A Variation Robust Inference Engine Based on STT-MRAM with Parallel Read-Out
ISCAS(2020)
摘要
STT-MRAM is a promising candidate as embedded non-volatile memory (NVM) at 28nm and beyond. Due to its limited on/off ratio, STT-MRAM is often used as digital memory that only allows row-by-row read-out for near-memory computing. This work proposes design strategies to overcome this limitation with a new bit-cell design to enable parallel read-out for in-memory computing, which is of great interests for deep neural network (DNN) acceleration. We consider the non-ideal device properties that degrade inference accuracy including small on/off ratio, cell-to-cell MTJ conductance variation and current sense amplifier (CSA) offset. We propose three techniques to minimize inference accuracy degradation: 1) a 2T-2MTJ bit-cell design with high on/off ratio, 2) redundancy for MSB weights to mitigate the impact of MTJ conductance variations, and 3) a hybrid-layer mapping scheme to reduce column current thus mitigating CSA offset effect. DNN benchmarking results show that on CIFAR-10 dataset, the inference accuracy can be maintained at > 90% in the presence of 10% MTJ conductance variations, and >87.5% after considering CSA offset effect, with minimal 8% energy and 4% chip area overhead.
更多查看译文
关键词
DNN,In-memory computing,STT-MRAM
AI 理解论文
溯源树
样例
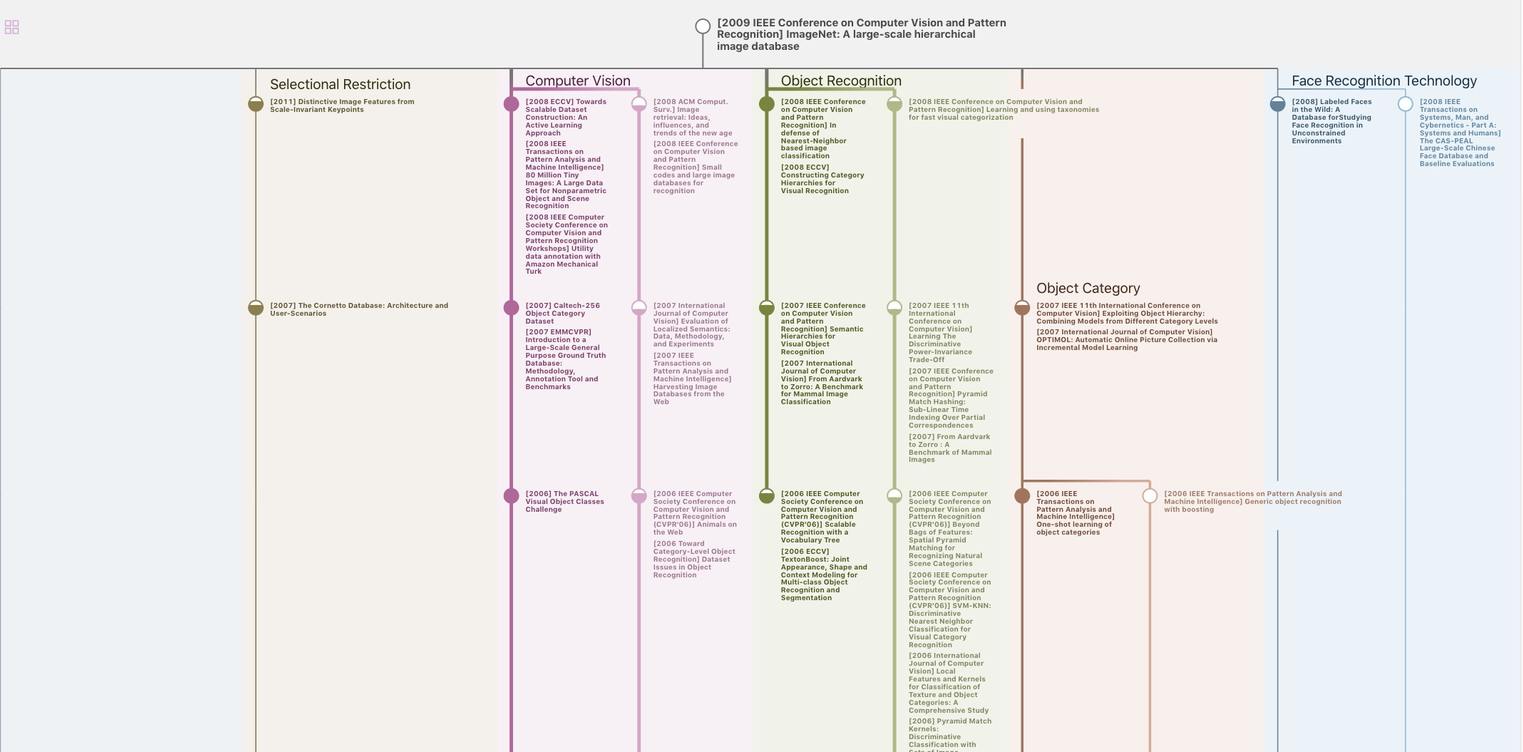
生成溯源树,研究论文发展脉络
Chat Paper
正在生成论文摘要