Automatic Extraction of Skeletal Maturity from Whole Body Pediatric Scoliosis X-rays Using Regional Proposal and Compound Scaling Convolutional Neural Networks.
BIBM(2020)
摘要
Skeletal maturity assessment plays an important role in the management of pediatric orthopedic conditions such as scoliosis, slipped capital femoral epiphysis (SCFE), and pectus. The most common methods to estimate bone age are the use of hand, shoulder, and pelvis x-rays; however, integrating multi-site data adds cost and ionizing radiation exposure. Whole body pediatric scoliosis x-rays, performed for measuring curvature of the spine, include in the field of view multiple development landmarks such as ossifications of the shoulder, pelvis, and proximal femurs in a single exam, potentially providing a comprehensive survey of skeletal maturity that can assist in surgical planning. Therefore, we propose a system to automatically extract multiple skeletal maturity classifications from a single whole body scoliosis x-ray exam. Since these anatomic regions of significance are as small as 2% of the image, we first apply a multi-class region proposal network to extract the humeral head and five pelvic regions based on the modified Oxford Bone Score. We then apply multiple compound scaling convolutional neural networks (EfficientNet) in parallel to clinically stage each region. Our regional detection achieved an F1-score of 0.99, and our staging models achieved an overall accuracy of 89% and intraclass correlation coefficient of 0.84. Our work holds promise for a skeletal maturity assessment system that uses a single image of the entire axial skeleton. This may enable more data points for surgical planning of orthopedic diseases in pediatric patients while minimizing exposure to harmful radiation.
更多查看译文
关键词
scoliosis, convolutional neural networks, machine learning, skeletal maturity, modified Oxford Bone Score
AI 理解论文
溯源树
样例
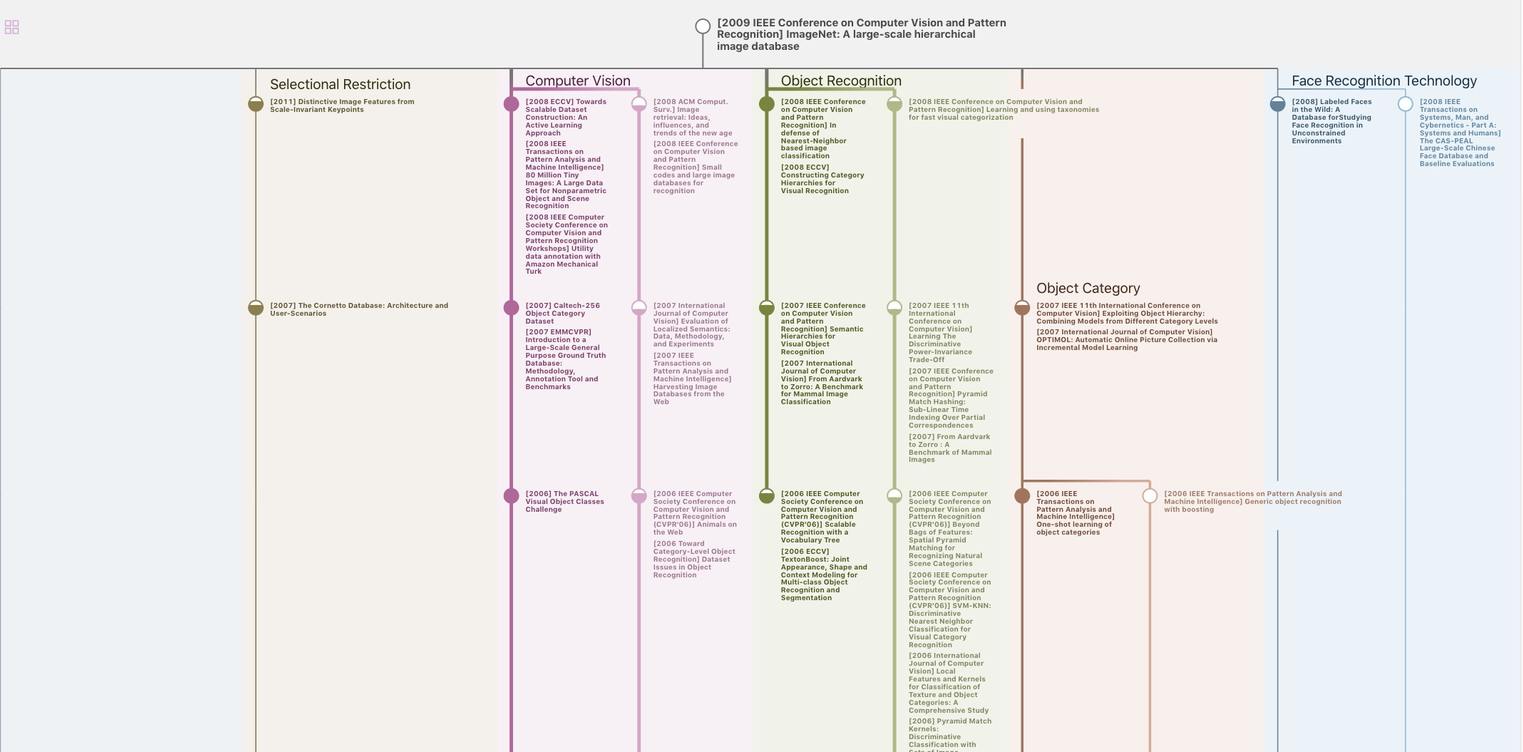
生成溯源树,研究论文发展脉络
Chat Paper
正在生成论文摘要