Multi-Agent Reinforcement Learning for Cooperative Adaptive Cruise Control
2020 IEEE 32nd International Conference on Tools with Artificial Intelligence (ICTAI)(2020)
摘要
A growing trend in the field of autonomous vehicles is the use of platooning. The design of control algorithms for platoons is challenging considering that coordination among vehicles is obtained through diverse communication channels. Currently, Adaptive Cruise Control (ACC) is used in individual vehicles to regulate certain driving functions. ACC can be extended to leverage inter-vehicle communication, creating a tightly coupled vehicle stream in the form of a platoon. This extension, Cooperative Adaptive Cruise Control (CACC), typically assumes full communication among vehicles. In this paper, we develop a deep reinforcement learning based CACC that allows platooning vehicles to learn a robust communication protocol alongside their coordination policies. LSTM is used to implement ACC for each vehicle and is trained using policy gradient. To coordinate driving, the vehicle's LSTM adapts to exchange relevant information with the other vehicles, creating the CACC. We simulate two platoons of 3 and 5 vehicles, respectively. We test our CACC with the learned communication protocol against full and inhibited communication baselines with and without a jamming attack. We also train our approach with local and global reward systems. Results suggest that models with individual rewards and the learned communication protocol achieve higher performance and faster convergence.
更多查看译文
关键词
autonomous vehicles,reinforcement learning,coordination,jamming,LSTM
AI 理解论文
溯源树
样例
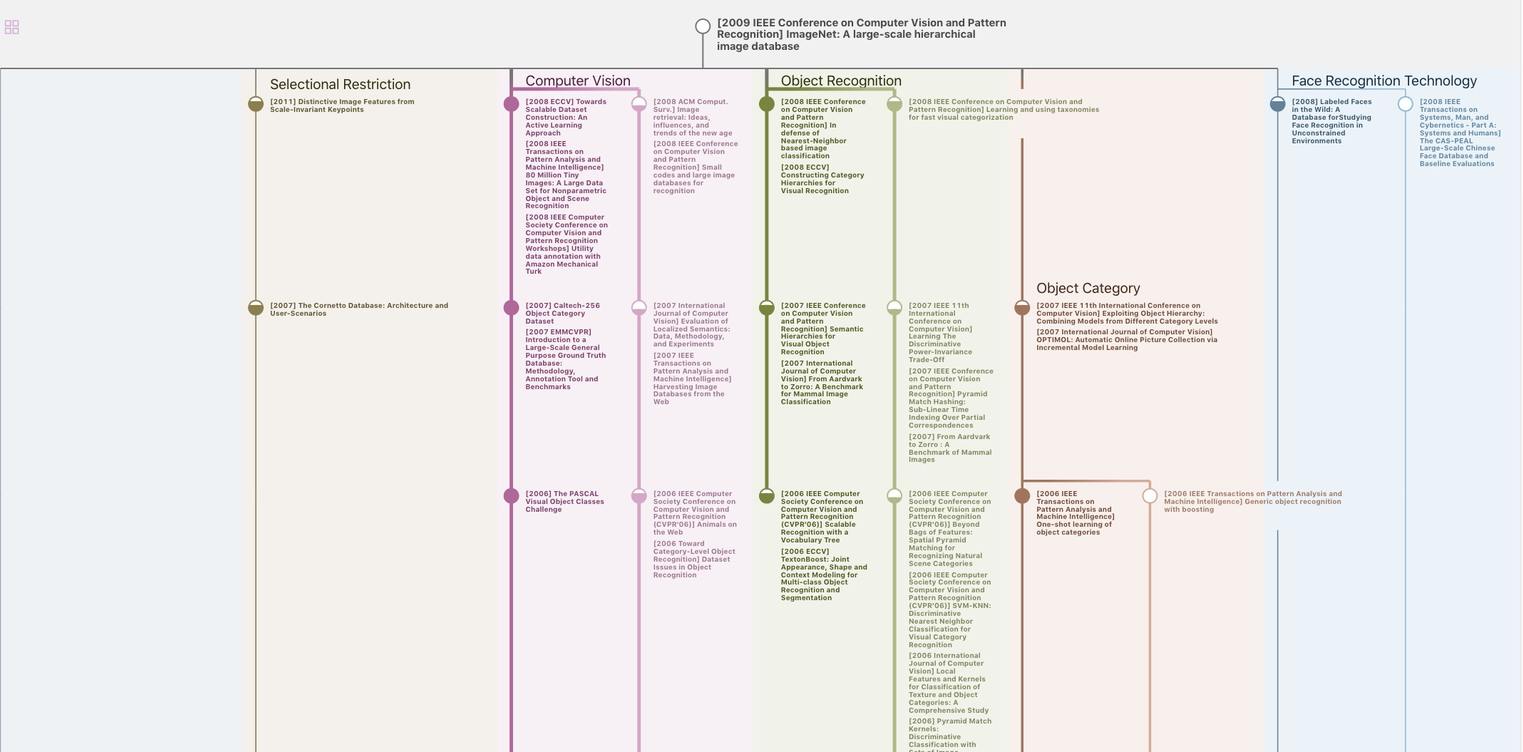
生成溯源树,研究论文发展脉络
Chat Paper
正在生成论文摘要