Hybrid Method Based on Random Convolution Nodes for Short-Term Wind Speed Forecasting
IEEE Transactions on Industrial Informatics(2022)
摘要
Despite having a plethora of works, wind speed time-series forecasting capabilities are prone to errors due to their intermittent and nonstationary nature as well as the limited generalization capabilities of forecasting methods for non-Gaussian distributed data. In this article, a hybrid method that consists of elastic variational mode decomposition (eVMD) and
forecasting
random convolution nodes (fRCN) is proposed to forecast the Gaussian heteroscedastic wind speed time-series. The proposed eVMD algorithm gauges the nonstationary characteristics (complexity) of the wind speed signal and thereafter decomposes the signal into its intrinsic components (ICs) accordingly. The fRCN method rely on local receptive fields to extract features that contribute to the local variations and the global trend in each IC. These features are subsequently learned using extreme learning machines theories. An ensemble unit is employed to learn appropriate weightages for each forecasted IC before yielding the final forecasting values. Suitability of the proposed hybrid method for wind speed forecasting is evaluated via an actual wind speed dataset and comparing against various existing hybrid methods.
更多查看译文
关键词
Local receptive fields,random convolution nodes,variational mode decomposition,wind speed forecasting
AI 理解论文
溯源树
样例
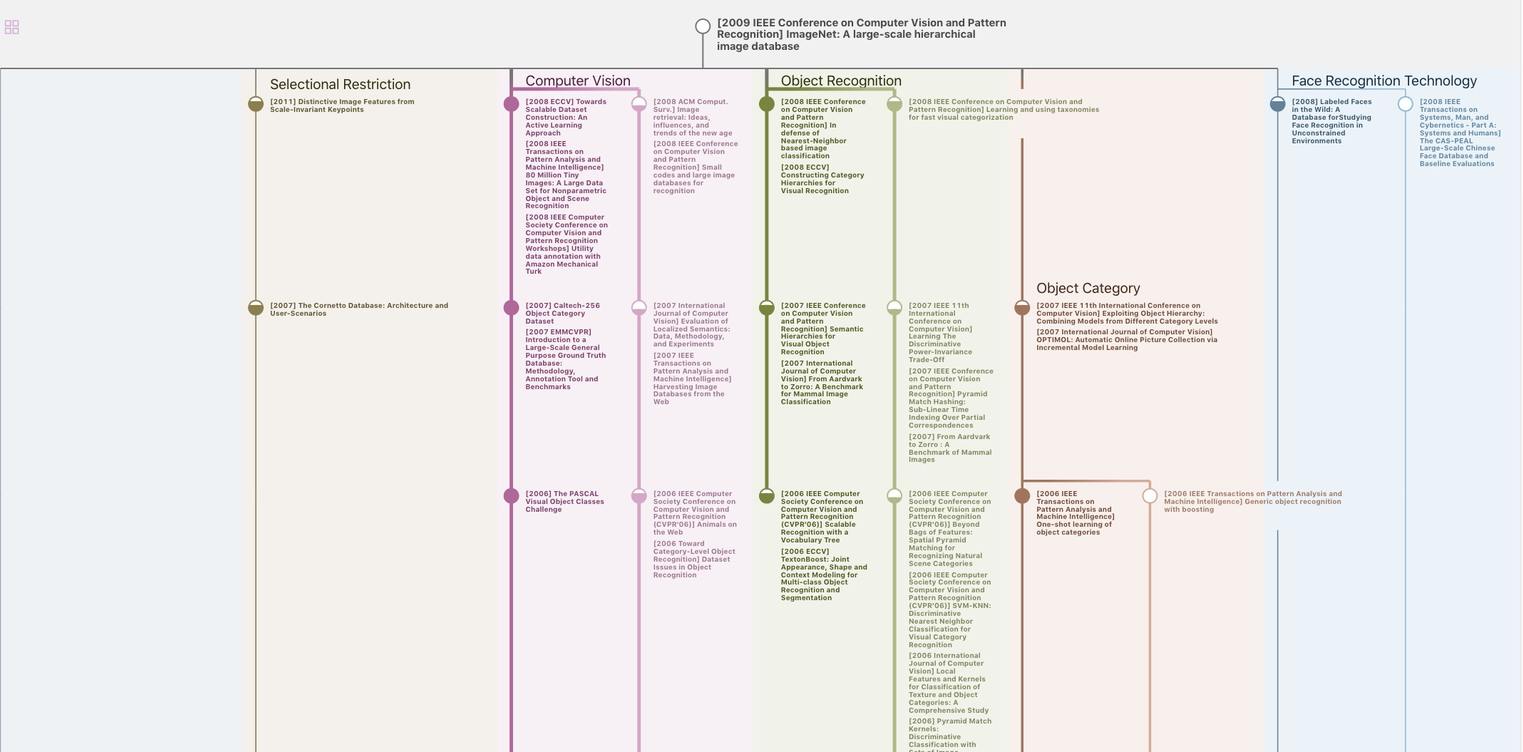
生成溯源树,研究论文发展脉络
Chat Paper
正在生成论文摘要