Adversarial Attack Attribution: Discovering Attributable Signals in Adversarial ML Attacks
arxiv(2021)
摘要
Machine Learning (ML) models are known to be vulnerable to adversarial inputs and researchers have demonstrated that even production systems, such as self-driving cars and ML-as-a-service offerings, are susceptible. These systems represent a target for bad actors. Their disruption can cause real physical and economic harm. When attacks on production ML systems occur, the ability to attribute the attack to the responsible threat group is a critical step in formulating a response and holding the attackers accountable. We pose the following question: can adversarially perturbed inputs be attributed to the particular methods used to generate the attack? In other words, is there a way to find a signal in these attacks that exposes the attack algorithm, model architecture, or hyperparameters used in the attack? We introduce the concept of adversarial attack attribution and create a simple supervised learning experimental framework to examine the feasibility of discovering attributable signals in adversarial attacks. We find that it is possible to differentiate attacks generated with different attack algorithms, models, and hyperparameters on both the CIFAR-10 and MNIST datasets.
更多查看译文
关键词
attribution,attributable signals
AI 理解论文
溯源树
样例
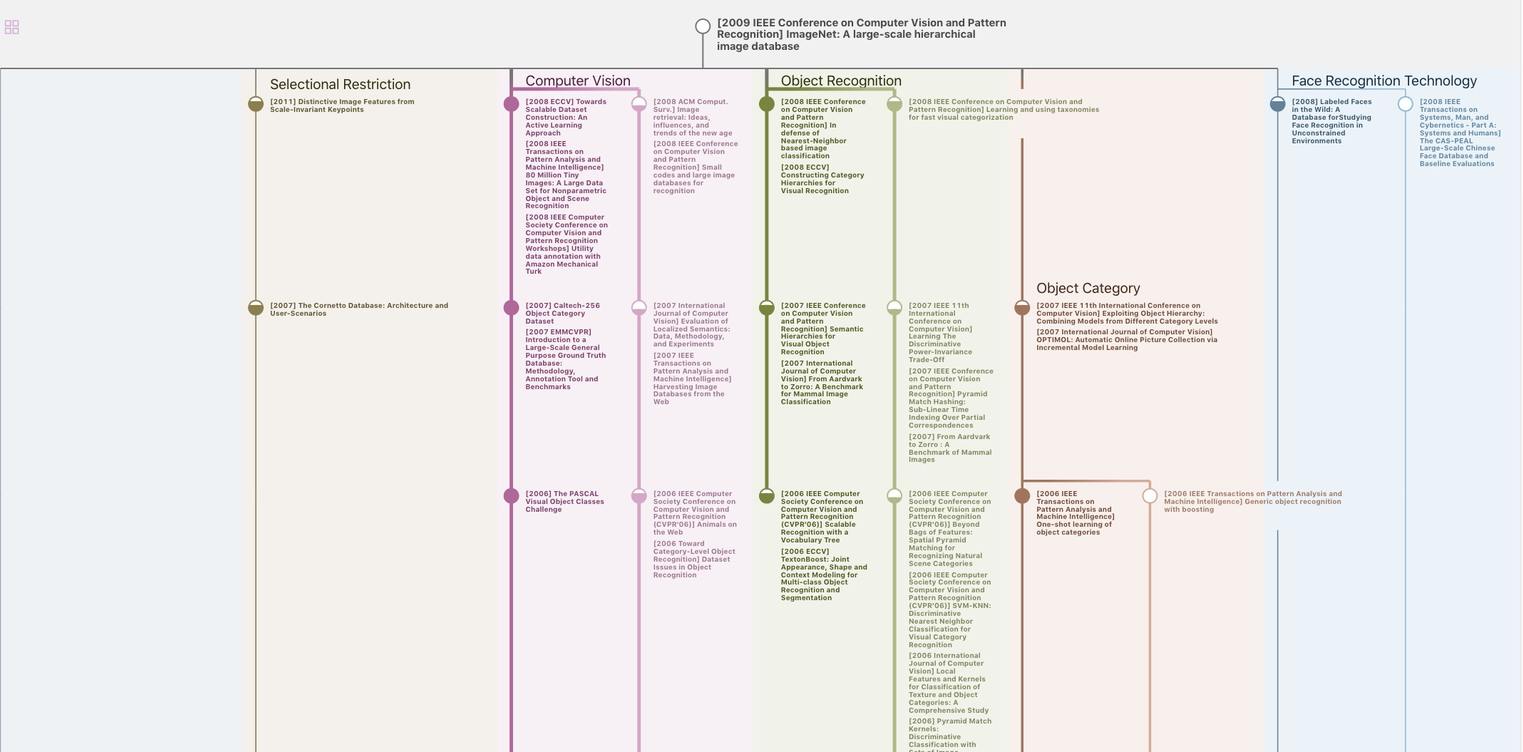
生成溯源树,研究论文发展脉络
Chat Paper
正在生成论文摘要