Learning Control Policy with Previous Experiences from Robot Simulator
2020 International Conference on Information and Communication Technology Convergence (ICTC)(2020)
摘要
Advances in deep reinforcement learning enabled cost-efficient training of control policy of physical robot actions from robot simulators. Learning control policy in a simulated environment is cost-efficient over learning in a real environment. Reward engineering is one of the key components to train efficient control policy. For tasks with long horizons such as navigation and manipulation, a sparse reward is providing limited information. The robot simulator for a physical engine of physical robot manipulation has made it easy for researchers in the field of deep reinforcement learning to simulate complicated robot manipulation environments. In this paper, A robot manipulation simulator and a deep RL framework are utilized for implement a training control policy by utilizing previous experiences. For implementation, Recent innovation Hindsight Experience Replay (HER) algorithms with previous experiences to calculate dense rewards from a sparse reward is leveraged . Proposed implementation showed an approach to investigate the reward engineering method to formulate dense reward in robot manipulator tasks.
更多查看译文
关键词
Deep Reinforcement Learning,Robot Learning,Robot Manipulation,Reward Engineering,Dense Reward
AI 理解论文
溯源树
样例
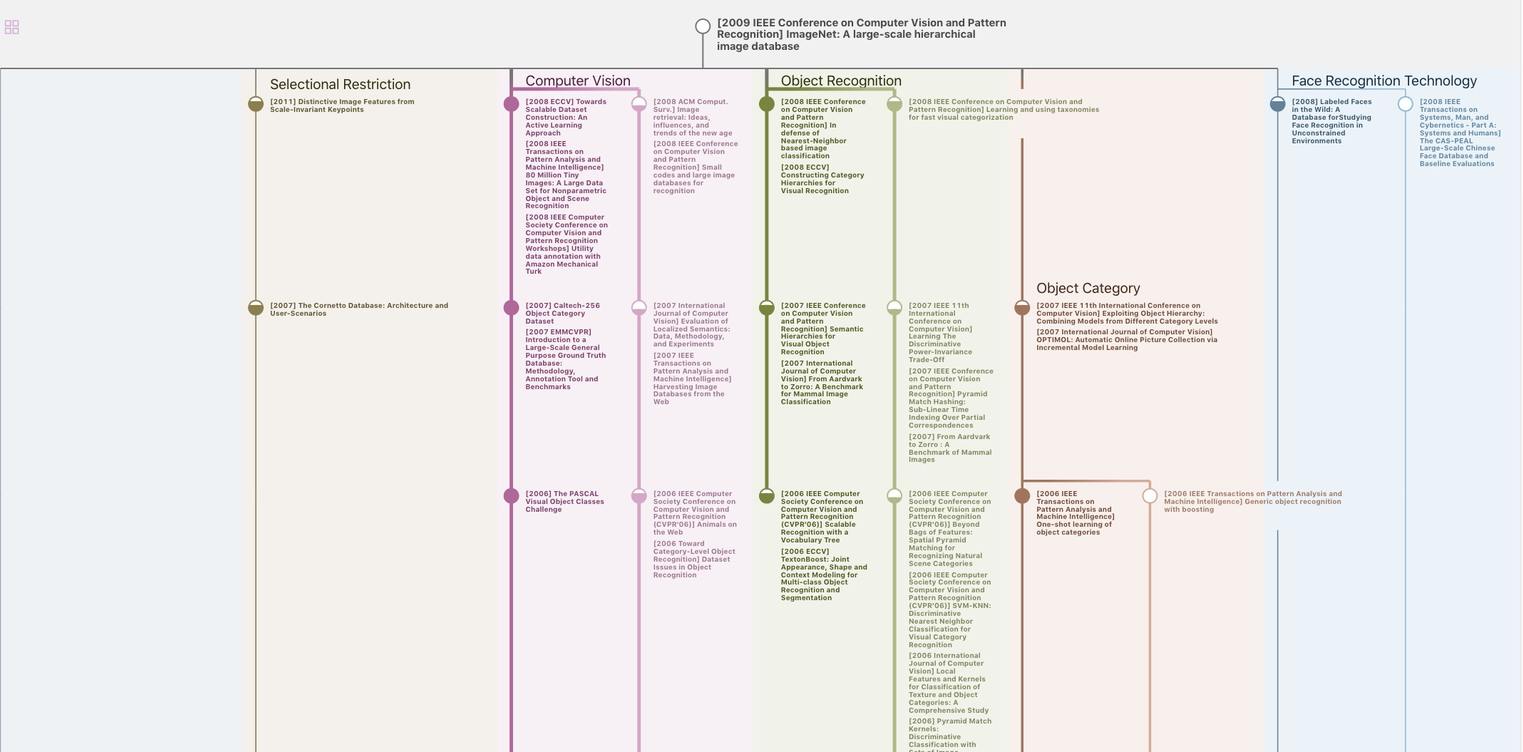
生成溯源树,研究论文发展脉络
Chat Paper
正在生成论文摘要