Graph Theory for Dimensionality Reduction: A Case Study to Prognosticate Parkinson's
2020 11th IEEE Annual Information Technology, Electronics and Mobile Communication Conference (IEMCON)(2020)
摘要
In the present world, the commotion centering Big Data is somewhat obscuring the craft of mining information from smaller samples. Populations with limited examples but huge dimensionality are a common phenomenon, otherwise known as the curse of dimensionality-especially in the health sector-thanks to the recently-discovered potential of data mining and the enthusiasm for feature engineering. Correlated, noisy, redundant features are byproducts of this tendency, which makes learning algorithms converge with greater efforts. This paper proposes a novel feature-pruning technique relying on computational graph theory. Restoring the appeal of pre-AI conventional computing, the paper applies Disjoint Set Union (DSU) on unidirectional graphs prepared basis thresholded Spearman's rank correlation coefficient, r. Gradual withdrawal of leniency on Spearman's r caused a greater tendency in features to form clusters, causing the dimensionality to shrink. The results-extracting out finer, more representative roots as features-have been k-fold cross-validated on a case study examining subjects for Parkinson's. Qualitatively, the method overcomes Principal Component Analysis's (PCA) limitation of inexplicit merging of features and Linear Discriminant Analysis's (LDA) limitation of inextendibility to multiple classes. Statistical inference verified a significant rise in performance, establishing an example of conventional hard computing reinforcing modern soft computing.
更多查看译文
关键词
disjoint set union,dimensionality reduction,Spearman's,Pearson's,Parkinson's disease,statistical inference
AI 理解论文
溯源树
样例
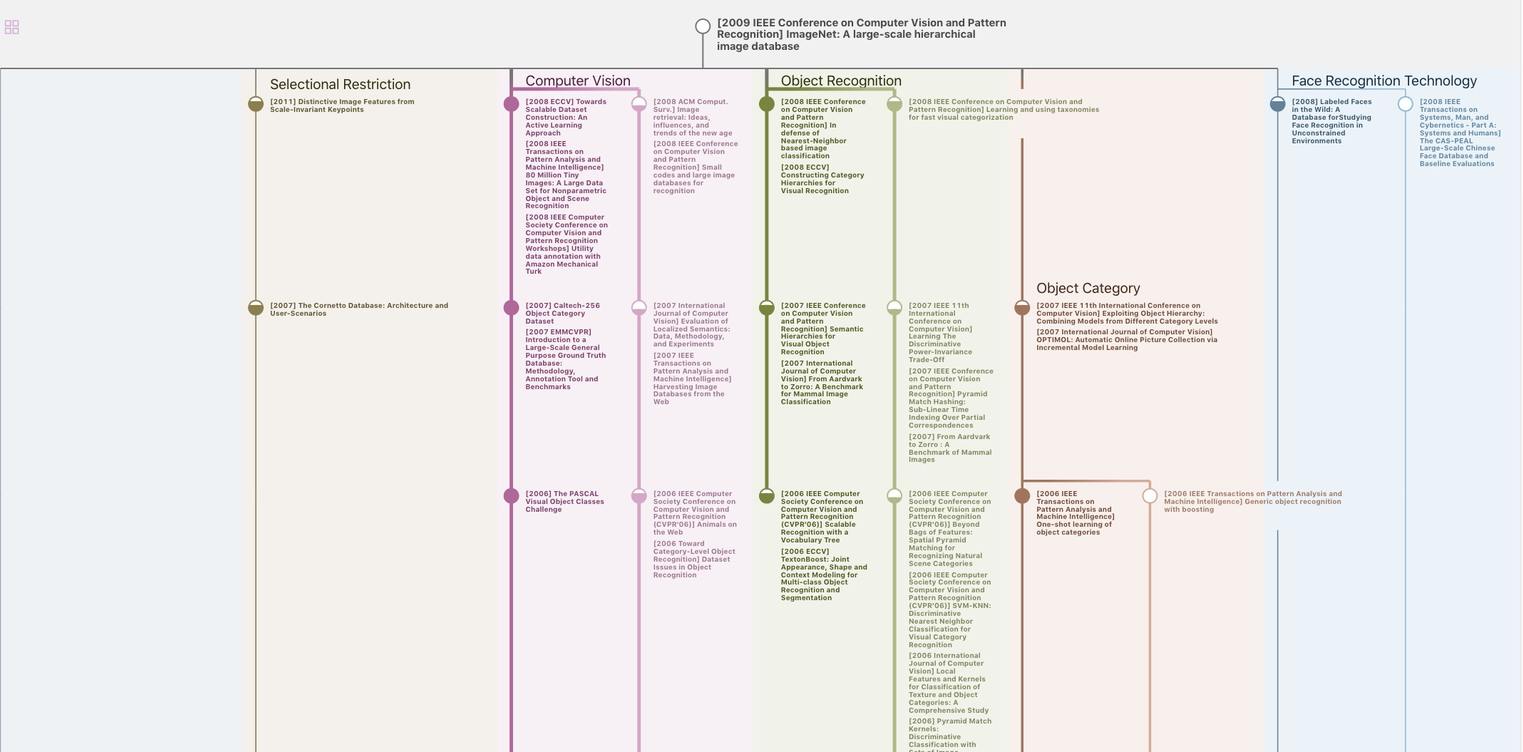
生成溯源树,研究论文发展脉络
Chat Paper
正在生成论文摘要