Rocorl: Transferable Reinforcement Learning-Based Robust Control For Cyber-Physical Systems With Limited Data Updates
IEEE ACCESS(2020)
摘要
Autonomous control systems are increasingly using machine learning technologies to process sensor data, making timely and informed decisions about performing control functions based on the data processing results. Among such machine learning technologies, reinforcement learning (RL) with deep neural networks has been recently recognized as one of the feasible solutions, since it enables learning by interaction with environments of control systems. In this paper, we consider RL-based control models and address the problem of temporally outdated observations often incurred in dynamic cyber-physical environments. The problem can hinder broad adoptions of RL methods for autonomous control systems. Specifically, we present an RL-based robust control model, namely rocorl, that exploits a hierarchical learning structure in which a set of low-level policy variants are trained for stale observations and then their learned knowledge can be transferred to a target environment limited in timely data updates. In doing so, we employ an autoencoder-based observation transfer scheme for systematically training a set of transferable control policies and an aggregated model-based learning scheme for data-efficiently training a high-level orchestrator in a hierarchy. Our experiments show that rocorl is robust against various conditions of distributed sensor data updates, compared with several other models including a state-of-the-art POMDP method.
更多查看译文
关键词
Real-time systems, Data models, Robot sensing systems, Reinforcement learning, Training, Sensors, Cyber-physical systems, Cyber-physical system, real-time data, reinforcement learning, model-based learning, stale observations
AI 理解论文
溯源树
样例
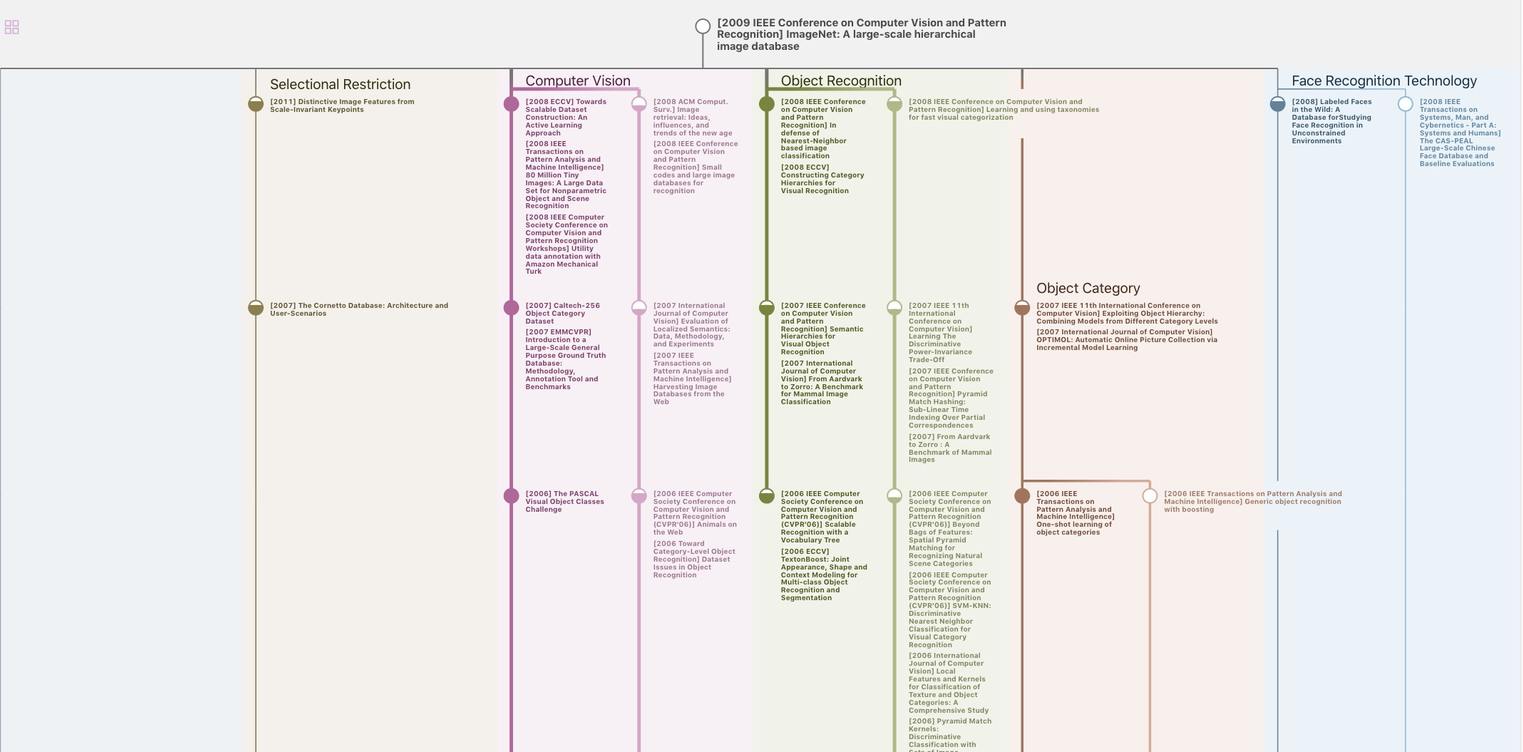
生成溯源树,研究论文发展脉络
Chat Paper
正在生成论文摘要