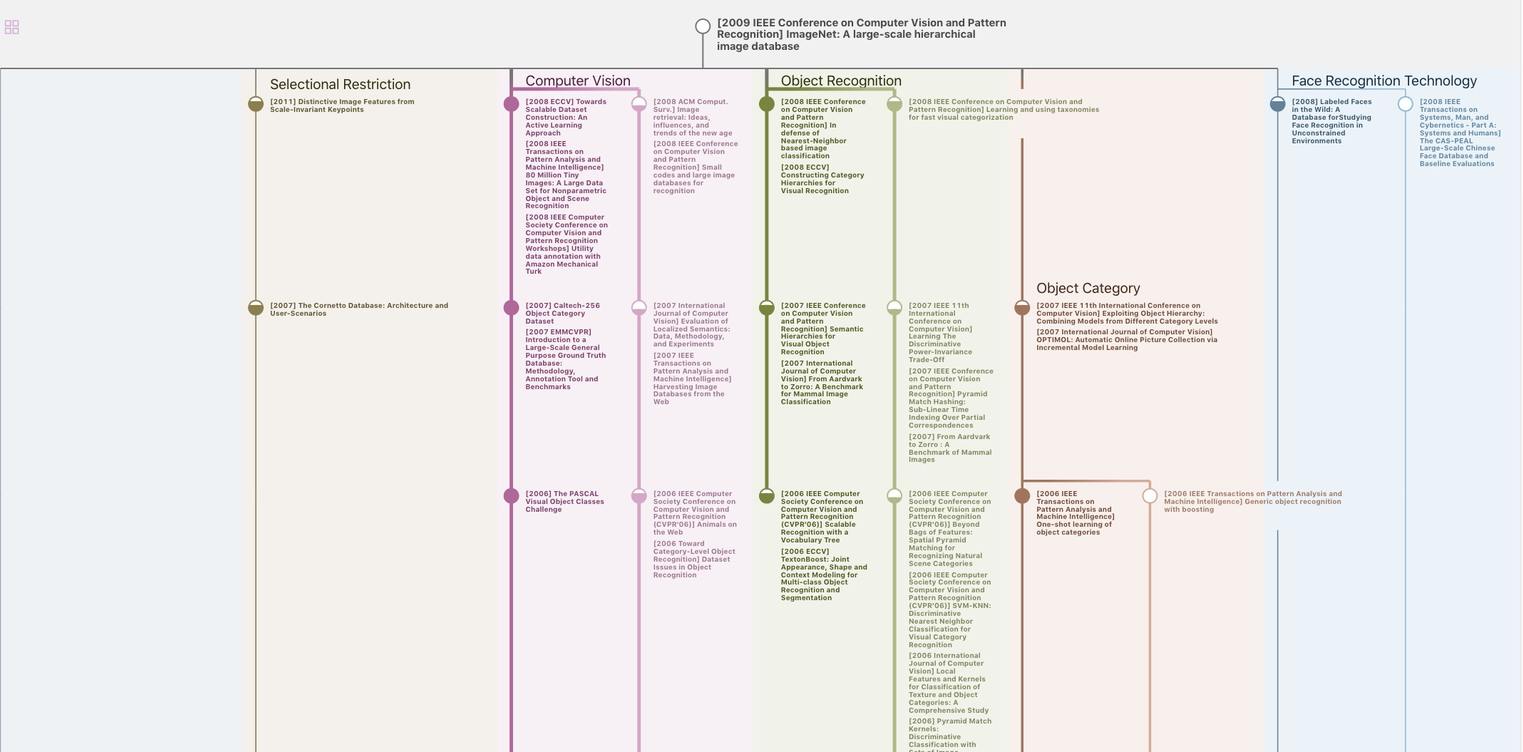
生成溯源树,研究论文发展脉络
Chat Paper
正在生成论文摘要