Prediction of Pathological Complete Response to Neoadjuvant Chemotherapy in Breast Cancer Using Deep Learning with Integrative Imaging, Molecular and Demographic Data.
medical image computing and computer-assisted intervention(2020)
摘要
Neoadjuvant chemotherapy is widely used to reduce tumor size to make surgical excision manageable and to minimize distant metastasis. Assessing and accurately predicting pathological complete response is important in treatment planing for breast cancer patients. In this study, we propose a novel approach integrating 3D MRI imaging data, molecular data and demographic data using convolutional neural network to predict the likelihood of pathological complete response to neoadjuvant chemotherapy in breast cancer. We take post-contrast T1-weighted 3D MRI images without the need of tumor segmentation, and incorporate molecular subtypes and demographic data. In our predictive model, MRI data and non-imaging data are convolved to inform each other through interactions, instead of a concatenation of multiple data type channels. This is achieved by channel-wise multiplication of the intermediate results of imaging and non-imaging data. We use a subset of curated data from the I-SPY-1 TRIAL of 112 patients with stage 2 or 3 breast cancer with breast tumors underwent standard neoadjuvant chemotherapy. Our method yielded an accuracy of 0.83, AUC of 0.80, sensitivity of 0.68 and specificity of 0.88. Our model significantly outperforms models using imaging data only or traditional concatenation models. Our approach has the potential to aid physicians to identify patients who are likely to respond to neoadjuvant chemotherapy at diagnosis or early treatment, thus facilitate treatment planning, treatment execution, or mid-treatment adjustment.
更多查看译文
关键词
neoadjuvant chemotherapy,breast cancer,deep learning,integrative imaging
AI 理解论文
溯源树
样例
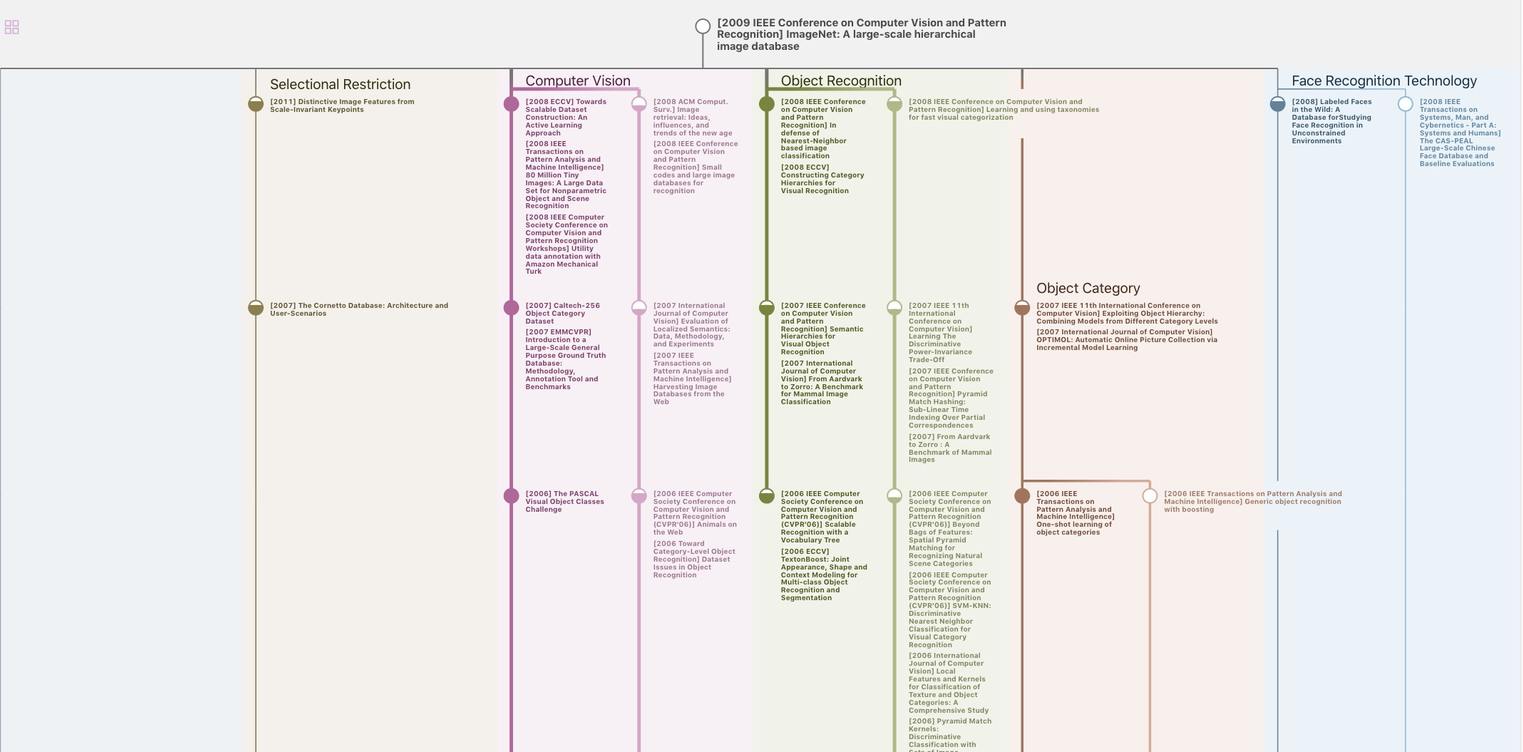
生成溯源树,研究论文发展脉络
Chat Paper
正在生成论文摘要