Fast Characterization of Input-Output Behavior of Non-Charge-Based Logic Devices by Machine Learning
ELECTRONICS(2020)
摘要
Non-charge-based logic devices are promising candidates for the replacement of conventional complementary metal-oxide semiconductors (CMOS) devices. These devices utilize magnetic properties to store or process information making them power efficient. Traditionally, to fully characterize the input-output behavior of these devices a large number of micromagnetic simulations are required, which makes the process computationally expensive. Machine learning techniques have been shown to dramatically decrease the computational requirements of many complex problems. We use state-of-the-art data-efficient machine learning techniques to expedite the characterization of their behavior. Several intelligent sampling strategies are combined with machine learning (binary and multi-class) classification models. These techniques are applied to a magnetic logic device that utilizes direct exchange interaction between two distinct regions containing a bistable canted magnetization configuration. Three classifiers were developed with various adaptive sampling techniques in order to capture the input-output behavior of this device. By adopting an adaptive sampling strategy, it is shown that prediction accuracy can approach that of full grid sampling while using only a small training set of micromagnetic simulations. Comparing model predictions to a grid-based approach on two separate cases, the best performing machine learning model accurately predicts 99.92% of the dense test grid while utilizing only 2.36% of the training data respectively.
更多查看译文
关键词
machine learning,sequential sampling,data-efficient machine learning,magnetic logic devices,classification
AI 理解论文
溯源树
样例
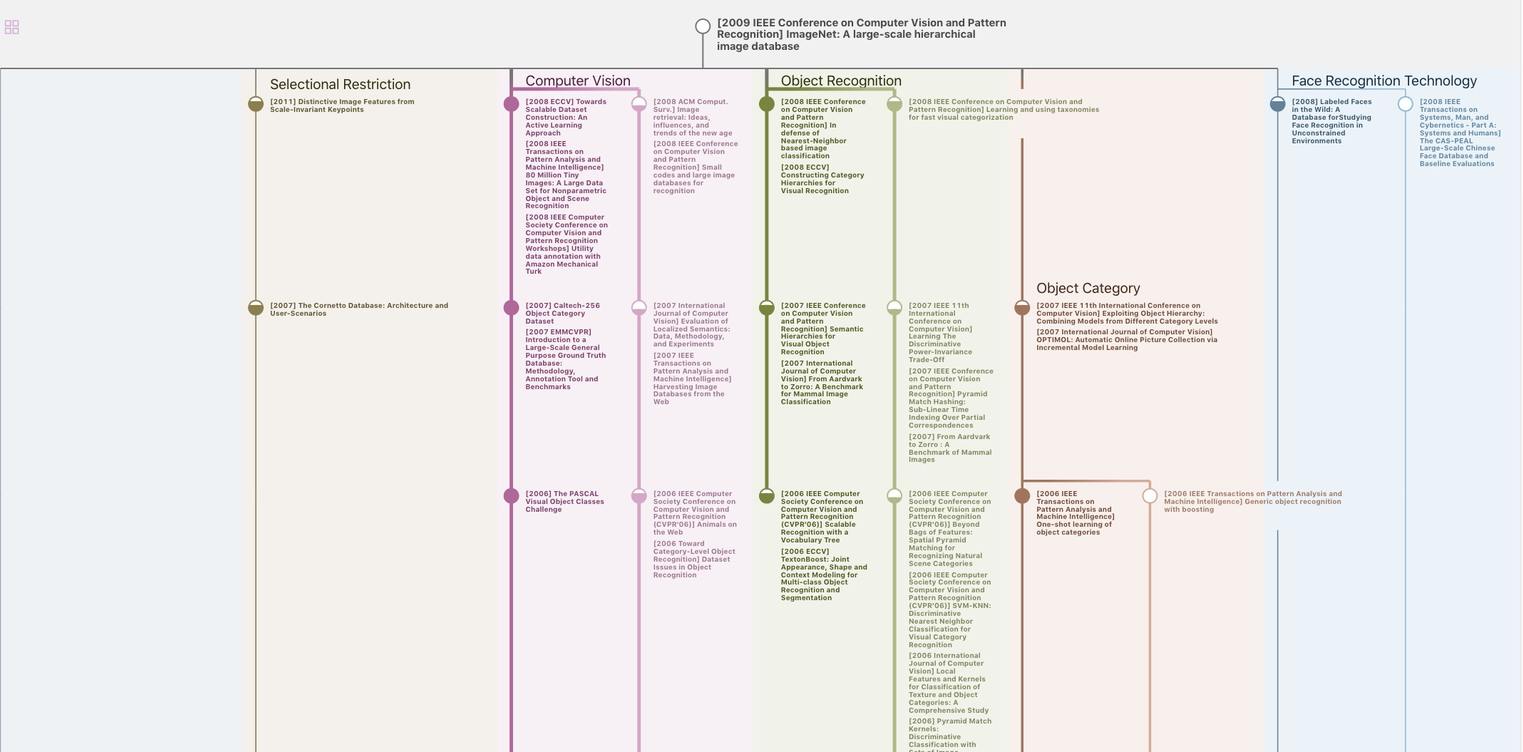
生成溯源树,研究论文发展脉络
Chat Paper
正在生成论文摘要