Object and Relation Centric Representations for Push Effect Prediction
Robotics and Autonomous Systems(2024)
Key words
Push manipulation,Effect prediction,Parameter estimation,Graph neural networks,Interactive perception,Articulation prediction
AI Read Science
Must-Reading Tree
Example
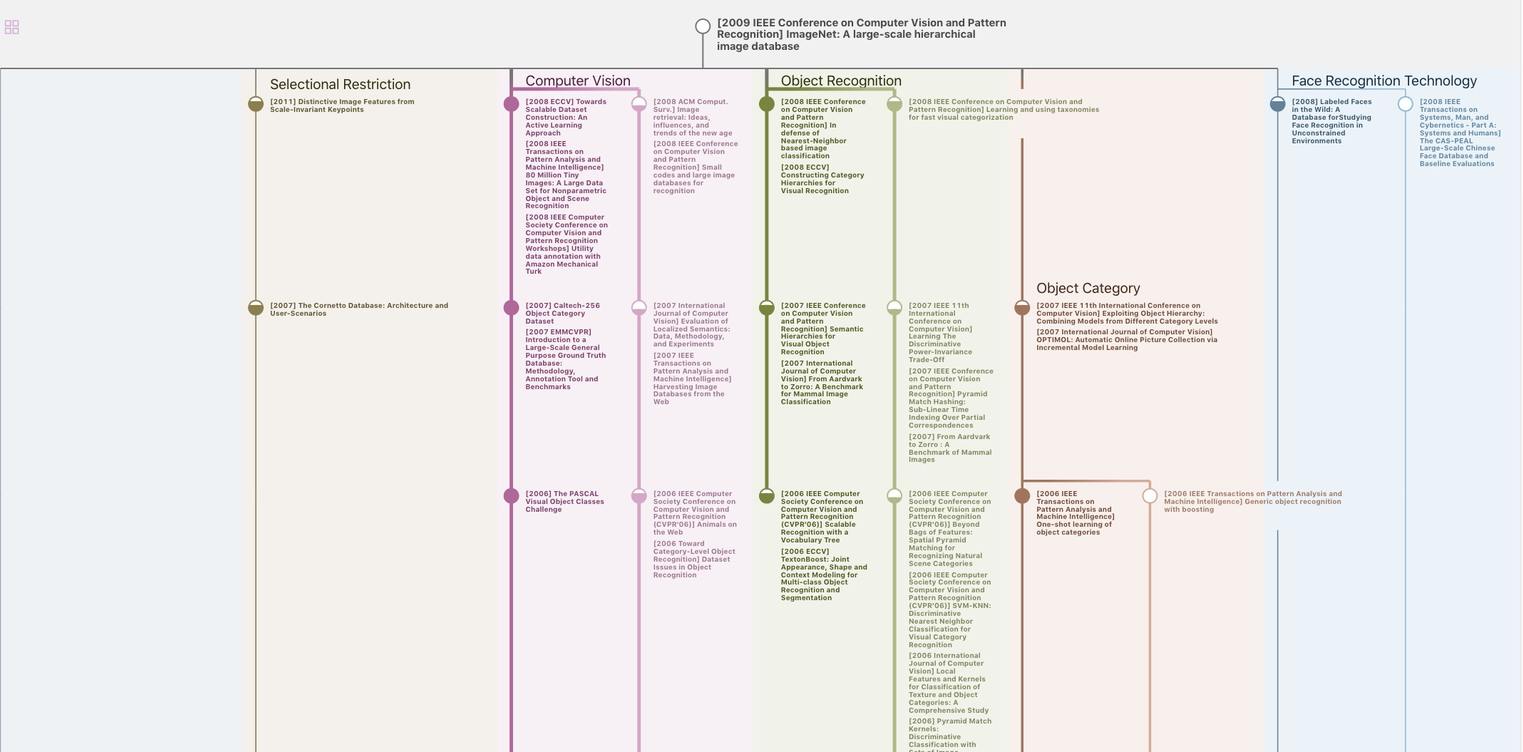
Generate MRT to find the research sequence of this paper
Chat Paper
Summary is being generated by the instructions you defined