Massive Lms Log Data Analysis For The Early Prediction Of Course-Agnostic Student Performance
COMPUTERS & EDUCATION(2021)
摘要
The early prediction of students' performance is a valuable resource to improve their learning. If we are able to detect at-risk students in the initial stages of the course, we will have more time to improve their performance. Likewise, excellent students could be motivated with customized additional activities. This is why there are research works aimed to early detect students' performance. Some of them try to achieve it with the analysis of LMS log files, which store information about student interaction with the LMS. Many works create predictive models with the log files generated for the whole course, but those models are not useful for early prediction because the actual log information used for predicting is different to the one used to train the models. Other works do create predictive models with the log information retrieved at the early stages of courses, but they are just focused on a particular type of course.In this work, we use machine learning to create models for the early prediction of students' performance in solving LMS assignments, by just analyzing the LMS log files generated up to the moment of prediction. Moreover, our models are course agnostic, because the datasets are created with all the University of Oviedo(1) courses for one academic year. We predict students' performance at 10%, 25%, 33% and 50% of the course length. Our objective is not to predict the exact student's mark in LMS assignments, but to detect at-risk, fail and excellent students in the early stages of the course. That is why we create different classification models for each of those three student groups. Decision tree, nave Bayes, logistic regression, multilayer perceptron (MLP) neural network, and support vector machine models are created and evaluated. Accuracies of all the models grow as the moment of prediction increases. Although all the algorithms but nave Bayes show accuracy differences lower than 5%, MLP obtains the best performance: from 80.1% accuracy when 10% of the course has been delivered to 90.1% when half of it has taken place. We also discuss the LMS log entries that most influence the students' performance. By using a clustering algorithm, we detect six different clusters of students regarding their interaction with the LMS. Analyzing the interaction patterns of each cluster, we find that those patterns are repeated in all the early stages of the course. Finally, we show how four out of those six student-LMS interaction patterns have a strong correlation with students' performance.
更多查看译文
关键词
Learning management systems, Early prediction, Interaction patterns, Student performance, Machine learning
AI 理解论文
溯源树
样例
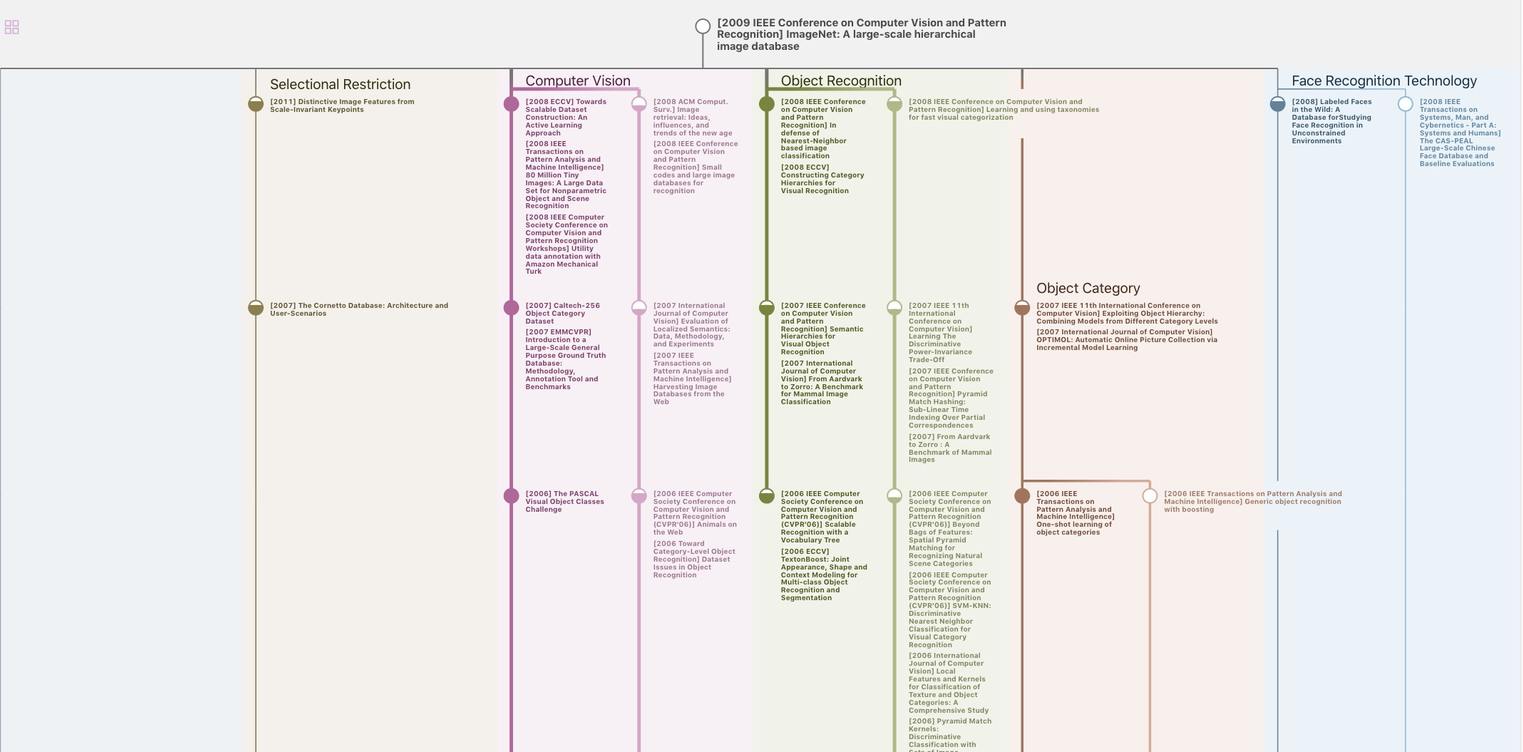
生成溯源树,研究论文发展脉络
Chat Paper
正在生成论文摘要