Explaining automated gender classification of human gait
Gait & Posture(2020)
摘要
State-of-the-art machine learning (ML) models are highly effective in classifying gait analysis data, however, they lack in providing explanations for their predictions. This "black-box" characteristic makes it impossible to understand on which input patterns, ML models base their predictions. The present study investigates whether Explainable Artificial Intelligence methods, i.e., Layer-wise Relevance Propagation (LRP), can be useful to enhance the explainability of ML predictions in gait classification. The research question was: Which input patterns are most relevant for an automated gender classification model and do they correspond to characteristics identified in the literature? We utilized a subset of the GAITREC dataset containing five bilateral ground reaction force (GRF) recordings per person during barefoot walking of 62 healthy participants: 34 females and 28 males. Each input signal (right and left side) was min-max normalized before concatenation and fed into a multi-layer Convolutional Neural Network (CNN). The classification accuracy was obtained over a stratified ten-fold cross-validation. To identify gender-specific patterns, the input relevance scores were derived using LRP. The mean classification accuracy of the CNN with 83.3% showed a clear superiority over the zero-rule baseline of 54.8%.
更多查看译文
AI 理解论文
溯源树
样例
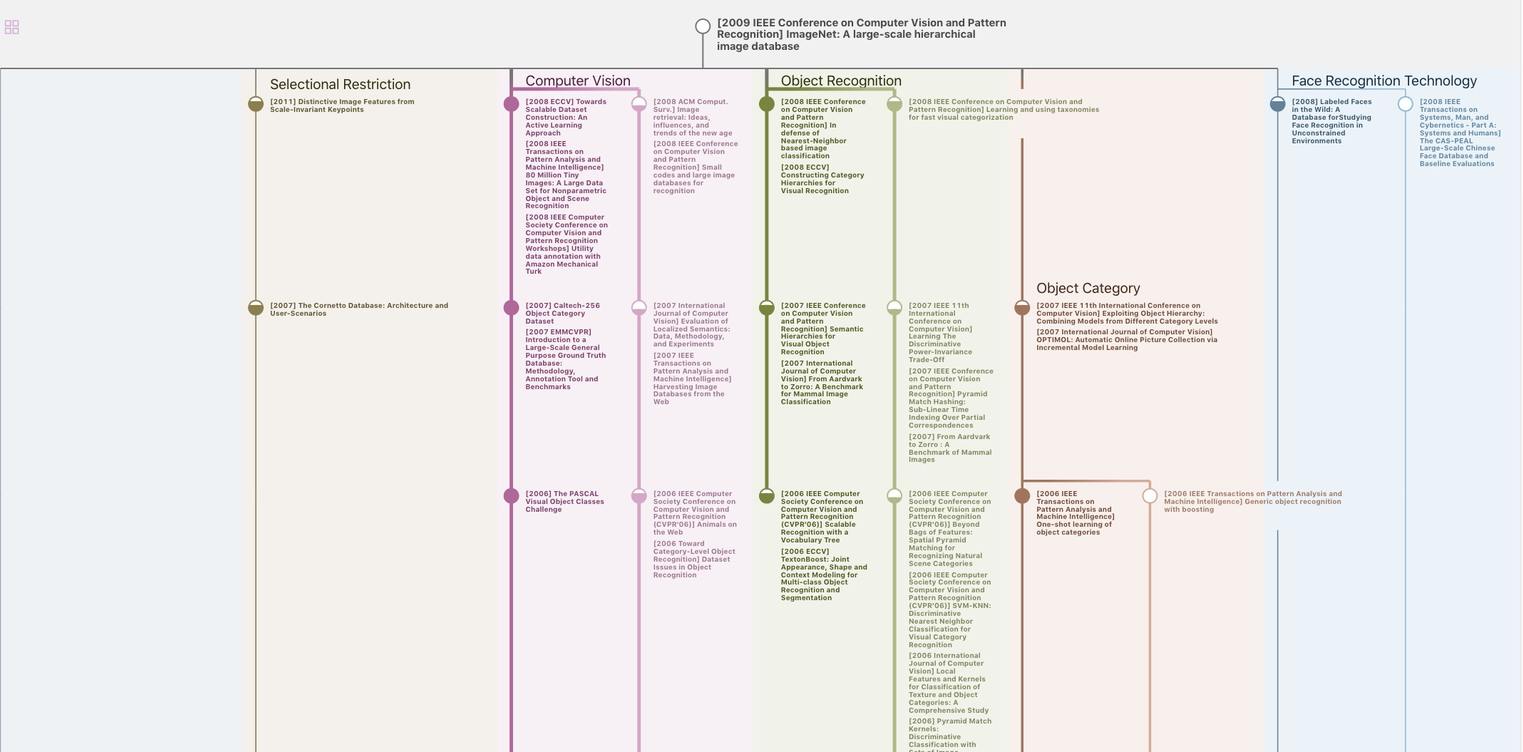
生成溯源树,研究论文发展脉络
Chat Paper
正在生成论文摘要