Multi-Label Emotion Detection via Emotion-Specified Feature Extraction and Emotion Correlation Learning
IEEE Transactions on Affective Computing(2023)
摘要
Textual emotion detection is an attractive task while previous studies mainly focused on polarity or single-emotion classification. However, human expressions are complex, and multiple emotions often co-occur with non-negligible emotion correlations. In this paper, a Multi-label Emotion Detection Architecture (MEDA) is proposed to detect all associated emotions expressed in a given piece of text. MEDA is mainly composed of two modules: Multi-Channel Emotion-Specified Feature Extractor (MC-ESFE) and Emotion Correlation Learner (ECorL). MEDA captures underlying emotion-specified features through MC-ESFE module, which is composed of multiple channel-wise ESFE networks. Each channel in MC-ESFE is devoted to the feature extraction of a specified emotion from sentence-level to context-level through a hierarchical structure. With underlying features, emotion correlation learning is implemented through an emotion sequence predictor in ECorL. Furthermore, we define a new loss function: multi-label focal loss. With this loss function, the model can focus more on misclassified positive-negative emotion pairs and improve the overall performance by balancing the prediction of positive and negative emotions. The evaluation of proposed MEDA architecture is carried out on emotional corpus: RenCECps and NLPCC2018 datasets. The experimental results indicate that the proposed method can achieve better performance than state-of-the-art methods in this task.
更多查看译文
关键词
Multi-label,emotion detection,emotion correlation,multi-label focal loss
AI 理解论文
溯源树
样例
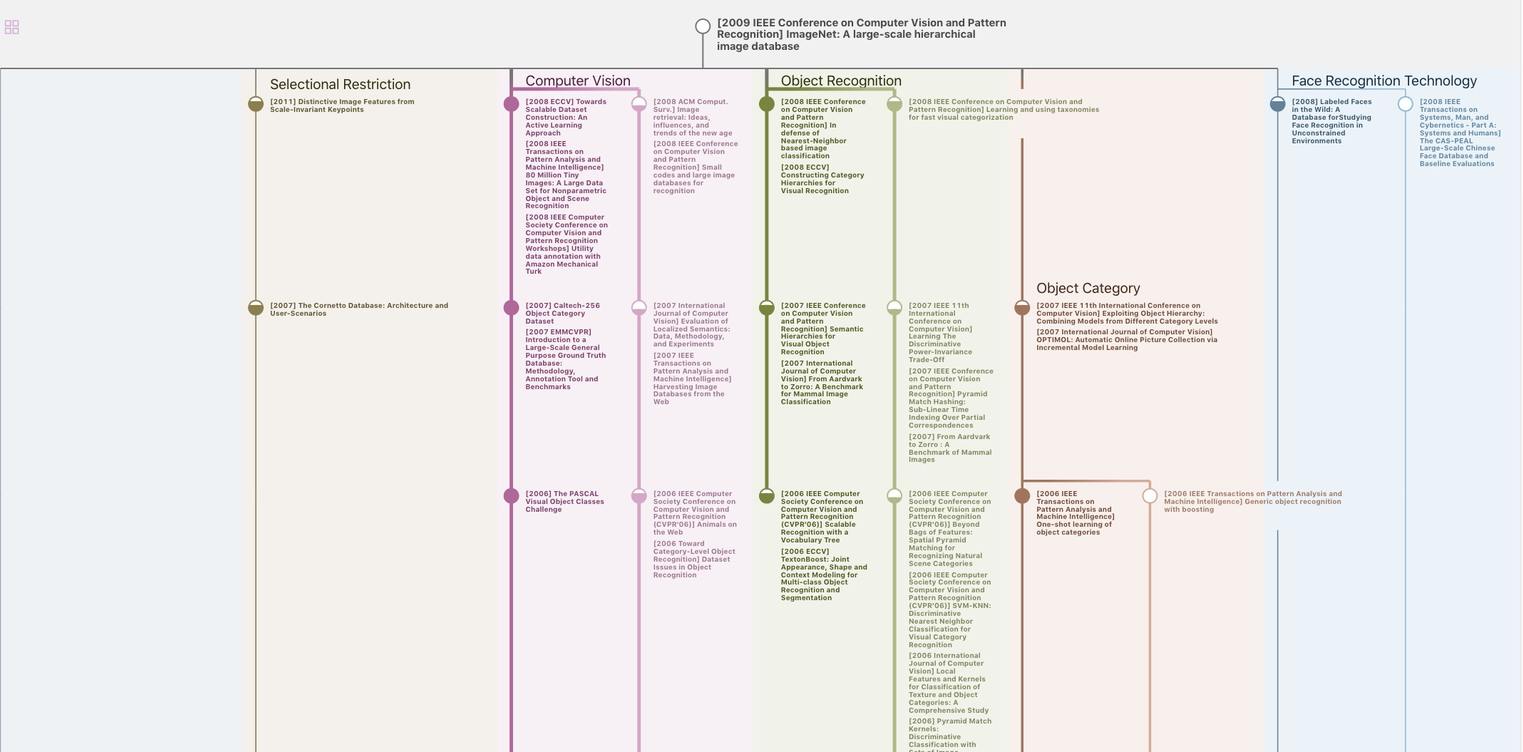
生成溯源树,研究论文发展脉络
Chat Paper
正在生成论文摘要