Simple and Effective Approaches for Uncertainty Prediction in Facial Action Unit Intensity Regression
2020 15th IEEE International Conference on Automatic Face and Gesture Recognition (FG 2020)(2020)
摘要
Knowing how much to trust a prediction is important for many critical applications. We describe two simple approaches to estimate uncertainty in regression prediction tasks and compare their performance and complexity against popular approaches. We operationalize uncertainty in regression as the absolute error between a model's prediction and the ground truth. Our two proposed approaches use a secondary model to predict the uncertainty of a primary predictive model. Our first approach leverages the assumption that similar observations are likely to have similar uncertainty and predicts uncertainty with a non-parametric method. Our second approach trains a secondary model to directly predict the uncertainty of the primary predictive model. Both approaches outperform other established uncertainty estimation approaches on the MNIST, DISFA, and BP4D+datasets. Furthermore, we observe that approaches that directly predict the uncertainty generally perform better than approaches that indirectly estimate uncertainty.
更多查看译文
关键词
uncertainty prediction,facial action unit intensity regression,critical applications,simple approaches,regression prediction tasks,secondary model,primary predictive model,similar uncertainty,uncertainty estimation approaches
AI 理解论文
溯源树
样例
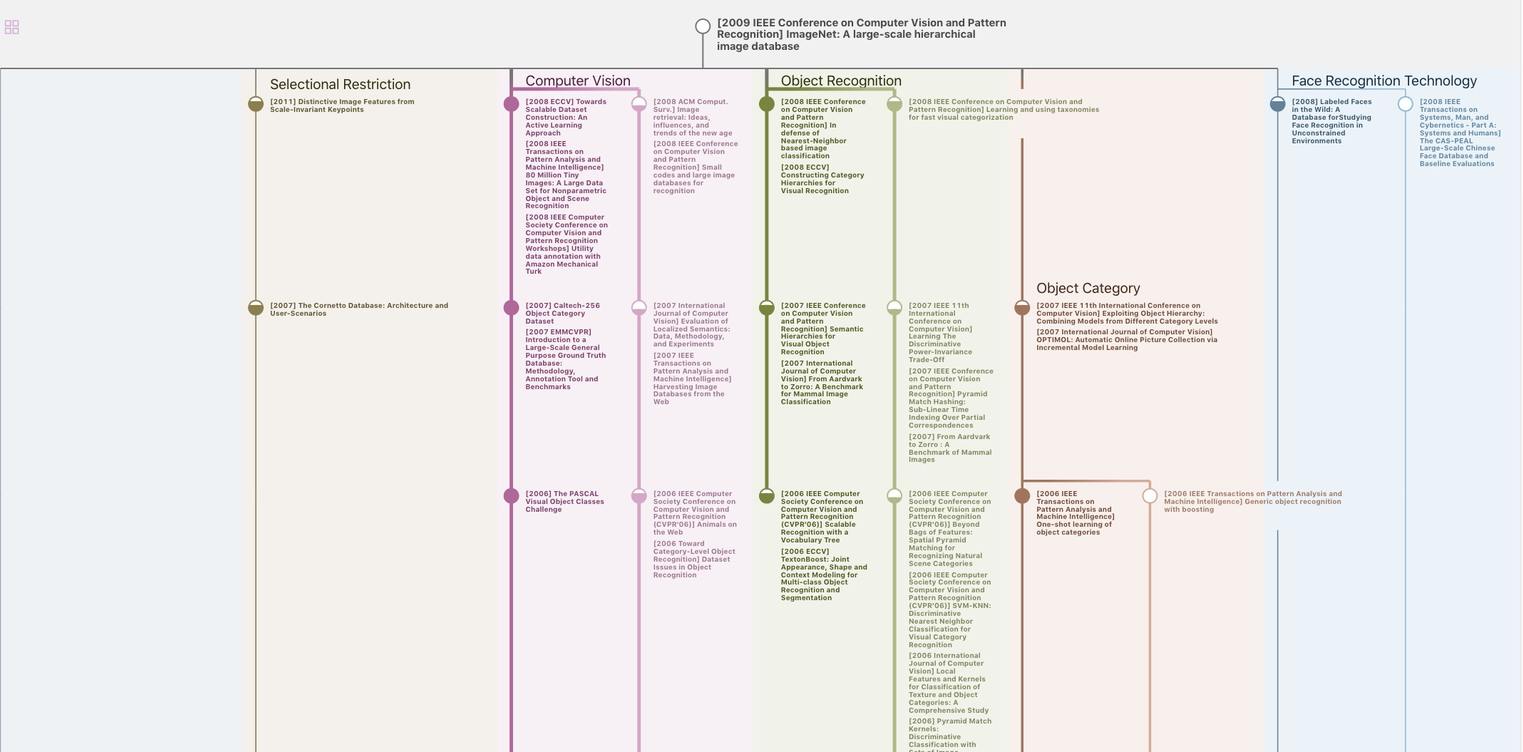
生成溯源树,研究论文发展脉络
Chat Paper
正在生成论文摘要