Improving Mapping for Sparse Direct Solvers - A Trade-Off Between Data Locality and Load Balancing.
Euro-Par(2020)
摘要
In order to express parallelism, parallel sparse direct solvers take advantage of the elimination tree to exhibit tree-shaped task graphs, where nodes represent computational tasks and edges represent data dependencies. One of the pre-processing stages of sparse direct solvers consists of mapping computational resources (processors) to these tasks. The objective is to minimize the factorization time by exhibiting good data locality and load balancing. The proportional mapping technique is a widely used approach to solve this resource-allocation problem. It achieves good data locality by assigning the same processors to large parts of the elimination tree. However, it may limit load balancing in some cases. In this paper, we propose a dynamic mapping algorithm based on proportional mapping. This new approach, named STEAL, relaxes the data locality criterion to improve load balancing. In order to validate the newly introduced method, we perform extensive experiments on the PASTIX sparse direct solver. It demonstrates that our algorithm enables better static scheduling of the numerical factorization while keeping good data locality.
更多查看译文
关键词
sparse direct solvers,data locality,mapping,trade-off
AI 理解论文
溯源树
样例
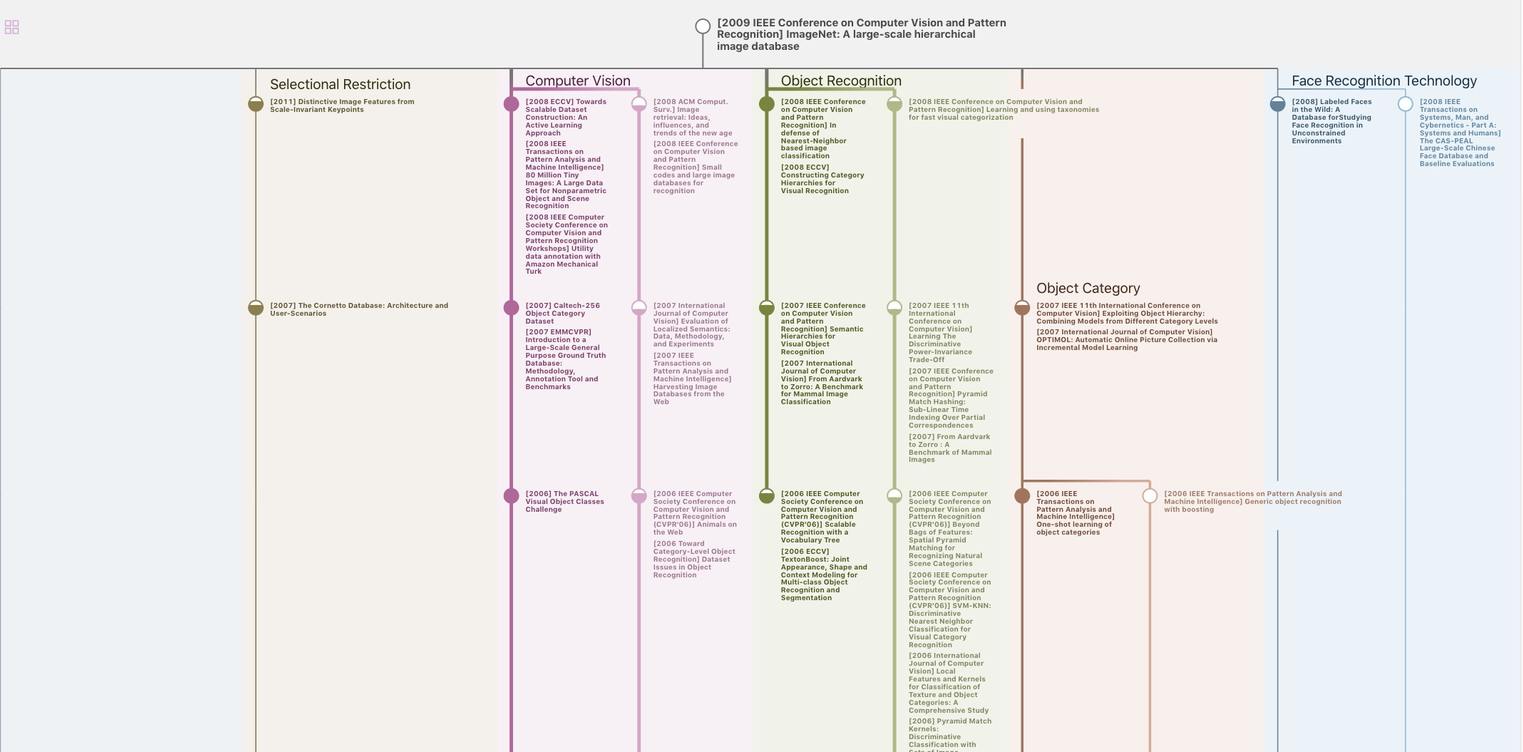
生成溯源树,研究论文发展脉络
Chat Paper
正在生成论文摘要