HisRec - Bridging Heterogeneous Information Spaces for Recommendation via Attentive Embedding.
ADMA(2020)
Abstract
A large volume of knowledge has been accumulated with the prevalence of social networks. Although extra knowledge can mitigate the problem of data sparsity, it is still a challenging task to integrate data across different information spaces for recommendation. In this paper, we propose to address recommendation that involves heterogeneous information spaces, namely interactive space (i.e. user-item), structural space (user-user) and semantic space (user-attribute). Instead of modeling each information space independently, we propose to seamlessly integrate information across heterogeneous spaces. To do this, we propose an attention mechanism in which the users/items attend differently to their structural neighbors (structural space) for learning compact representations. The attentions are parameterized by the interactions between user/item attributes (semantic space), and they are collaboratively learned for the recommendation task (interactive space). In this way, information across different spaces can be complementary to each other for boosting recommendation performance. We also prove that the proposed attentive embedding method is a generalization of traditional social regularization and network embedding methods. We validate the effectiveness of the proposed model with two real world datasets, and show that the proposed model is able to outperform state-of-the-art recommender models significantly.
MoreTranslated text
Key words
attentive embedding,heterogeneous information spaces,recommendation
AI Read Science
Must-Reading Tree
Example
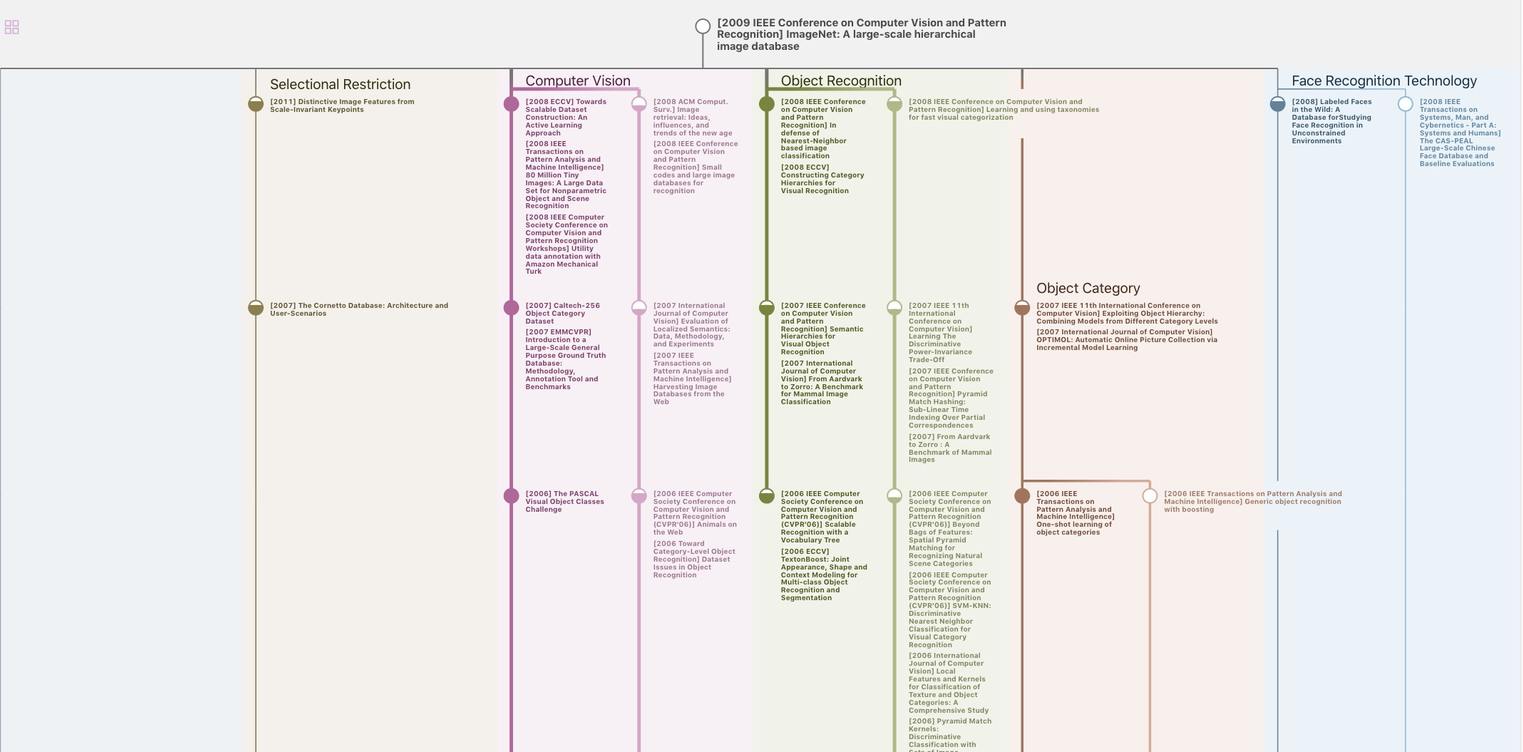
Generate MRT to find the research sequence of this paper
Chat Paper
Summary is being generated by the instructions you defined