Why are target absent searches so systematic?
Journal of Vision(2020)
摘要
We all know that finding a target is easy if the distractors are dissimilar. But what about when there is no target? Do target-absent responses vary systematically too? Surprisingly there have not been any attempts to answer this question. Here we set out to investigate this question using a combination of behavioral experiments in humans and recordings of single neurons in monkey inferior temporal cortex. In Experiment 1, subjects had to view a search array and indicate whether any oddball target is present or absent. Target absent times were highly systematic, as evidenced by a strong split-half correlation across subjects (r = 0.75, p < 0.0005). We hypothesized that the target-absent search time might depend on how distinctive an object is compared to other objects. We measured the pairwise dissimilarity between all pairs of images in Experiment 2. For each object, we measured its distinctiveness as its average distance from all other objects in the experiment. This quantity, derived from target-present search times, was strongly predictive of the target-absent search time (r = -0.77, p < 0.0005). In Experiment 3, we showed that target-absent search times are independent of context, suggesting that they are driven by a universal rather than a context-dependent computation. Finally, we wondered how distinctiveness might be calculated in the brain, since it is unlikely that the neural response to a given (viewed) object can be compared with the response to many other objects not currently visible. To address this issue, we asked whether distinctiveness can be predicted by the activity of a population of neurons responding to a single object. Indeed, a weighted absolute difference computation was strongly predictive of distinctiveness. Taken together our results highlight a universal variance computation on objects, which we call distinctiveness, that systematically predicts absent-search times.
更多查看译文
关键词
absent searches,target
AI 理解论文
溯源树
样例
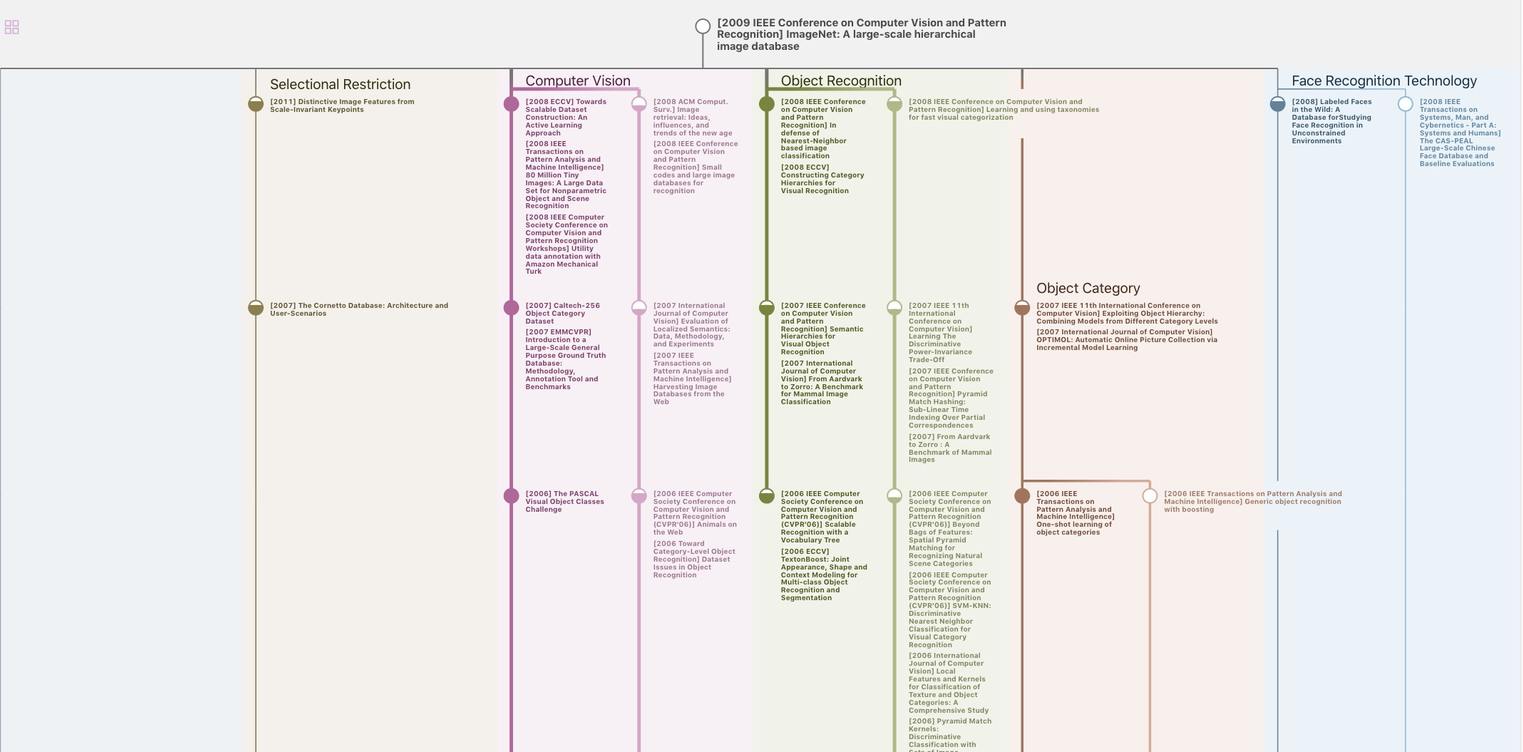
生成溯源树,研究论文发展脉络
Chat Paper
正在生成论文摘要