A Graph-Based Approach To Interpreting Recurrent Neural Networks In Process Mining
IEEE ACCESS(2020)
摘要
Process mining is often used by organisations to audit their business processes and improve their services and customer relations. Indeed, process execution (or event) logs constantly generated through various information systems can be employed to derive valuable insights about business operations. Compared to traditional process mining techniques such as Petri nets and the Business Process Model and Notation (BPMN), deep learning methods such as Recurrent Neural Networks, and Long Short-Term Memory (LSTM) in particular, have proven to achieve a better performance in terms of accuracy and generalising ability when predicting next events in business processes. However, unlike the traditional network-based process mining techniques that can be used to visually present the entire discovered process, the existing deep learning-based methods for process mining lack a mechanism explaining how the predictions of next events are made. This study proposes a new approach to process mining by combining the benefits of the earlier, visually explainable graph-based methods and later, more accurate but unexplainable deep learning methods. According to the proposed approach, an LSTM model is employed first to find probabilities for each known event to appear in the process next. These probabilities are then used to generate a visually interpretable process model graph that represents the decision-making process of the LSTM model. The level of detail in this graph can be adjusted using a probability threshold, allowing to address a range of process mining tasks such as business process discovery and conformance checking. The advantages of the proposed approach over existing LSTM-based process mining methods in terms of both accuracy and explainability are demonstrated using real-world event logs.
更多查看译文
关键词
Unified modeling language, Machine learning, Task analysis, Recurrent neural networks, Predictive models, Process control, Directly-follows graph, explainable AI, long short term memory, process mining, recurrent neural network
AI 理解论文
溯源树
样例
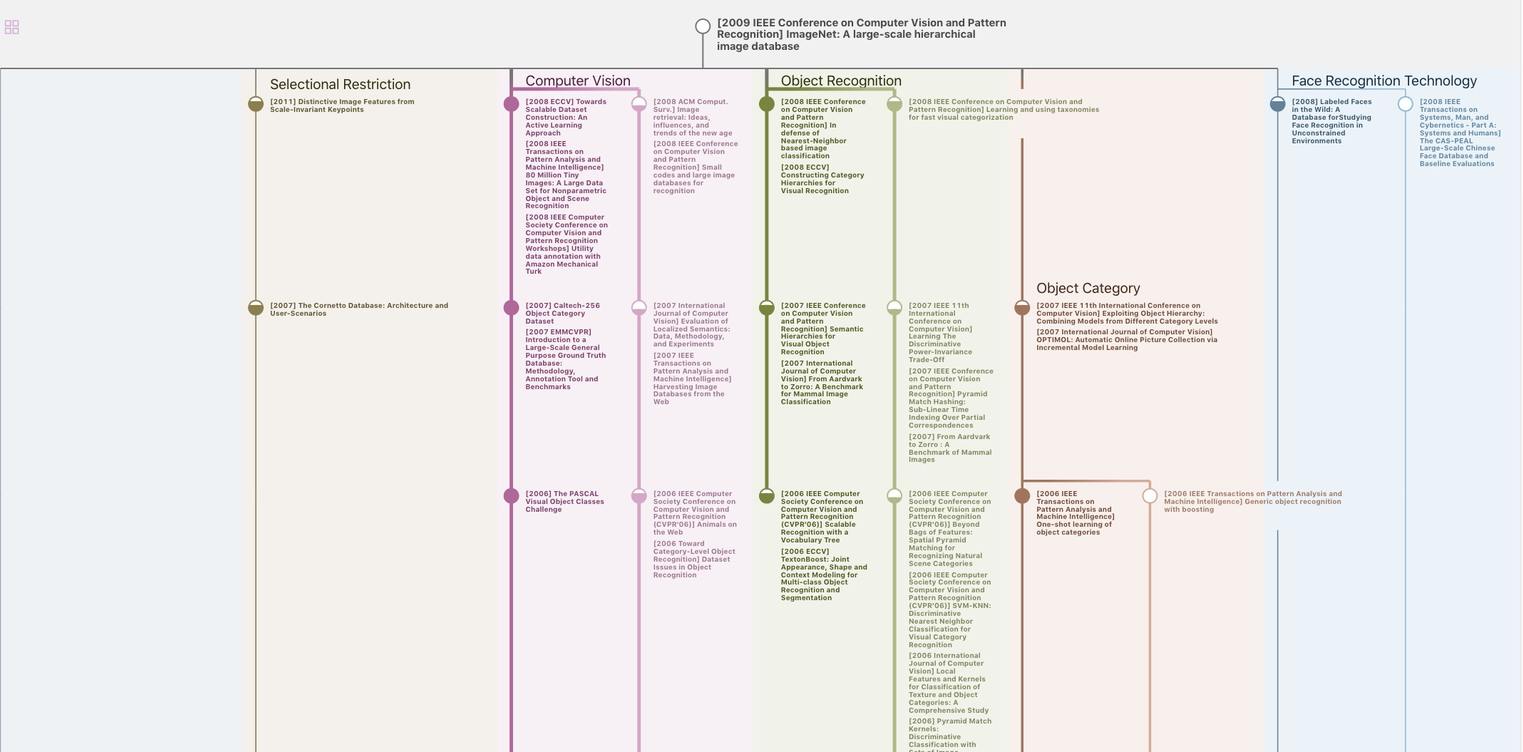
生成溯源树,研究论文发展脉络
Chat Paper
正在生成论文摘要