Data-Driven Multi-contrast Spectral Microstructure Imaging with InSpect
medical image computing and computer assisted intervention(2020)
摘要
We introduce and demonstrate an unsupervised machine learning method for spectroscopic analysis of quantitative MRI (qMRI) experiments. qMRI data can support estimation of multidimensional correlation (or single-dimensional) spectra, which allow model-free investigation of tissue properties, but this requires an ill-posed calculation. Moreover, in the vast majority of applications ground truth knowledge is unobtainable, preventing the application of supervised machine learning. Here we present a new method that addresses these limitations in a data-driven way. The algorithm simultaneously estimates a canonical basis of spectral components and voxelwise maps of their weightings, thereby pooling information across whole images to regularise the ill-posed problem. We show that our algorithm substantially outperforms current voxelwise spectral approaches. We demonstrate the method on combined diffusion-relaxometry placental MRI scans, revealing anatomically-relevant substructures, and identifying dysfunctional placentas. Our algorithm vastly reduces the data required to reliably estimate multidimensional correlation (or single-dimensional) spectra, opening up the possibility of spectroscopic imaging in a wide range of new applications.
更多查看译文
关键词
spectral component estimation,imaging,microstructure,data-driven,multi-contrast
AI 理解论文
溯源树
样例
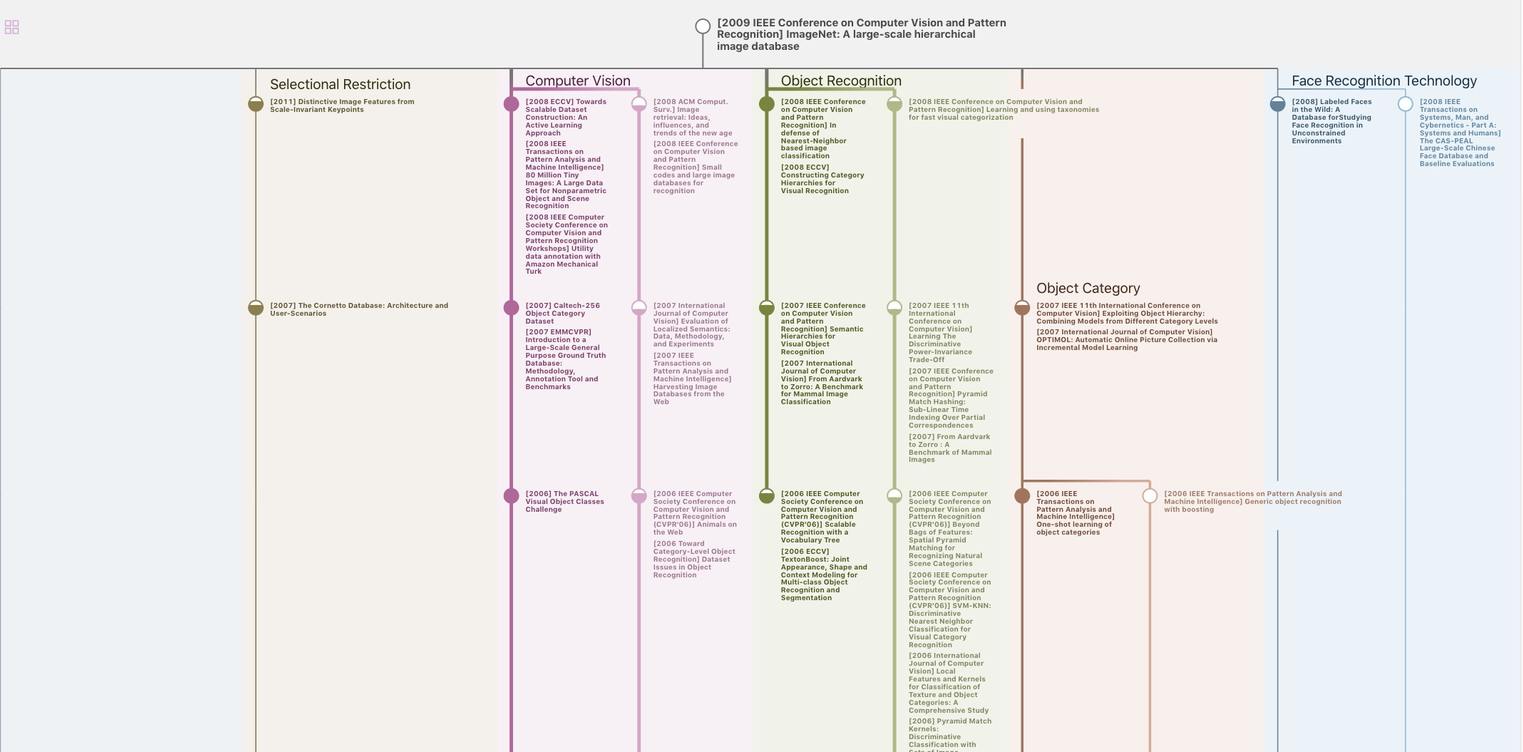
生成溯源树,研究论文发展脉络
Chat Paper
正在生成论文摘要