Context prior-based with residual learning for face detection: A deep convolutional encoder-decoder network.
Signal Processing: Image Communication(2020)
摘要
In the field of security, faces are usually blurry, occluded, diverse pose and small in the image captured by an outdoor surveillance camera, which is affected by the external environment such as the camera pose and range, weather conditions, etc. It can be described as a problem of hard face detection in natural images. To solve this problem, we propose a deep convolutional neural network named feature hierarchy encoder–decoder network (FHEDN). It is motivated by two observations from contextual semantic information and the mechanism of multi-scale face detection. The proposed network is a scale-variant style architecture and single stage, which are composed of encoder and decoder subnetworks. Based on the assumption that contextual semantic information around face being auxiliary to detect faces, we introduce a residual mechanism to fuse context prior-based information into face feature and formulate the learning chain to train each encoder–decoder pair. In addition, we discuss some important factors in implement details such as the distribution of training dataset, the scale of feature hierarchy, and anchor box size, etc. They have some impact on the detection performance of the final network. Compared with some state-of-the-art algorithms, our method achieves promising performance on the popular benchmarks including AFW, PASCAL FACE, FDDB, and WIDER FACE. Consequently, the proposed approach can be efficiently implemented and routinely applied to detect faces with severe occlusion and arbitrary pose variations in unconstrained scenes. Our code and results are available on https://github.com/zzxcoder/EvaluationFHEDN.
更多查看译文
关键词
Context prior,Residual learning,Deep convolutional neural network,Encoder–decoder,Face detection
AI 理解论文
溯源树
样例
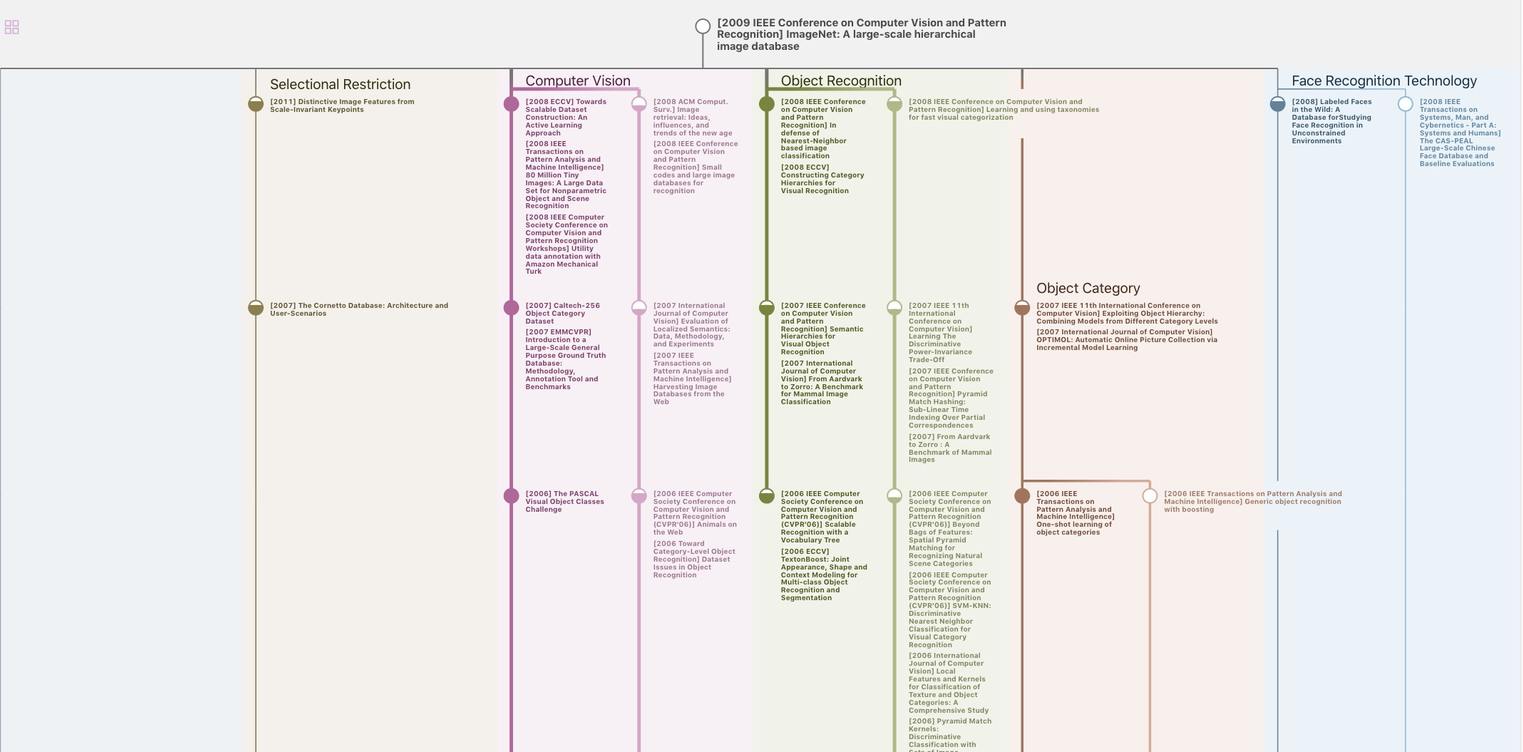
生成溯源树,研究论文发展脉络
Chat Paper
正在生成论文摘要