Using Analysis Corrections To Address Model Error In Atmospheric Forecasts
MONTHLY WEATHER REVIEW(2020)
摘要
This paper illustrates that analysis corrections, when applied as a model tendency term, can be used to improve nonlinear model forecasts and are consistent with the hypothesis that they represent an additive 6-h accumulation of model error. Comparison of mean analysis corrections with observational estimates of bias further illustrates the fidelity with which mean analysis corrections capture the model bias. While most previous implementations have explored the use of analysis corrections to correct forecast biases in short-range forecasts, this is the first implementation of the correction method using both a seasonal mean and random analysis correction for medium-range forecasts (out to 10 days). Overall, the analysis correction-based perturbations are able to improve forecast skill in ensemble and deterministic systems, especially in the first 5 days of the forecast where bias and RMSE in both lower-tropospheric temperature and 500 hPa geo-potential height are significantly improved across all experiments. However, while the method does provide some significant improvement to forecast skill, some degradation in bias can occur at later lead times when the average bias at analysis time trends toward zero over the length of the forecast, leading to an overcorrection by the analysis correction-based additive inflation (ACAI) method. Additionally, it is shown that both the mean and random component of the ACAI perturbations play a role in adjusting the model bias, and that the two components can have a complicated and sometimes nonlinear interaction.
更多查看译文
AI 理解论文
溯源树
样例
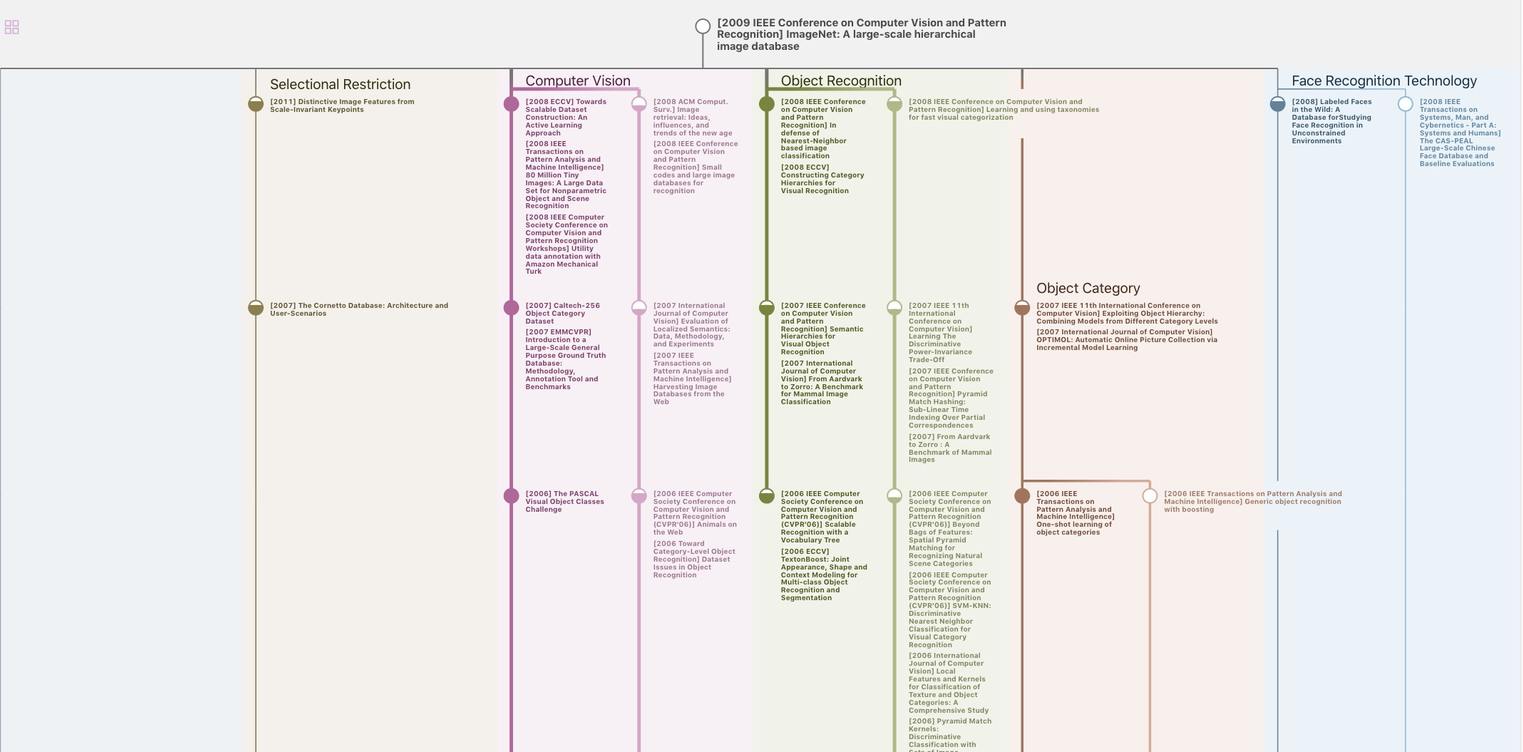
生成溯源树,研究论文发展脉络
Chat Paper
正在生成论文摘要