Deep Learning for Accurate Indoor Human Tracking with a mm-Wave Radar
2020 IEEE Radar Conference (RadarConf20)(2020)
摘要
We address the use of backscattered mm-wave radio signals to track humans as they move within indoor environments. The common approach in the literature leverages the extended Kalman filter (EKF) method, which however undergoes a severe performance degradation when the system evolution model is highly non-linear or presents long-term time dependencies among the system states. In this work, we propose an original model-free tracking procedure based on denoising autoencoders and sequence-to-sequence neural networks, showing its superior performance with respect to state-of-the-art methods. Our architecture can be trained in either a supervised or unsupervised manner, trading tracking accuracy for flexibility. The proposed system is tested on our own measurements, obtained with a 77 GHz radar on single and multiple subjects simultaneously moving in an indoor space. The results are compared against the ground truth trajectories from a motion tracking system, obtaining average tracking errors as low as 12 cm.
更多查看译文
关键词
mm-wave radar,indoor sensing,human tracking,denoising autoencoders,sequence-to-sequence autoencoders
AI 理解论文
溯源树
样例
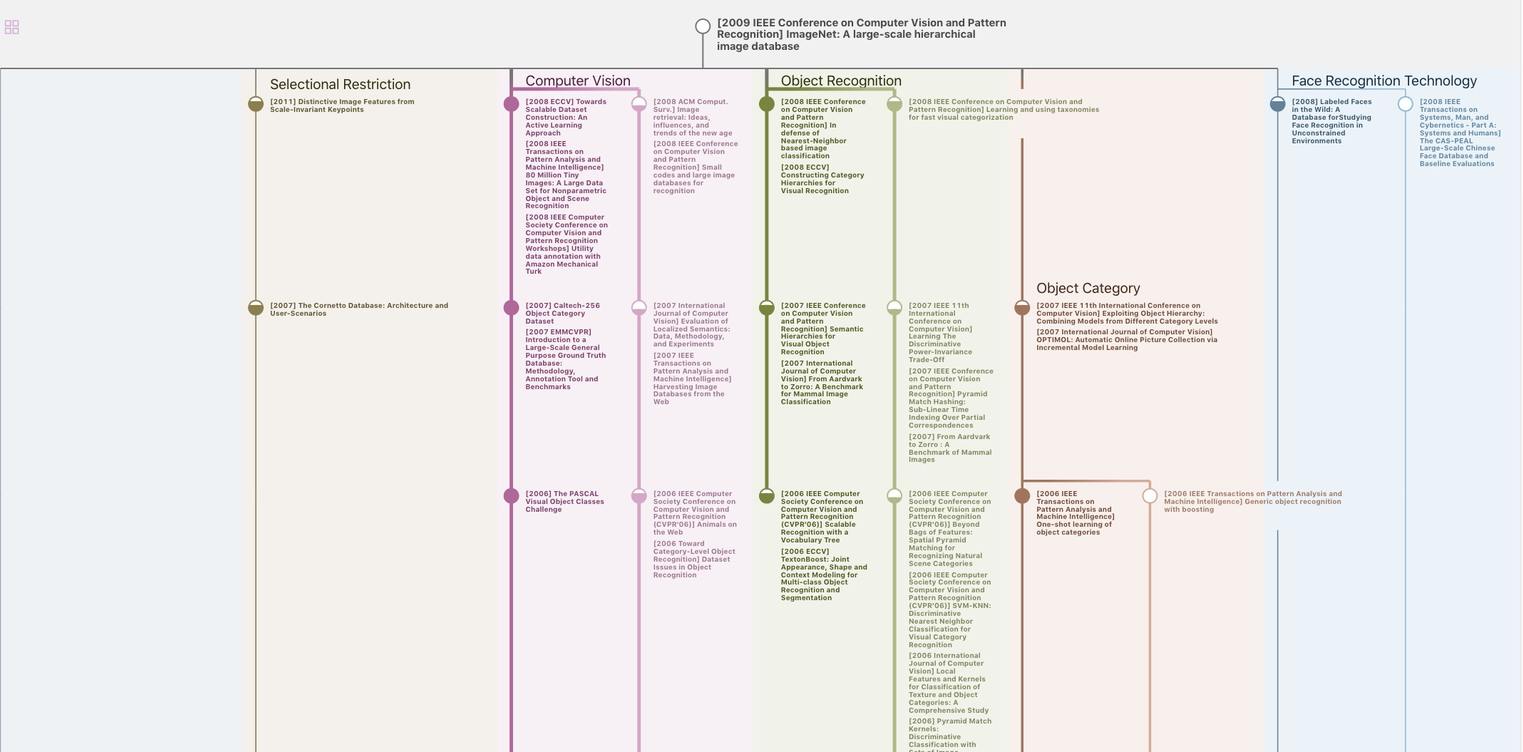
生成溯源树,研究论文发展脉络
Chat Paper
正在生成论文摘要