Deep Generative Model for Synthetic-CT Generation with Uncertainty Predictions
medical image computing and computer assisted intervention(2020)
摘要
MR-only radiation treatment planning is attractive due to the superior soft tissue definition of MRI as compared to CT, and the elimination of the uncertainty introduced by CT-MRI registration. To facilitate MR-only radiation therapy planning, synthetic-CT (sCT) algorithms (for electron density correction) are required for dose calculation. Deep neural networks for sCT generation are useful due to their predictive power, but lack of uncertainty information is a concern for clinical implementation. The feasibility of using a conditional generative adversarial model (cGAN) to generate sCTs with accompanying uncertainty maps was investigated. Dropout-based variational inference was used to account for uncertainty in the trained model. The cGAN loss function was also combined with an additional term such that the network learns which regions of input data are associated with highly variable outputs. On a dataset of 105 brain cancer patients, our results demonstrate that the network generates well-calibrated uncertainty predictions and produces sCTs with equivalent accuracy as previously reported deterministic models.
更多查看译文
关键词
Generative adversarial networks, MR-only radiation therapy, Uncertainty
AI 理解论文
溯源树
样例
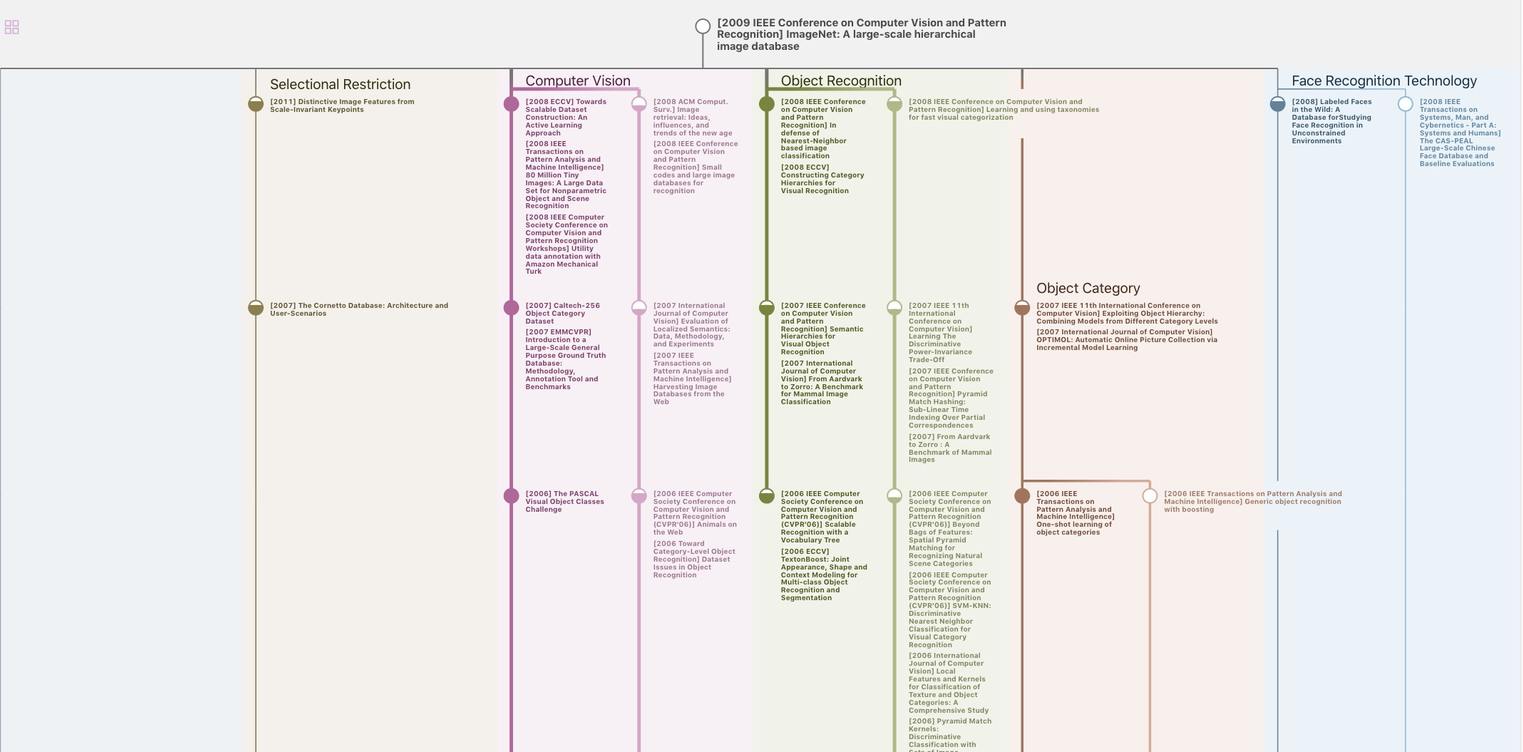
生成溯源树,研究论文发展脉络
Chat Paper
正在生成论文摘要