Clustering-Based Anomaly Detection In Multivariate Time Series Data
APPLIED SOFT COMPUTING(2021)
摘要
Multivariate time series data come as a collection of time series describing different aspects of a certain temporal phenomenon. Anomaly detection in this type of data constitutes a challenging problem yet with numerous applications in science and engineering because anomaly scores come from the simultaneous consideration of the temporal and variable relationships. In this paper, we propose a clustering-based approach to detect anomalies concerning the amplitude and the shape of multivariate time series. First, we use a sliding window to generate a set of multivariate subsequences and thereafter apply an extended fuzzy clustering to reveal a structure present within the generated multivariate subsequences. Finally, a reconstruction criterion is employed to reconstruct the multivariate subsequences with the optimal cluster centers and the partition matrix. We construct a confidence index to quantify a level of anomaly detected in the series and apply Particle Swarm Optimization as an optimization vehicle for the problem of anomaly detection. Experimental studies completed on several synthetic and six real-world datasets suggest that the proposed methods can detect the anomalies in multivariate time series. With the help of available clusters revealed by the extended fuzzy clustering, the proposed framework can detect anomalies in the multivariate time series and is suitable for identifying anomalous amplitude and shape patterns in various application domains such as health care, weather data analysis, finance, and disease outbreak detection.(c) 2020 Elsevier B.V. All rights reserved.
更多查看译文
关键词
Fuzzy C-Means (FCM), Anomaly detection, Multivariate time series, Reconstruction criterion
AI 理解论文
溯源树
样例
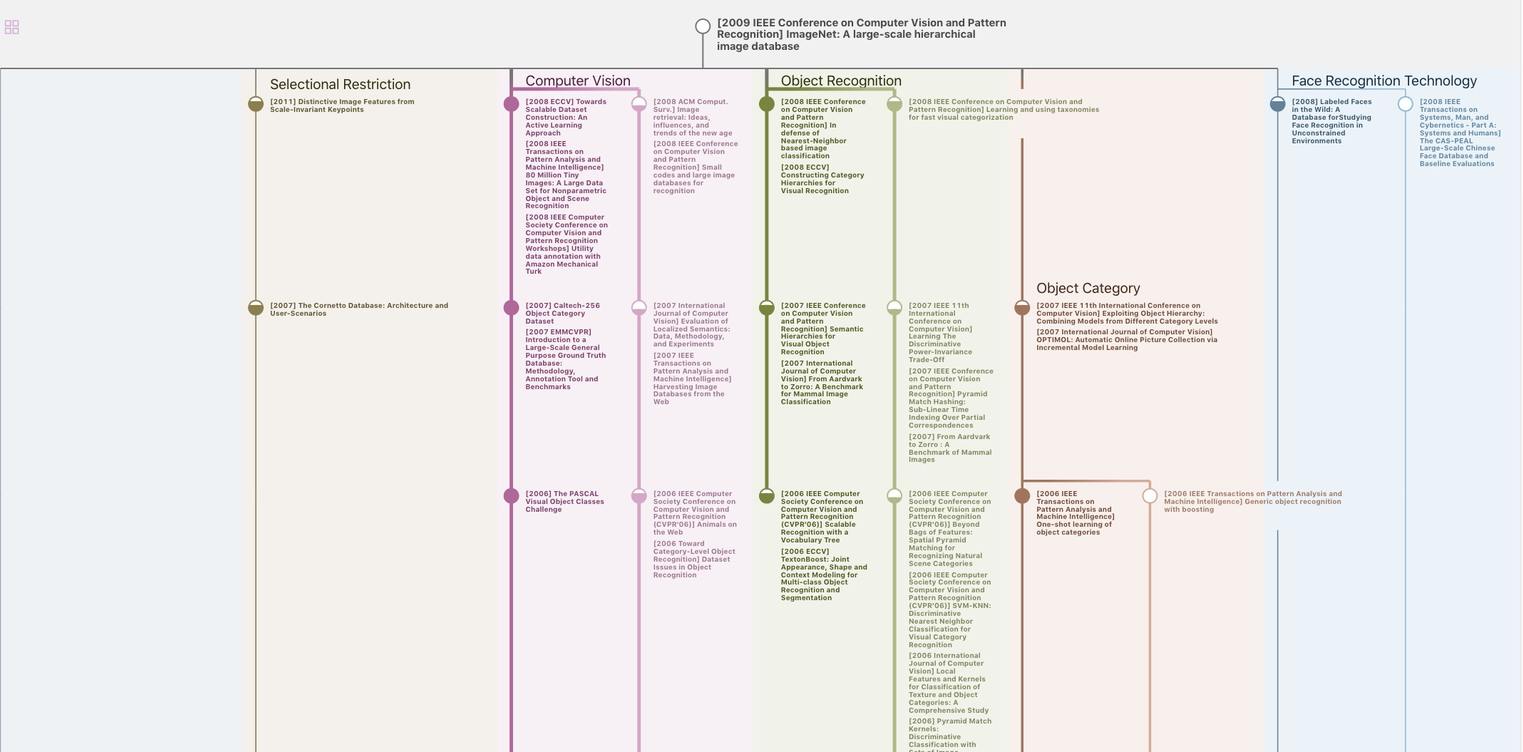
生成溯源树,研究论文发展脉络
Chat Paper
正在生成论文摘要