A novel attribute-based generation architecture for facial image editing
MULTIMEDIA TOOLS AND APPLICATIONS(2020)
摘要
Facial image editing is one of the hot topics in recent years due to the great development in deep generative models. Current models are either based on variational autoencoder(VAE) or generative adversarial network(GAN). However, VAE-based models usually generate oversmooth images, while GAN-based-only models cannot randomly generate images with specific attributes and suffer from unstable training. To overcome these limitations, a novel attribute-disentangled generative model based on the combination of VAE and GAN is proposed for facial image editing by manipulating specific attributes and synthesizing facial images conditioned on the specified attributes. In the encoder-decoder architecture of the proposed model, the latent space mapped by the encoder is split into two subspaces: the attribute-irrelevant space and the attribute-relevant space. The attribute-irrelevant space characterizes the factors such as identity, position, background etc, which are expected to be kept unchanged during the editing. The attribute-relevant space is used to represent the attributes such as hair color, gender, age etc that we want to manipulate. We use the adversarial training scheme to train the model, where images generated by the proposed model are re-feeded to the encoder to ensure their distribution is close to the real data distribution in the attribute-irrelevant subspace while they can be correctly classified in the attribute-relevant subspace, without explicitly giving the discriminators such as in GANs. To evaluate the performance of the proposed model, quantitative and qualitative comparisons between the proposed model and other state-of-the-art algorithms were tesed on the CelebA dataset. The evaluation results show that the proposed model can effectively generate high-quality facial images with diverse specified attributes.
更多查看译文
关键词
Adversarial Variational Autoencoders,Generative Adversarial Networks,Facial editing,Disentangled features
AI 理解论文
溯源树
样例
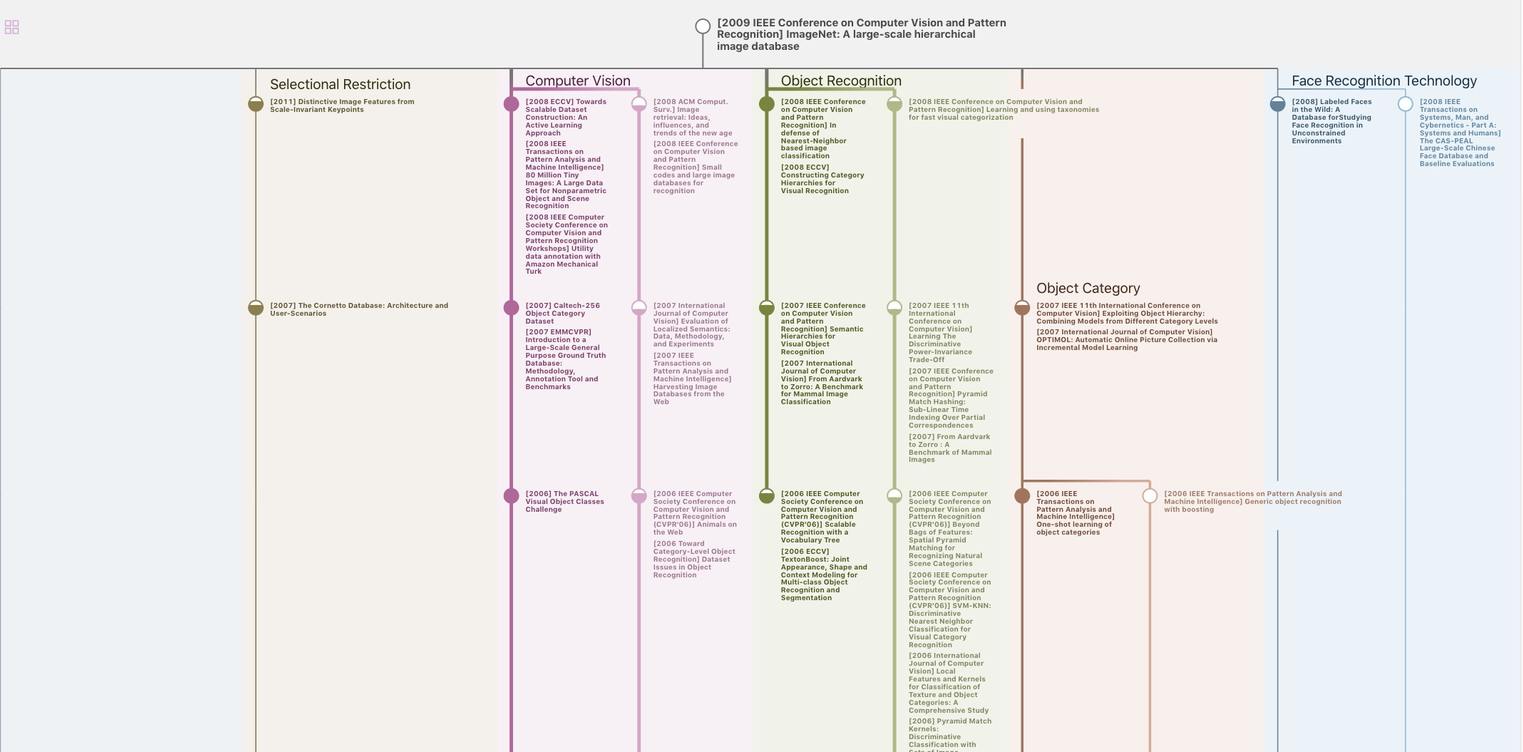
生成溯源树,研究论文发展脉络
Chat Paper
正在生成论文摘要