Neural representation and generation for RNA secondary structures
ICLR(2021)
摘要
Our work is concerned with the generation and targeted design of RNA, a type of genetic macromolecule that can adopt complex structures which influence their cellular activities and functions. The design of large scale and complex biological structures spurs dedicated graph-based deep generative modeling techniques, which represents a key but underappreciated aspect of computational drug discovery. In this work, we investigate the principles behind representing and generating different RNA structural modalities, and propose a flexible framework to jointly embed and generate these molecular structures along with their sequence in a meaningful latent space. Equipped with a deep understanding of RNA molecular structures, our most sophisticated encoding and decoding methods operate on the molecular graph as well as the junction tree hierarchy, integrating strong inductive bias about RNA structural regularity and folding mechanism such that high structural validity, stability and diversity of generated RNAs are achieved. Also, we seek to adequately organize the latent space of RNA molecular embeddings with regard to the interaction with proteins, and targeted optimization is used to navigate in this latent space to search for desired novel RNA molecules.
更多查看译文
关键词
rna,neural representation,secondary structures
AI 理解论文
溯源树
样例
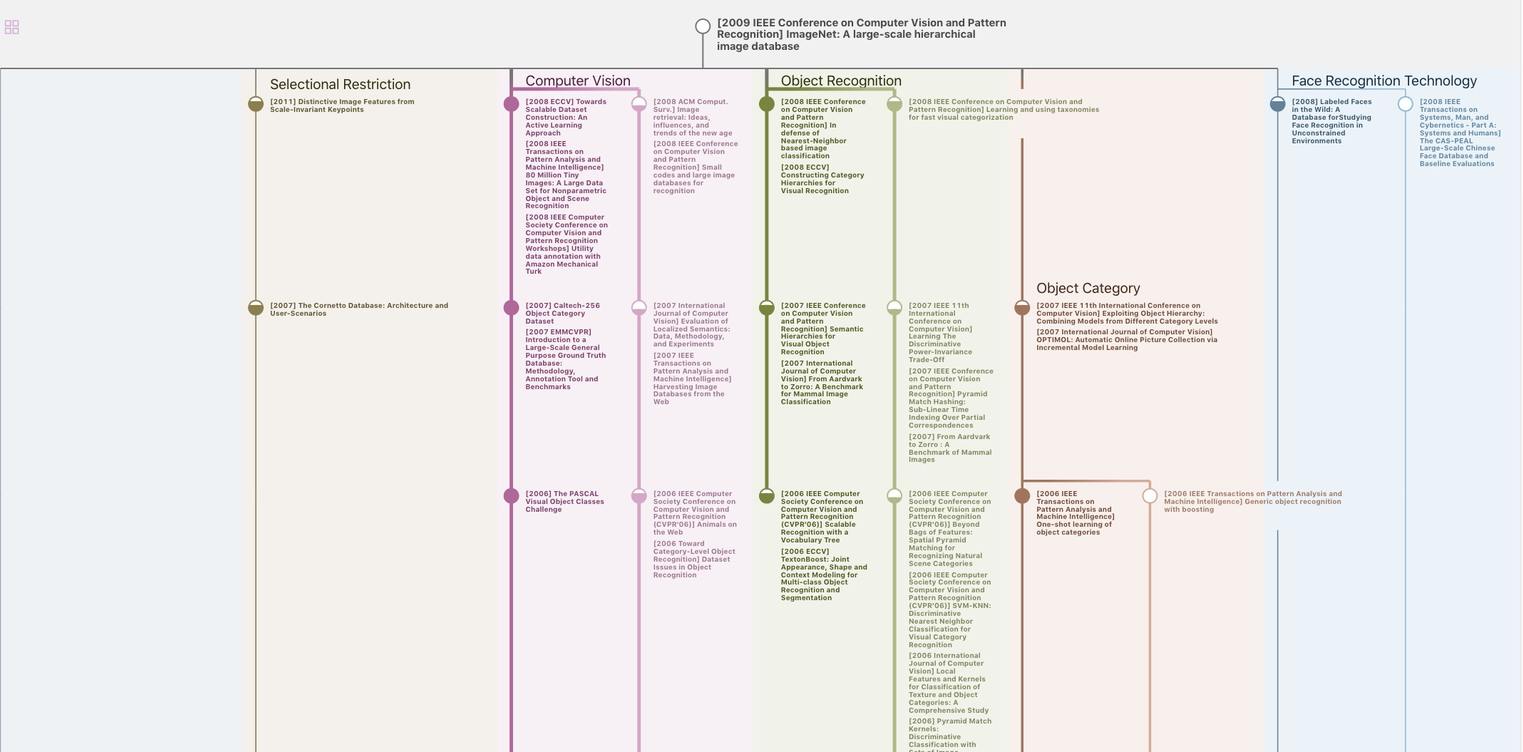
生成溯源树,研究论文发展脉络
Chat Paper
正在生成论文摘要