Accelerated 4D Respiratory Motion-Resolved Cardiac MRI with a Model-Based Variational Network.
medical image computing and computer assisted intervention(2020)
摘要
Respiratory motion and long scan times remain major challenges in free-breathing 3D cardiac MRI. Respiratory motion-resolved approaches have been proposed by binning the acquired data to different respiratory motion states. After inter-bin motion estimation, motion-compensated reconstruction can be obtained. However, respiratory bins from accelerated acquisitions are highly undersampled and have different undersampling patterns depending on the subject-specific respiratory motion. Remaining undersampling artifacts in the bin images can influence the accuracy of the motion estimation. We propose a model-based variational network (VN) which reconstructs motion-resolved images jointly by exploiting shared information between respiratory bins. In each stage of VN, conjugate gradient is adopted to enforce data-consistency (CG-VN), achieving better enforcement of data consistency per stage than the classic VN with proximal gradient descent step (GD-VN), translating to faster convergence and better reconstruction performance. We compare the performance of CG-VN and GD-VN for reconstruction of respiratory motion-resolved images for two different cardiac MR sequences. Our results show that CG-VN with less stages outperforms GD-VN by achieving higher PSNR and better generalization on prospectively undersampled data. The proposed motion-resolved CG-VN provides consistently good reconstruction quality for all motion states with varying undersampling patterns by taking advantage of redundancies among motion bins.
更多查看译文
关键词
cardiac mri,motion-resolved,model-based
AI 理解论文
溯源树
样例
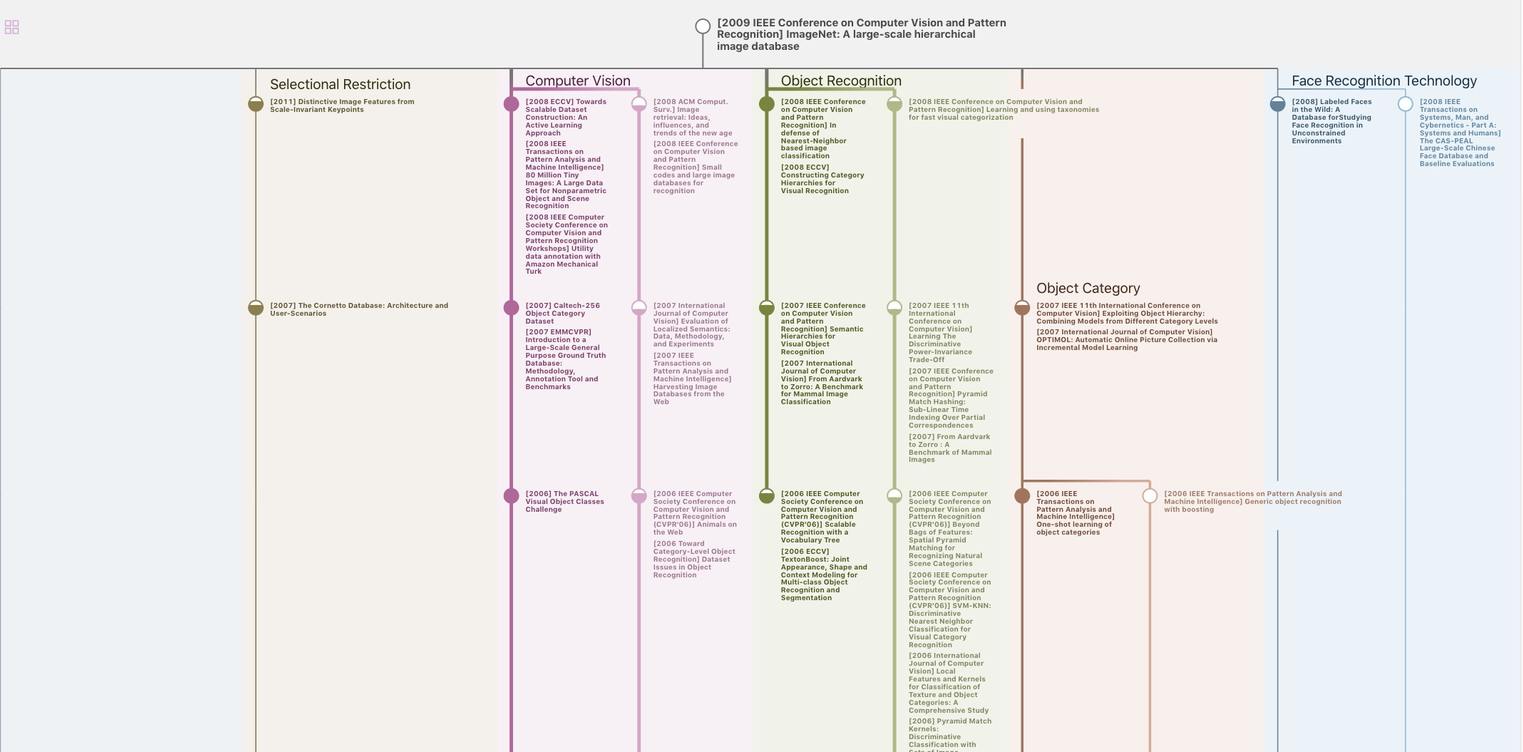
生成溯源树,研究论文发展脉络
Chat Paper
正在生成论文摘要