Entropy Repulsion for Semi-supervised Learning Against Class Mismatch.
ICONIP (2)(2020)
摘要
A series of semi-supervised learning (SSL) algorithms have been proposed to alleviate the need for labeled data by leveraging large amounts of unlabeled data. Those algorithms have achieved good performance on standard benchmark datasets, however, their performance can degrade drastically when there exists a class mismatch between the labeled and unlabeled data, which is common in practice. In this work, we propose a new technique, entropy repulsion for mismatch (ERCM), to improve SSL against a class mismatch situation. Specifically, we design an entropy repulsion loss and a batch annealing and reloading mechanism, which work together to prevent potentially mismatched unlabeled data from participating in the early training stages as well as facilitate the minimization of the unsupervised loss term of traditional SSL algorithms. ERCM can be adopted to enhance existing SSL algorithms with minor extra computation cost and no change to their network structures. Our extensive experiments demonstrate that ERCM can significantly improve the performance of state-of-the-art SSL algorithms, namely Mean Teacher, Virtual Adversarial Training (VAT) and Mixmatch in various class-mismatch cases.
更多查看译文
关键词
class mismatch,entropy,learning,semi-supervised
AI 理解论文
溯源树
样例
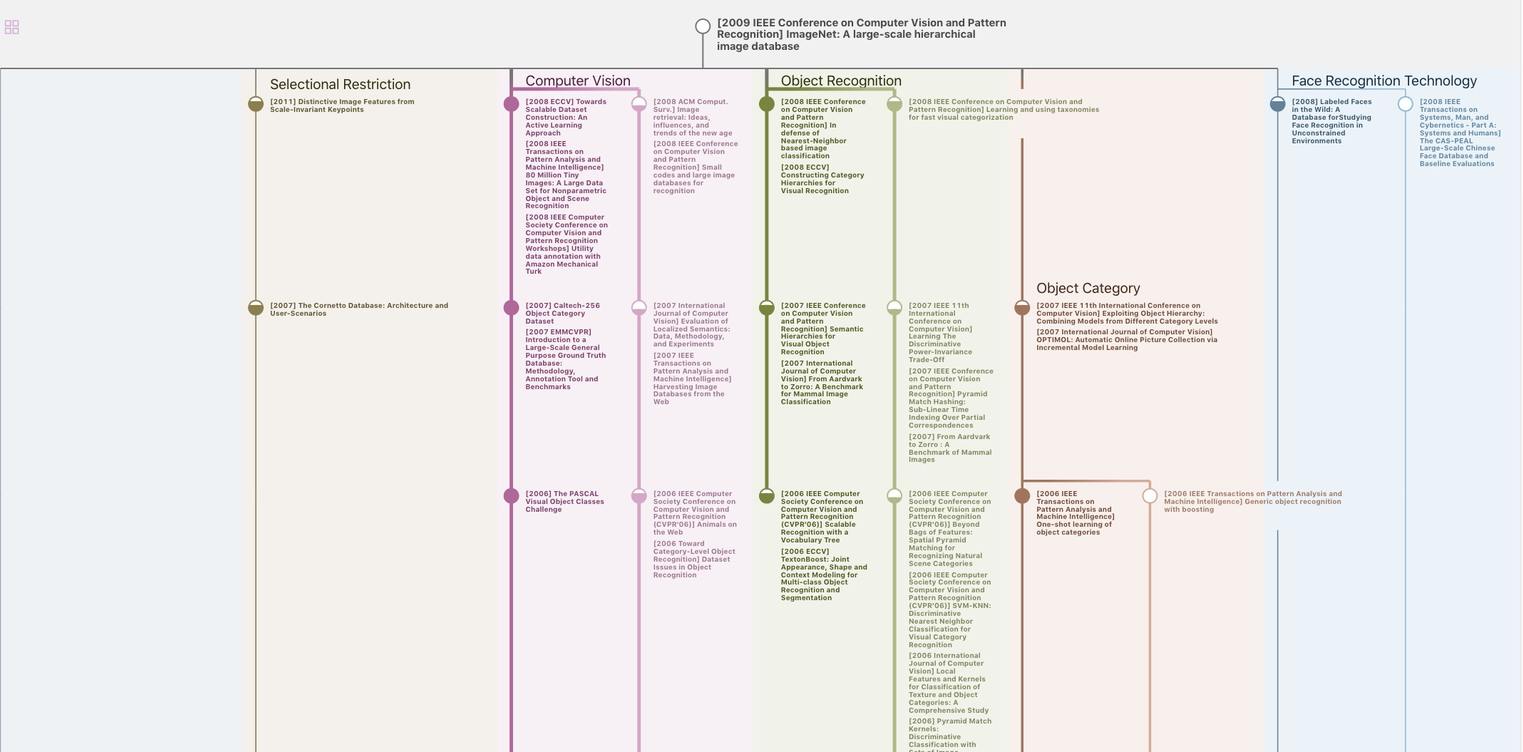
生成溯源树,研究论文发展脉络
Chat Paper
正在生成论文摘要