DLHub: Simplifying publication, discovery, and use of machine learning models in science
Journal of Parallel and Distributed Computing(2021)
摘要
Machine Learning (ML) has become a critical tool enabling new methods of analysis and driving deeper understanding of phenomena across scientific disciplines. There is a growing need for “learning systems” to support various phases in the ML lifecycle. While others have focused on supporting model development, training, and inference, few have focused on the unique challenges inherent in science, such as the need to publish and share models and to serve them on a range of available computing resources. In this paper, we present the Data and Learning Hub for science (DLHub), a learning system designed to support these use cases. Specifically, DLHub enables publication of models, with descriptive metadata, persistent identifiers, and flexible access control. It packages arbitrary models into portable servable containers, and enables low-latency, distributed serving of these models on heterogeneous compute resources. We show that DLHub supports low-latency model inference comparable to other model serving systems including TensorFlow Serving, SageMaker, and Clipper, and improved performance, by up to 95%, with batching and memoization enabled. We also show that DLHub can scale to concurrently serve models on 500 containers. Finally, we describe five case studies that highlight the use of DLHub for scientific applications.
更多查看译文
关键词
Learning systems,Model serving,Machine learning,DLHub
AI 理解论文
溯源树
样例
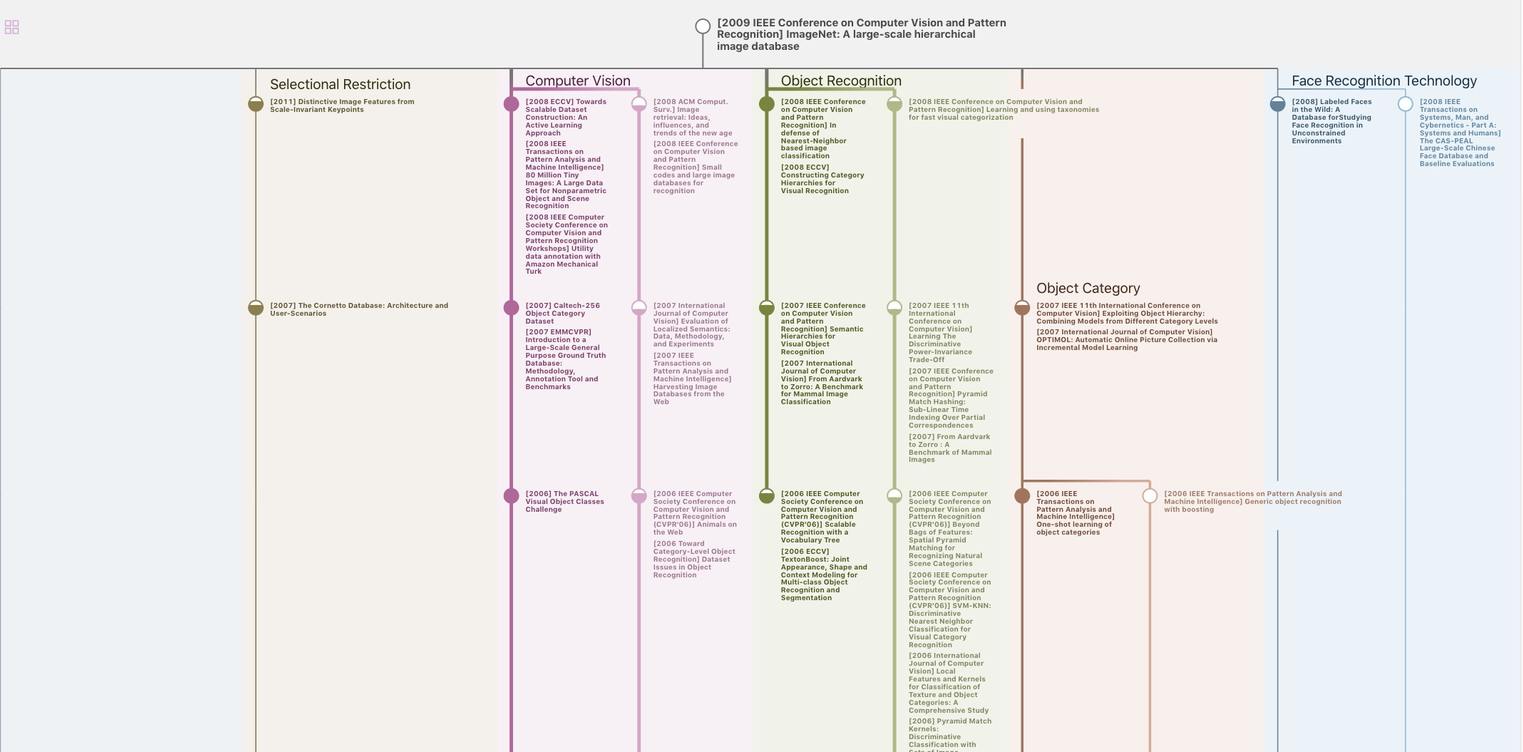
生成溯源树,研究论文发展脉络
Chat Paper
正在生成论文摘要