Learning Properties in LTL ∩ ACTL from Positive Examples Only
2020 Formal Methods in Computer Aided Design (FMCAD)(2020)
摘要
Inferring correct and meaningful specifications of complex (black-box) systems is an important problem in practice, which arises naturally in debugging, reverse engineering, formal verification, and explainable AI, to name just a few examples. Usually, one here assumes that both positive and negative examples of system traces are given-an assumption that is often unrealistic in practice because negative examples (i.e., examples that the system cannot exhibit) are typically hard to obtain. To overcome this serious practical limitation, we develop a novel technique that is able to infer specifications in the form of universal very-weak automata from positive examples only. This type of automata captures exactly the class of properties in the intersection of Linear Temporal Logic (LTL) and the universal fragment of Computation Tree Logic (ACTL), and features an easy-to-interpret graphical representation. Our proposed algorithm reduces the problem of learning a universal very-weak automaton to the enumeration of elements in the Pareto front of a specifically-designed monotonous function and uses classical automaton minimization to obtain a concise, finite-state representation of the learned property. In a case study with specifications from the Advanced Microcontroller Bus Architecture, we demonstrate that our approach is able to infer meaningful, concise, and easy-to-interpret specifications from positive examples only.
更多查看译文
关键词
system traces,learned property,complex black-box system,universal very-weak automata,linear temporal logic,LTL,computation tree logic,ACTL,Pareto front
AI 理解论文
溯源树
样例
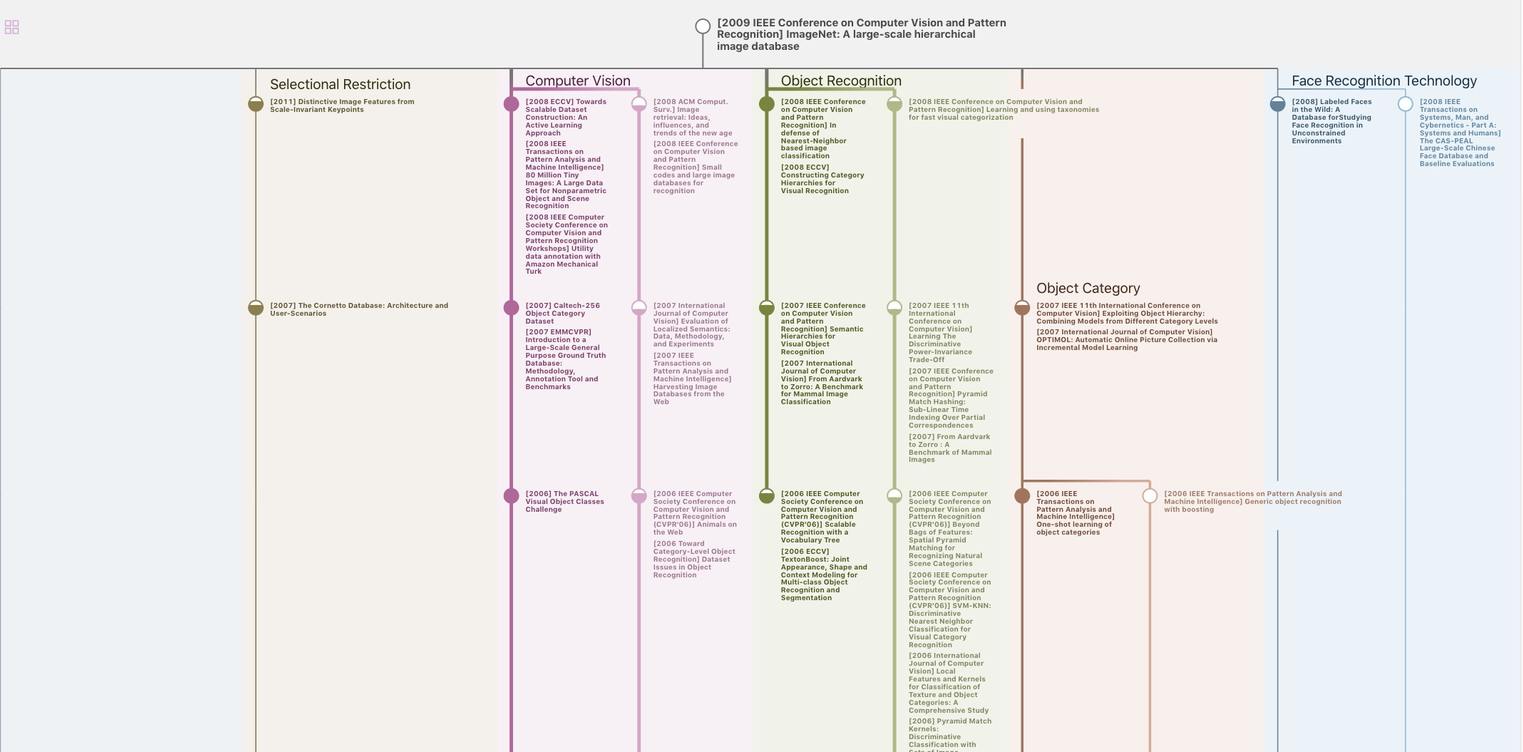
生成溯源树,研究论文发展脉络
Chat Paper
正在生成论文摘要