Intelligent Detection And Recovery Of Missing Electric Load Data Based On Cascaded Convolutional Autoencoders
SCIENTIFIC PROGRAMMING(2020)
摘要
Under the background of Energy Internet, the ever-growing scale of the electric power system has brought new challenges and opportunities. Numerous categories of measurement data, as the cornerstone of communication, play a crucial role in the security and stability of the system. However, the present sampling and transmission equipment inevitably suffers from data missing, which seriously degrades the stable operation and state estimation. Therefore, in this paper, we consider the load data as an example and first develop a missing detection algorithm in terms of the absolute difference sequence (ADS) and linear correlation to detect any potential missing data. Then, based on the detected results, we put forward a missing recovery model named cascaded convolutional autoencoders (CCAE), to recover those missing data. Innovatively, a special preprocessing method has been adopted to reshape the one-dimensional load data as a two-dimensional matrix, and hence, the image inpainting technologies can be conducted to address the problem. Also, CCAE is designed to reconstruct the missing data grade by grade due to its priority strategy, which enhances the robustness upon extreme missing situations. The numerical results on the load data of the Belgium grid validate the promising performance and effectiveness of the proposed solutions.
更多查看译文
AI 理解论文
溯源树
样例
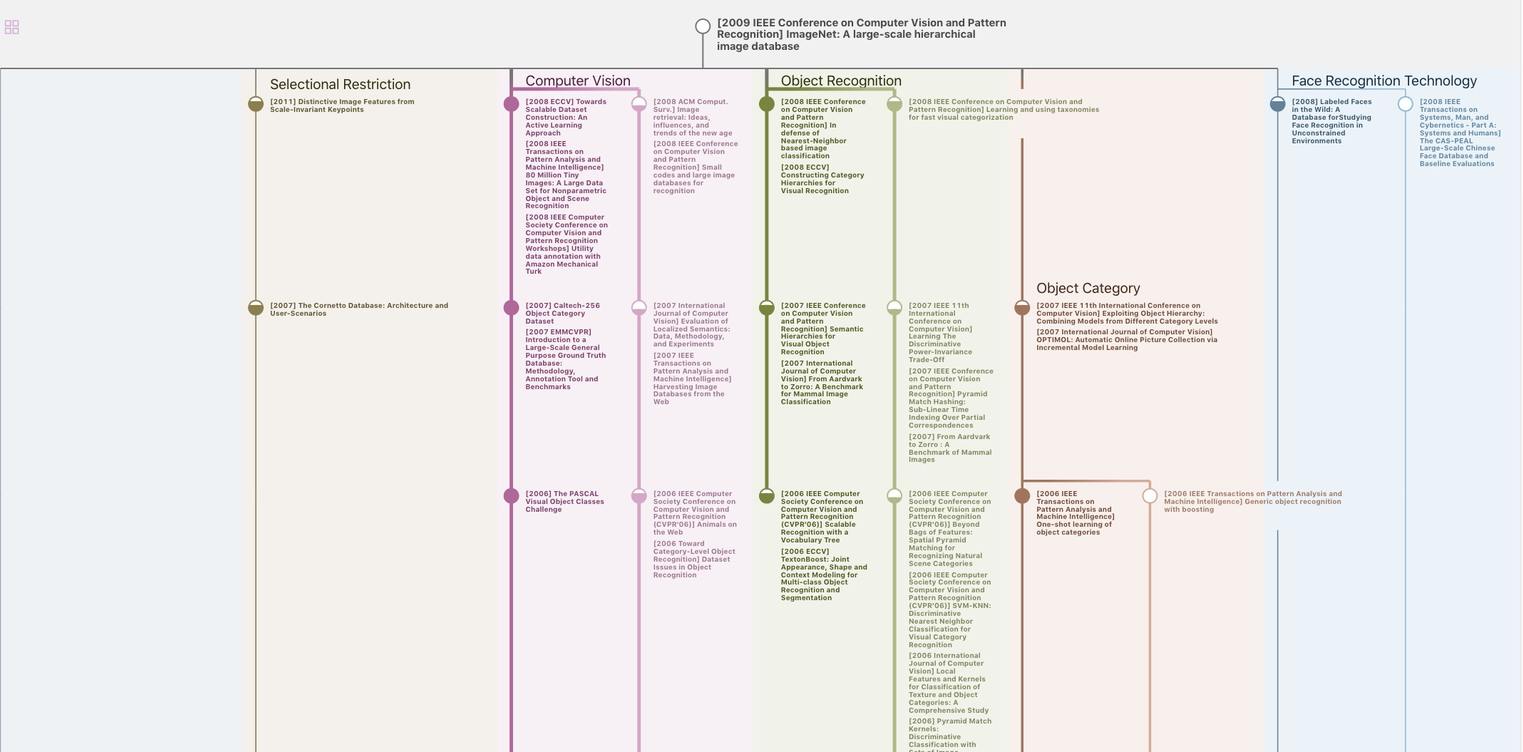
生成溯源树,研究论文发展脉络
Chat Paper
正在生成论文摘要