Requet: Real-Time QoE Metric Detection for Encrypted YouTube Traffic
ACM Transactions on Multimedia Computing, Communications, and Applications(2020)
摘要
AbstractAs video traffic dominates the Internet, it is important for operators to detect video quality of experience (QoE) to ensure adequate support for video traffic. With wide deployment of end-to-end encryption, traditional deep packet inspection--based traffic monitoring approaches are becoming ineffective. This poses a challenge for network operators to monitor user QoE and improve upon their experience. To resolve this issue, we develop and present a system for REal-time QUality of experience metric detection for Encrypted Traffic—Requet—which is suitable for network middlebox deployment. Requet uses a detection algorithm that we develop to identify video and audio chunks from the IP headers of encrypted traffic. Features extracted from the chunk statistics are used as input to a machine learning algorithm to predict QoE metrics, specifically buffer warning (low buffer, high buffer), video state (buffer increase, buffer decay, steady, stall), and video resolution. We collect a large YouTube dataset consisting of diverse video assets delivered over various WiFi and LTE network conditions to evaluate the performance. We compare Requet with a baseline system based on previous work and show that Requet outperforms the baseline system in accuracy of predicting buffer low warning, video state, and video resolution by 1.12×, 1.53×, and 3.14×, respectively.
更多查看译文
关键词
Machine learning, HTTP adaptive streaming
AI 理解论文
溯源树
样例
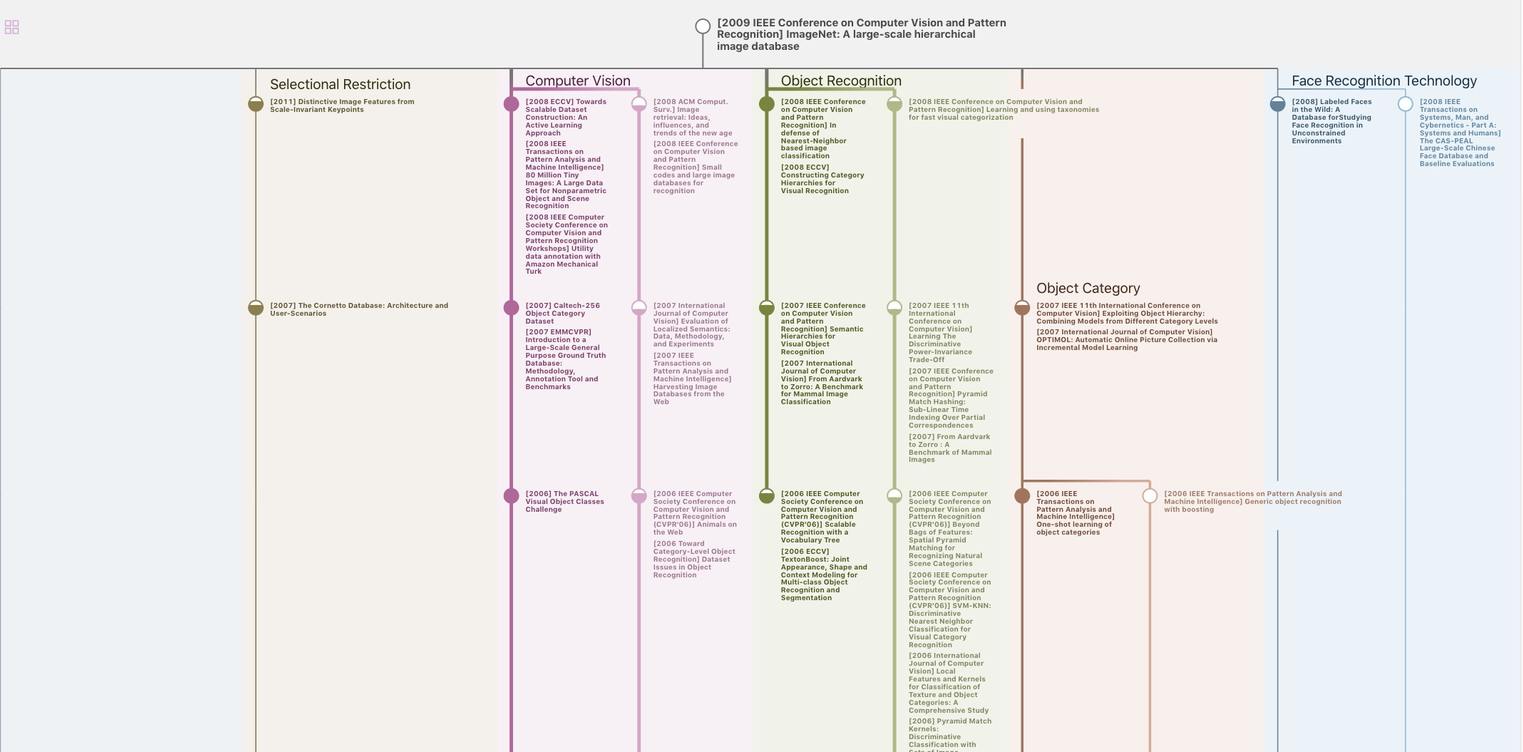
生成溯源树,研究论文发展脉络
Chat Paper
正在生成论文摘要