Uncouple Generative Adversarial Networks For Transferring Stylized Portraits To Realistic Faces
IEEE ACCESS(2020)
摘要
Stylized portraits widely exist in artwork or paintings. It is interesting to restore the original identity of portrait artworks, but consider that the rarity and the style diversity of these artworks, it is difficult to pair and obtain sufficient training data to restore their original identities by existing methods. Therefore, it is challenging to explore a method to restore a single stylized portrait to its original identity. Although CycleGAN can convert paintings into realistic photographs in unpaired datasets, it was not developed specifically for portraits, and photo-realistic faces require more accurate structures, thus the visual results obtained with CycleGAN are not satisfactory. In this paper, we propose Uncouple-Generative Adversarial Networks (UncGANs) for transferring stylized portraits to realistic faces. Our UncGANs framework is inspired by CariGANs to tackle the visual problem in CycleGAN for obtaining realistic faces from stylized portraits. In addition, we introduce three losses, namely, the semantic style consistency loss and the cycle consistency loss to effectively guide the training of generators and discriminators on unpaired datasets, the global and local adversarial loss ensure the consistency of appearance characteristics before and after translation, and the location consistency loss to establish the precise correspondence between the source domain and the target domain as well as assist the discriminators. Extensive experimental results and comparisons with state-of-the-art methods including Style, Deep-Image-Analogy, UNIT, MUNIT, CycleGAN, CP-GAN, and PS2-MAN demonstrate that our framework is better at generating realistic faces from stylized portraits with accurate structures and features.
更多查看译文
关键词
Faces, Generators, Task analysis, Image restoration, Semantics, Training, Painting, Stylized portraits, reality, generative adversarial networks (GANs), deep learning
AI 理解论文
溯源树
样例
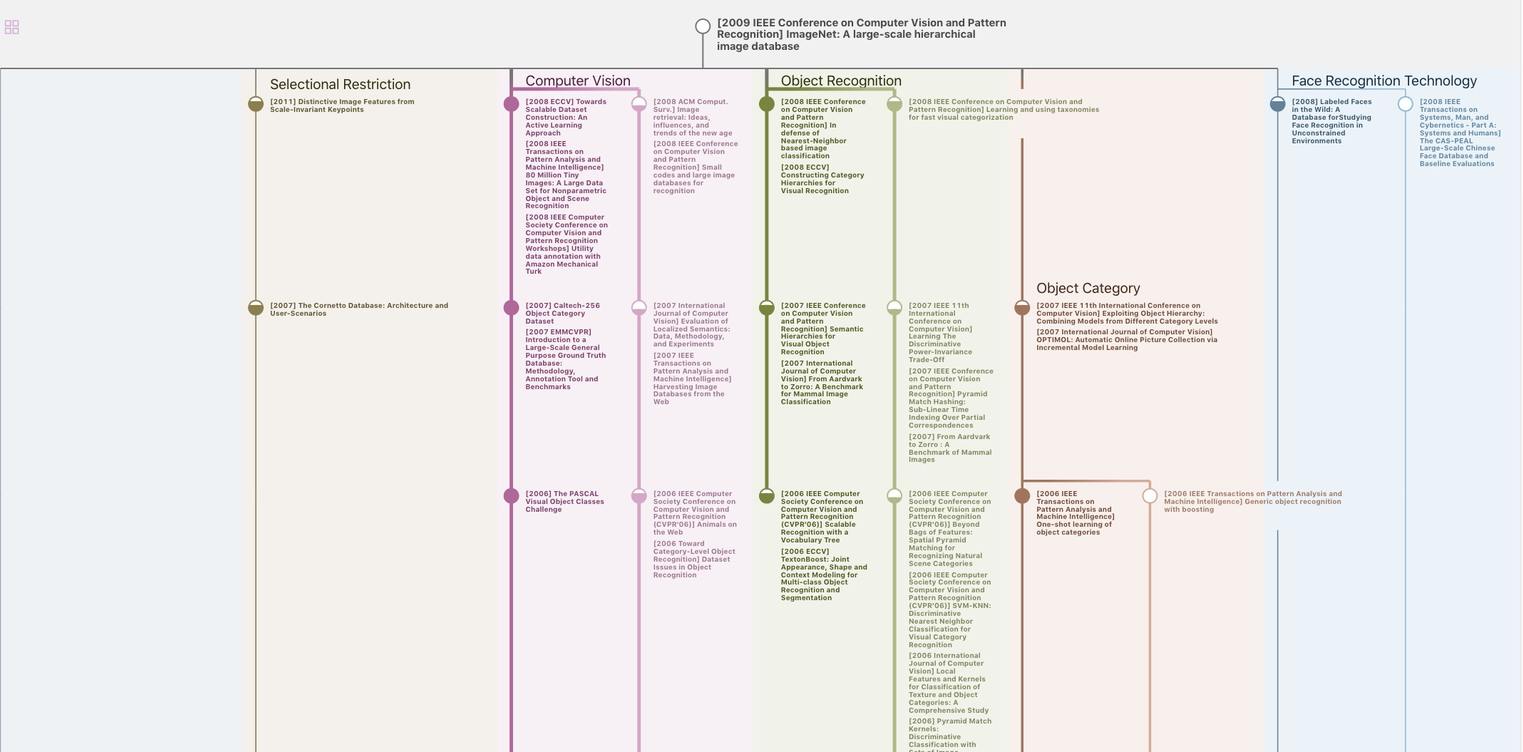
生成溯源树,研究论文发展脉络
Chat Paper
正在生成论文摘要