DEFace: Deep Efficient Face Network for Small Scale Variations
IEEE ACCESS(2020)
摘要
This study proposes a novel face detector called DEFace that focuses on the challenging tasks of face detection to cope with a small size that is under 12 pixels and occlusions due to a mask or human body parts. This study proposed the extended feature pyramid network (FPN) module to detect small faces by expanding the range of P layer, and the network by adding a receptive context module (RCM) after each predicted feature head from the top-down pathway in the FPN architecture to enhance the feature discriminability and the robustness. Based on the FPN principle, the combination between the low- and high-resolutions are beneficial for object detection with different object sizes. Furthermore, with assistance from the RCM, the proposed method can use a broad range of context information especially for small faces. To evaluate the performance of the proposed method, various public face datasets are used such as the WIDER Face dataset, the face detection dataset and benchmark (FDDB), and the masked faces (MAFA) dataset, which consist of challenging samples such as small face regions and occlusions by hair or other people. The results indicate that DEFace can detect the face region more accurately in comparison to the other state-of-the-art methods while maintaining the processing time.
更多查看译文
关键词
Face,Face detection,Feature extraction,Detectors,Object detection,Computational modeling,Semantics,Small face detection,context module,single-shot convolutional module
AI 理解论文
溯源树
样例
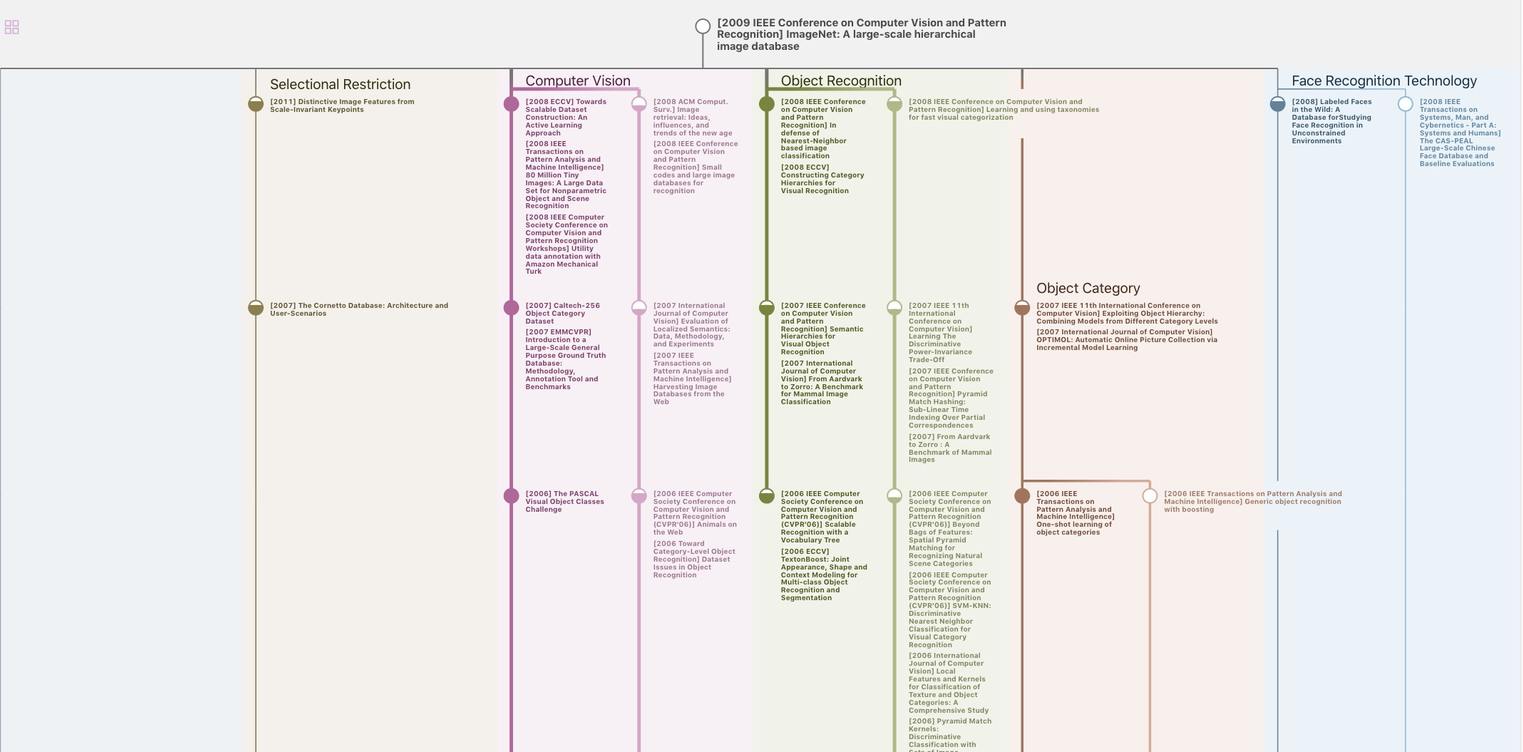
生成溯源树,研究论文发展脉络
Chat Paper
正在生成论文摘要