Modeling Price and Risk in Chinese Financial Derivative Market with Deep Neural Network Architectures
2020 5th International Conference on Computational Intelligence and Applications (ICCIA)(2020)
摘要
As rapid growth, Chinese financial derivative market is holding increasingly large proportions in entire domestic capital market as well as in global shares. To the nature of derivative instruments, plenty of market data features (such as prices and trading volumes) and off-market factors (such as financial news and policies) can directly impact on the price and risk in Chinese financial derivative markets, which is becoming more and more infeasible to model by using only traditional financial models and hand-crafted features. To alleviate the issue, in this paper we introduce some state-of-art deep neural network architectures and model two significant futures market price and risk indicators that are widely used by Chinese regulators, which are turn-over ratio (ratio of daily trading volumes and daily open interest volumes) and price basis (gap between futures price and corresponding spot product price). The extensive experimental results show that deep learning methods perform better prediction accuracy than traditional methods, among which convolutional LSTM achieves better results in most cases as it can capture local time-variant patterns. In addition, we also propose methods to exploit alternative off-market features (such as social media emotions and Baidu Search Index) with DNN models, which are proven beneficial to the price and risk prediction by rendering extra information than only market data.
更多查看译文
关键词
Deep Learning,Convolutional LSTM,Time Series Prediction,Financial Derivatives
AI 理解论文
溯源树
样例
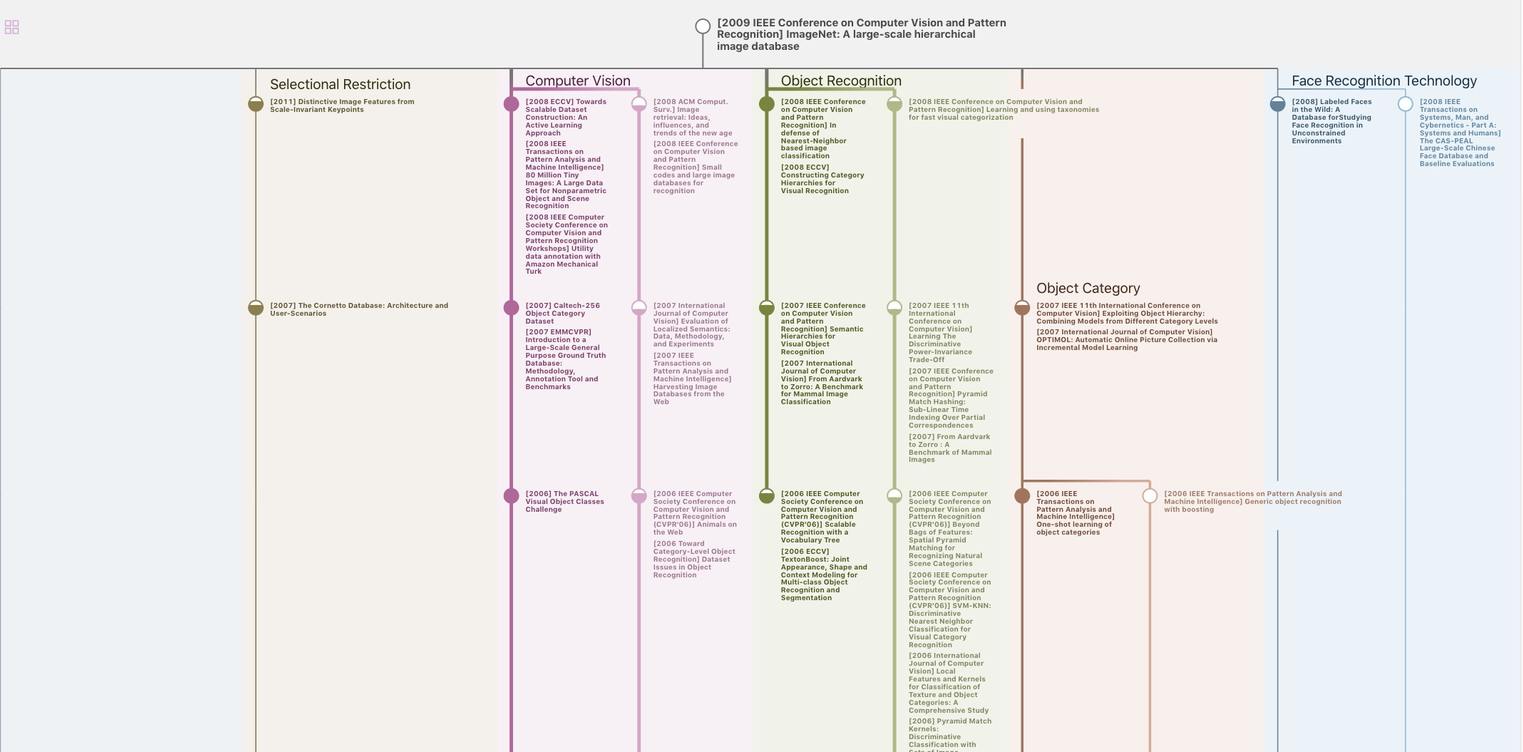
生成溯源树,研究论文发展脉络
Chat Paper
正在生成论文摘要