Faster, Better and More Detailed: 3D Face Reconstruction with Graph Convolutional Networks.
ACCV (5)(2020)
摘要
For the 2D image encoder in coarse mesh reconstruction network, we manage to adopt both ResNet-50 [1] and MobileNetV2 [2] for our purpose, with almost the same parameter settings and training procedure. We illustrate our system again in Fig. 1 for ease of reference. We denote spiral convolution layer with h hops, t filters and v vertices as SPConv(h,t,v), pooling and unpooling by a factor of p as Unpool(p) and Pool(p) respectively. FC layer is written as FC(d), where d is the output feature dimension. It has be mentioned that, in Fig. 1 as well as the following paragraphs, we take ResNet-50 as the example. Our spiral mesh decoder takes a 256-d feature embedding from image encoder as input, and has the following structure: FC(52*128)→Unpool(4)→SPConv(1,128,208)→Unpool(4)→SPConv(1,64,832) →Unpool(4)→SPConv(2,32,3326)→Unpool(4)→SPConv(2,16,13304) →Unpool(4)→SPConv(2,8,53215)→SPConv(2,3,53215). For simplicity, we describe the image feature sampling, adaptation and summation as one operation, viz. AddF(r,d,v), where r represents the spatial resolution of image features. Our refinement network uses a concatenation of coarse face normals and per vertex RGB values as input, and can be described as: SPConv(2,8,53215)→Pool(4)→SPConv(2,16,13304)→Pool(4) →SPConv(2,32,3326)→AddF(64,32,3326)→Unpool(4)→SPConv(2,16,13304) →AddF(128,16,13304)→Unpool(4)→SPConv(2,8,53215)→SPConv(2,3,53215). ELU [3] activation is used after each spiral convolution and fully connected layer, except for the last layer before getting the coarse mesh or per vertex shape displacement prediction.
更多查看译文
AI 理解论文
溯源树
样例
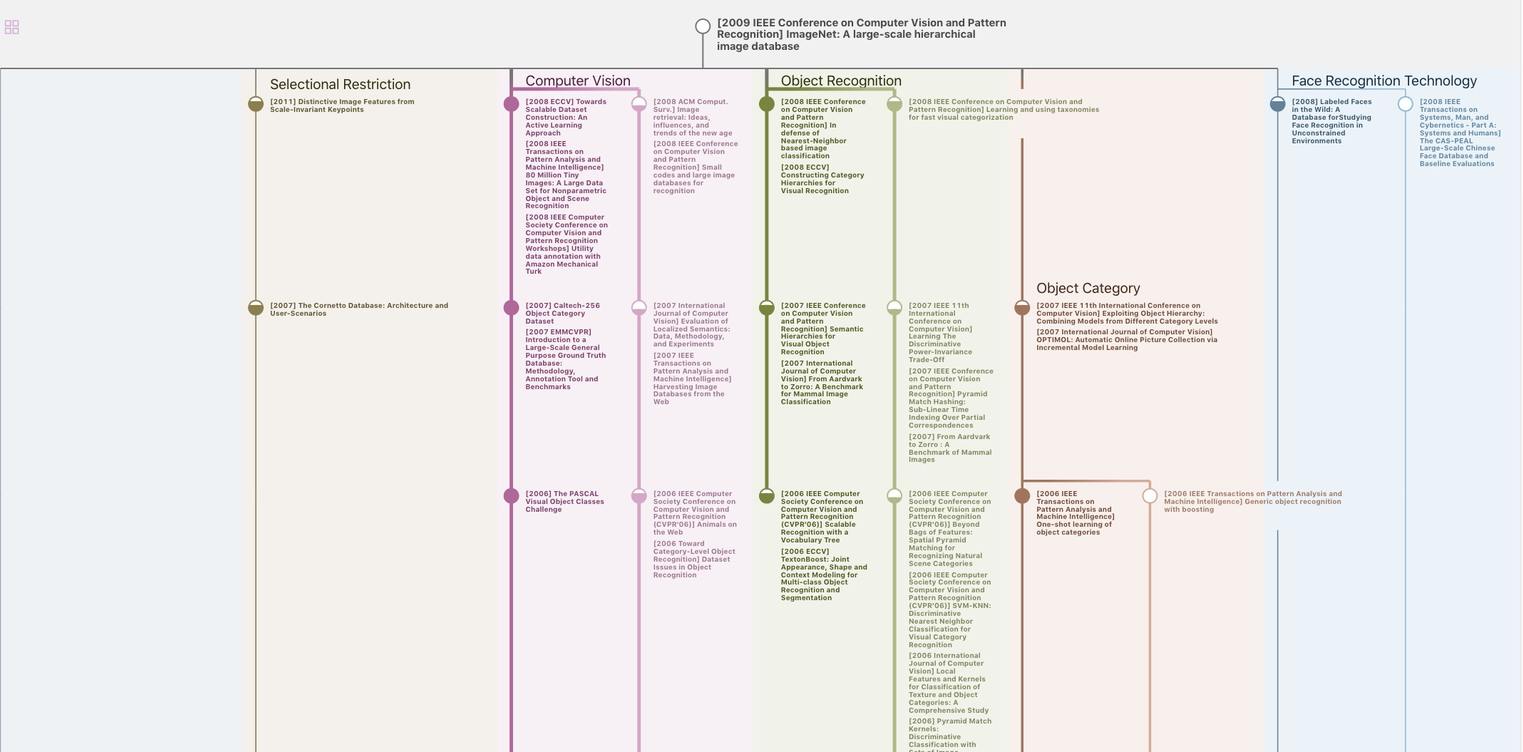
生成溯源树,研究论文发展脉络
Chat Paper
正在生成论文摘要