Learning the Front-End Speech Feature with Raw Waveform for End-to-End Speaker Recognition.
ICCAI(2020)
摘要
State-of-the-art deep neural network-based speaker recognition systems tend to follow the paradigm of speech feature extraction and then the speaker classifier training, namely "divide and conquer" approaches. These methods usually rely on fixed, handcrafted features such as Mel frequency cepstral coefficients (MFCCs) to preprocess the waveform before the classification pipeline. In this paper, inspired by the success and promising work to model a system directly from the raw speech signal for applications such as audio speech recognition, anti-spoofing and emotion recognition, we present an end-to-end speaker recognition system, combining front-end raw waveform feature extractor, back-end speaker embedding classifier and angle-based loss optimizer. Specifically, this means that the proposed frontend raw waveform feature extractor builds on a trainable alternative for MFCCs without modification of the acoustic model. And we will detail the superiority of the raw waveform feature extractor, namely utilizing the time convolution layer to reduce temporal variations aiming to adaptively learn a front-end speech feature representation by supervised training together with the rest of classification model. Our experiments, conducted on CSTR VCTK Corpus dataset, demonstrate that the proposed end-to-end speaker recognition system can achieve state-of-the-art performance compared to baseline models.
更多查看译文
AI 理解论文
溯源树
样例
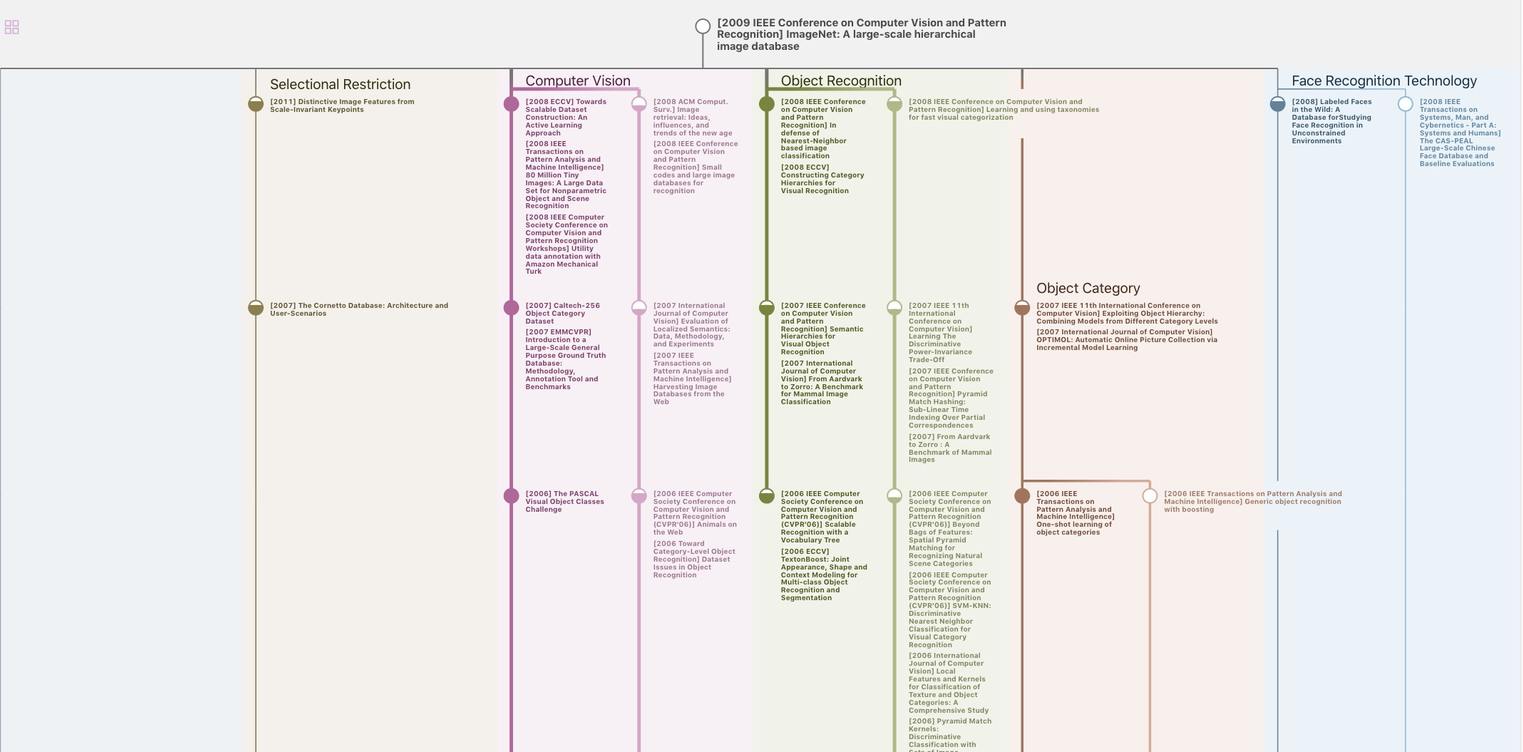
生成溯源树,研究论文发展脉络
Chat Paper
正在生成论文摘要