Adaptive Adversarial Latent Space For Novelty Detection
IEEE ACCESS(2020)
摘要
Novelty detection is a challenging task of identifying whether a new sample obeys to a known class. Note that the boundary between normal and novel is not clear enough in existing works, resulting from adequately reconstructing novel samples or crudely reconstructing normal samples. To tackle the above issues, we propose a general framework named Adaptive Adversarial Latent Space (AALS), which mainly consists of two components, Adaptive Latent Space Generator (ALSG) and Constrained-based Adversarial AutoEncoder (CAAE). ALSG is established to obtain the real latent space distribution by adaptive mapper and the manner of adversarial learning. Moreover, CAAE is presented to obtain a better boundary between normal and novel by Global and Local Channel-wise Attention (GLCA), which is proposed to reweight the global and local information in different channels. Given a sample, the latent space representation z of the sample is firstly obtained by encoder with GLCA. Next, the output of ALSG is applied to constrain the latent space by latent adversarial loss. Further, z is transformed into the decoder to reconstruct the given sample with GLCA. Experiments on three available datasets demonstrates the effectiveness of the proposed method, which achieves state-of-the-art performance compared with other methods.
更多查看译文
关键词
Novetly detection, adaptive latent space, latent constraint, channel attention
AI 理解论文
溯源树
样例
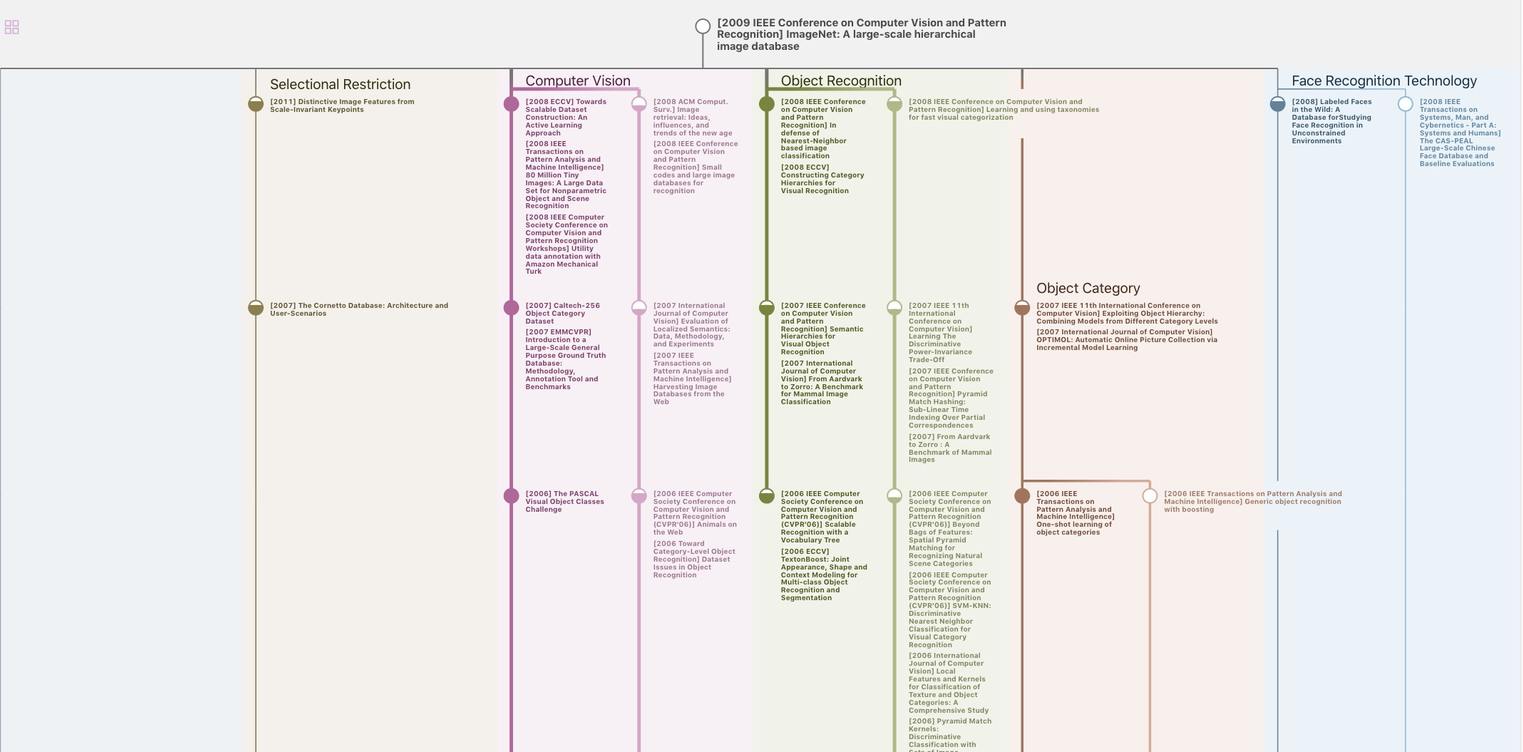
生成溯源树,研究论文发展脉络
Chat Paper
正在生成论文摘要