Data-driven Improvement of Online Conformance Checking
2020 IEEE 24th International Enterprise Distributed Object Computing Conference (EDOC)(2020)
摘要
Conformance checking takes a process model and a process log as input and quantifies the degree of conformance between both. This allows a comparison between the intended behavior represented by the model and the actual behavior captured by the log and is useful for many applications such as auditing. Existing approaches calculate conformance as follows: each deviation between model and log is corrected by an alignment, e.g., inserting a missing event to the log, that has a standard per-deviation cost of 1. While deviations in the model can be handled this way, there is no way to differentiate between intended (e.g., ad-hoc repair of instances) and unintended (e.g., security breaches) deviations. Hence this work proposes an advanced cost function, that allows for per-deviation adjustments of the per-deviation costs. By inspecting how the data elements of subsequent tasks are affected, it becomes possible to automatically increase or decrease the per-deviation costs of 1, thus allowing for an automatic classification of deviation causes. The proposed approach works offline and online (i.e., at runtime) and is evaluated based on a real-world dataset from the manufacturing domain.
更多查看译文
关键词
Process mining and business analytics,Online Conformance Checking,Logging Errors,Data-driven Alignment Costs
AI 理解论文
溯源树
样例
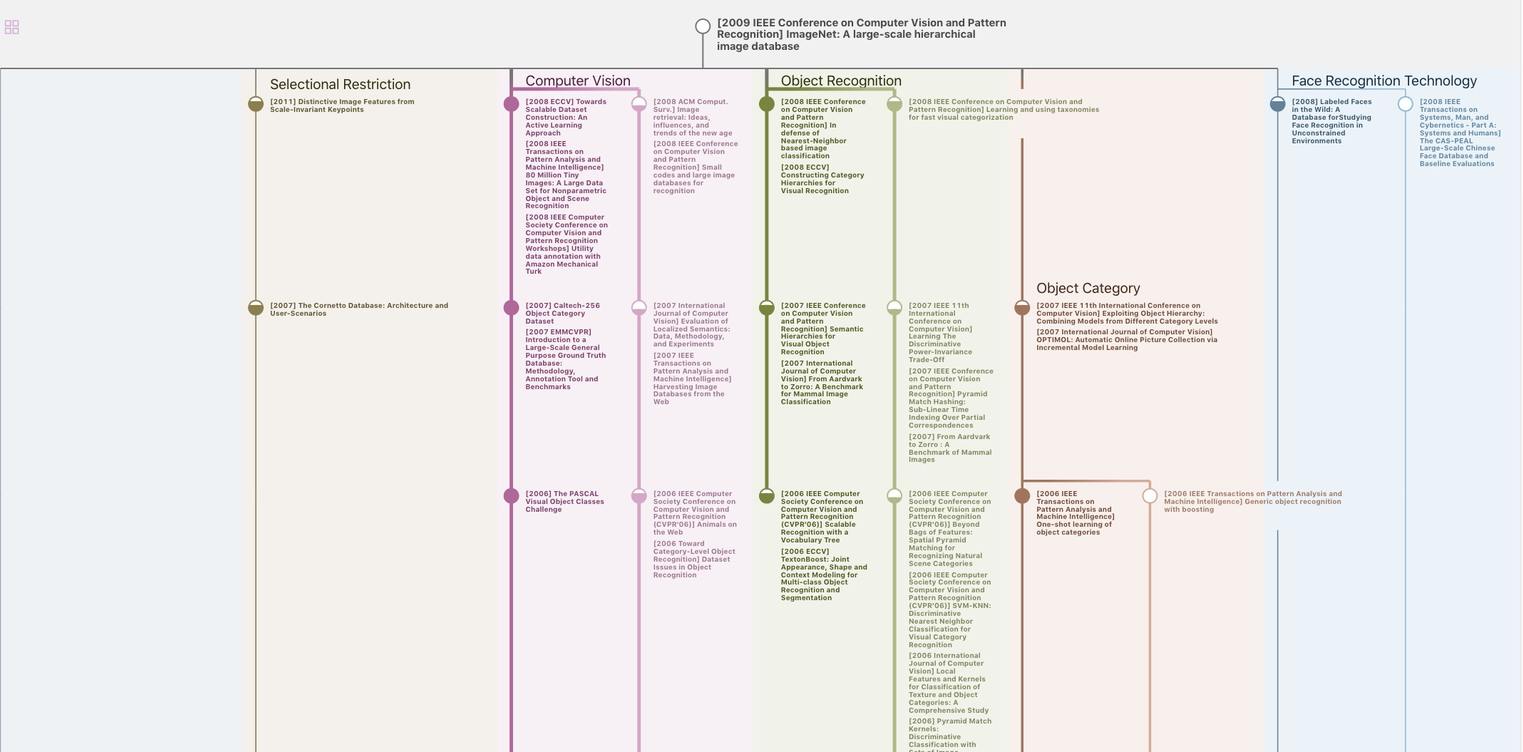
生成溯源树,研究论文发展脉络
Chat Paper
正在生成论文摘要