Making Intelligent Reflecting Surfaces More Intelligent: A Roadmap Through Reservoir Computing
IEEE Network(2022)
摘要
This article introduces a neural network-based signal processing framework for intelligent Reflecting surface-aided (IRS) wireless communications systems. By modeling radio frequency (RF) impairments inside the meta-atoms of IRS - including nonlinearity and memory effects) - we present an approach that generalizes the entire IRS-aided system as a reservoir computing (RC) system, an efficient recurrent neural network (RNN) operating in a state near the “edge of chaos.” This framework enables us to take advantage of the nonlinearity of this “fabricated” wireless environment to overcome link degradation due to model mismatch. Accordingly, the randomness of the wireless channel and RF imperfections are naturally embedded into the RC framework, enabling the internal RC dynamics lying on the edge of chaos. Furthermore, several practical issues, such as channel state information acquisition, passive beamforming design, and physical layer reference signal design, are discussed.
更多查看译文
关键词
RF,meta-atoms,including nonlinearity,memory effects,entire IRS-aided system,reservoir computing system,efficient recurrent neural network,fabricated wireless environment,wireless channel,RC framework,internal RC dynamics,channel state information acquisition,physical layer reference signal design,intelligent Reflecting surfaces,neural network-based signal,radio frequency,intelligent Reflecting surface-aided wireless communications systems
AI 理解论文
溯源树
样例
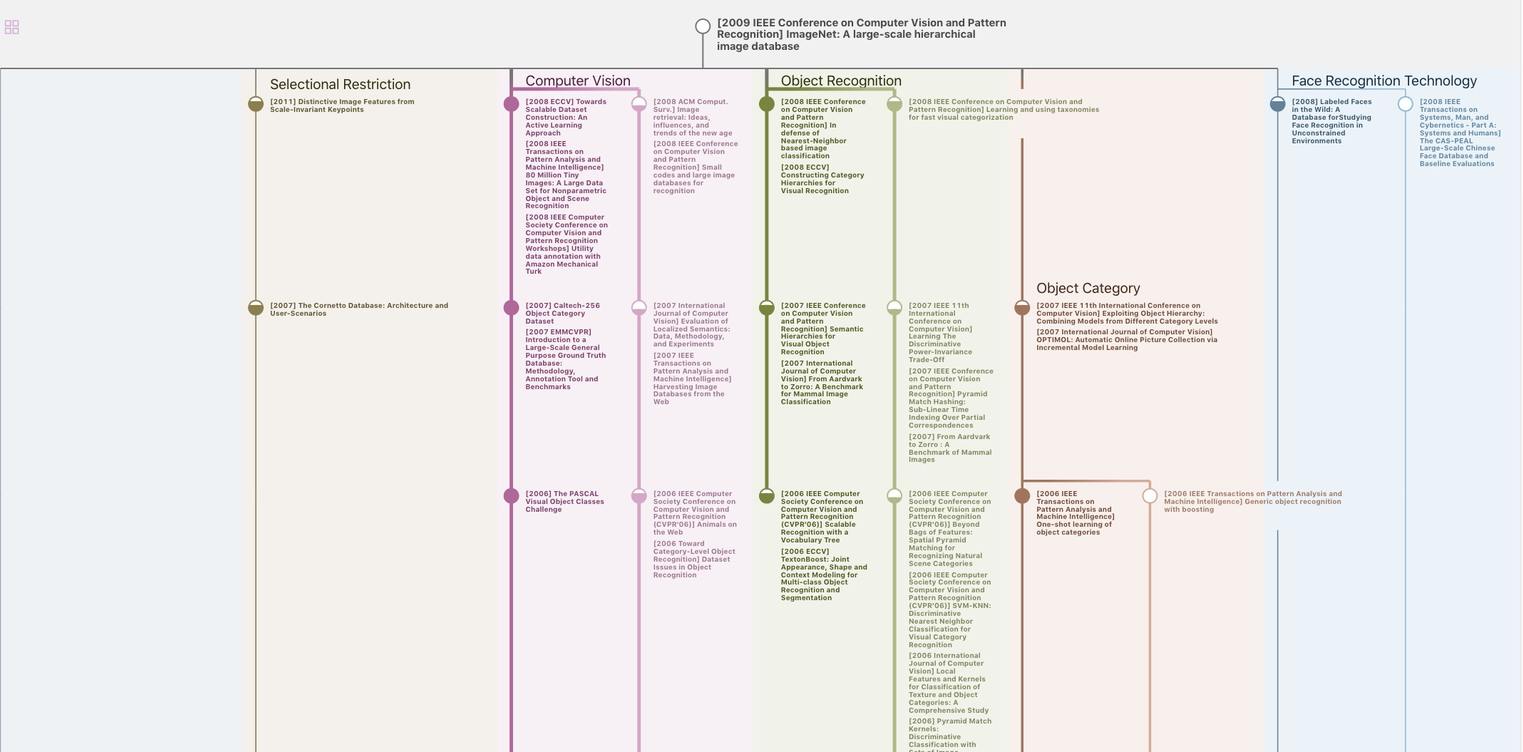
生成溯源树,研究论文发展脉络
Chat Paper
正在生成论文摘要