Generating Novel Compounds Targeting SARS-CoV-2 Main Protease Based on Imbalanced Dataset
2020 IEEE International Conference on Bioinformatics and Biomedicine (BIBM)(2020)
摘要
The de novo drug design plays an important role in the drug discovery. Recently deep learning based method has been popular as a promising approach for the design of novel drugs with desirable properties. However, conventional target-specific generative models mainly concentrate on the known inhibitors and thus produce similar molecules. And these derivatives of known inhibitors are probably negative against the same target. Considering the cost of chemical synthesis and experimental validation, the low false positive rate of generative molecules is very important. In this paper, we propose an efficient pipeline to generate novel SARS-CoV-2 3C-like protease inhibitors. Based on the GPT2 generator and the well performing multi-task predictor which achieves high precision on the highly imbalanced 3CL in vitro screening dataset (650 positive of 297,467 molecules), we acquired a number of novel 3CL-target compounds and analyzed their molecular properties. Moreover, we applied randomized SMILES for data augmentation of positive molecules to create larger chemical space for the generator. Finally, the selected positive compounds with desirable properties are exhibited, as well as their nearest neighbors of 3CL inhibitors which have already been verified in vitro.
更多查看译文
关键词
de novo drug design,SARS-CoV-2,3C-like protease,imbalanced dataset,transformer
AI 理解论文
溯源树
样例
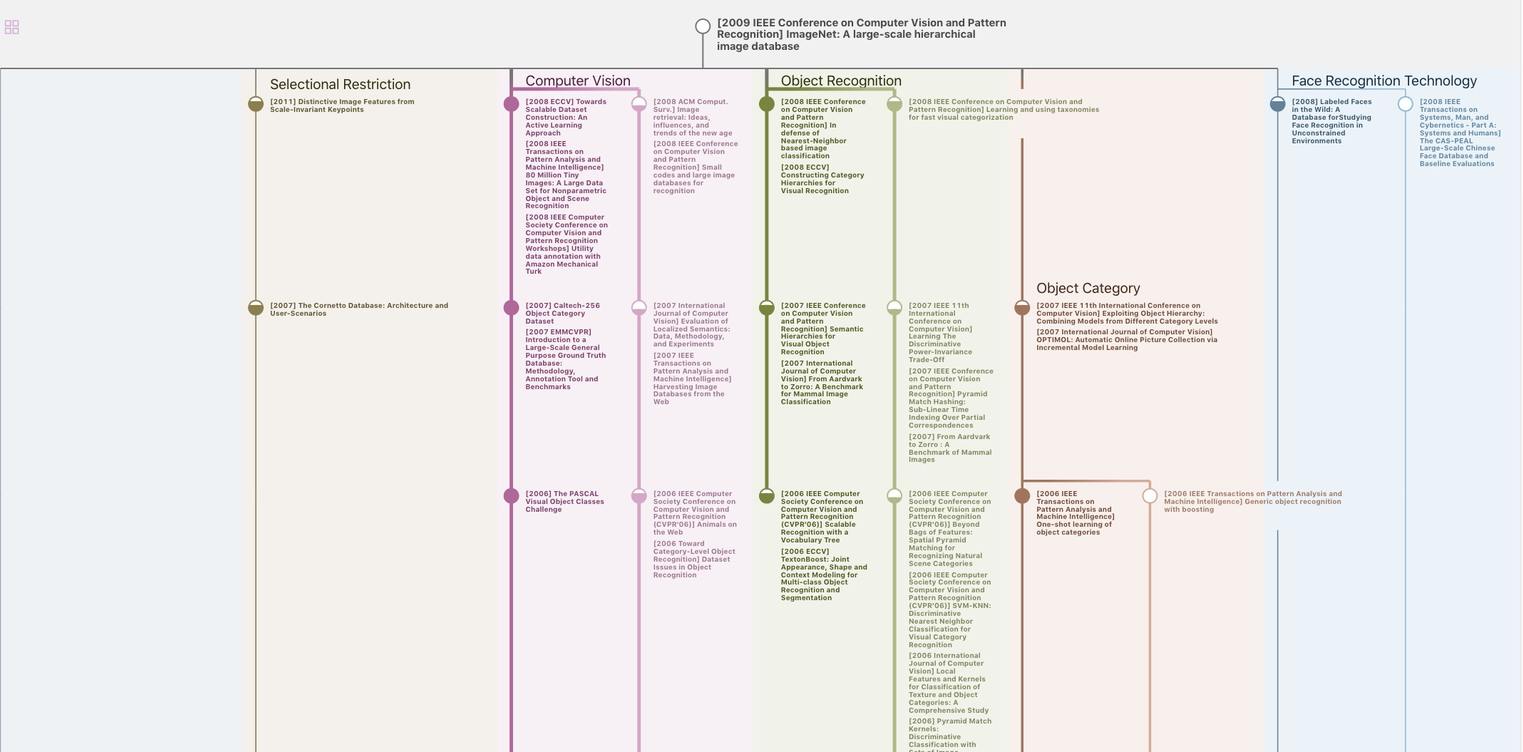
生成溯源树,研究论文发展脉络
Chat Paper
正在生成论文摘要