Double deep multiagent reinforcement learning for autonomous driving in traffic maps with road segments and unsignaled intersections
ITSC(2020)
摘要
In this work we present an advanced deep multiagent reinforcement learning scheme for autonomous driving of multiple vehicles in traffic networks with road segments and unsignaled intersections. The key aspect of the proposed method lies on the definition of the possible routes one vehicle can follow as agents, called route-agents, that allows the transfer learning and the reuse of their learned policies. An informative state space is constructed by introducing a collision matrix that “displays” a predictive traffic map of the intersections that the vehicles traverse. Also, an efficient reward function is designed that aims at providing optimal driving policies in order to navigate vehicles safely and rapidly to their destinations, avoiding collisions. A combined scheme of a Deep Q-Network (DQN) and a feedforward neural network along with the Double Q-leaning technique is employed that offers more stable solutions. Several experiments were made in simulated road traffic maps that empirically illustrate the efficiency of the proposed deep multiagent framework.
更多查看译文
关键词
advanced deep multiagent reinforcement learning scheme,autonomous driving,deep multiagent framework,simulated road traffic maps,Double Q-leaning technique,feedforward neural network,Deep Q-Network,combined scheme,optimal driving policies,efficient reward function,vehicles traverse,predictive traffic map,collision matrix,informative state space,learned policies,transfer learning,called route-agents,possible routes one vehicle,unsignaled intersections,road segments,traffic networks,multiple vehicles
AI 理解论文
溯源树
样例
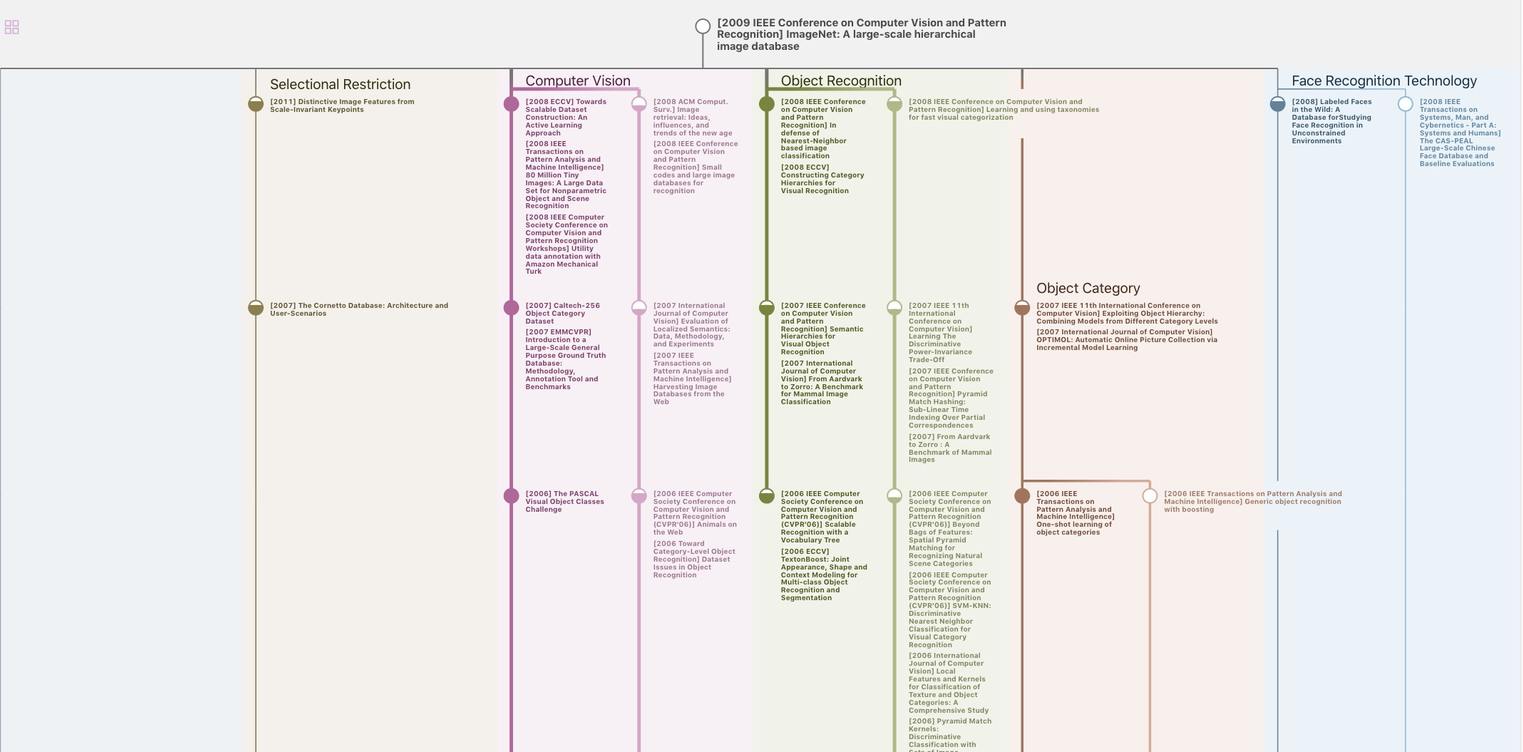
生成溯源树,研究论文发展脉络
Chat Paper
正在生成论文摘要