A Targeted Estimation of Distribution Algorithm Compared to Traditional Methods in Feature Selection
Studies in Computational Intelligence(2016)
摘要
The Targeted Estimation of Distribution Algorithm (TEDA) introduces into an EDA/GA hybrid framework a 'Targeting' process, whereby the number of active genes, or 'control points', in a solution is driven in an optimal direction. For larger feature selection problems with over a thousand features, traditional methods such as forward and backward selection are inefficient. Traditional EAs may perform better but are slow to optimize if a problem is sufficiently noisy that most large solutions are equally ineffective and it is only when much smaller solutions are discovered that effective optimization may begin. By using targeting, TEDA is able to drive down the feature set size quickly and so speeds up this process. This approach was tested on feature selection problems with between 500 and 20,000 features using all of these approaches and it was confirmed that TEDA finds effective solutions significantly faster than the other approaches.
更多查看译文
关键词
Estimation of distribution algorithms,Feature selection,Genetic algorithms,Hybrid algorithms
AI 理解论文
溯源树
样例
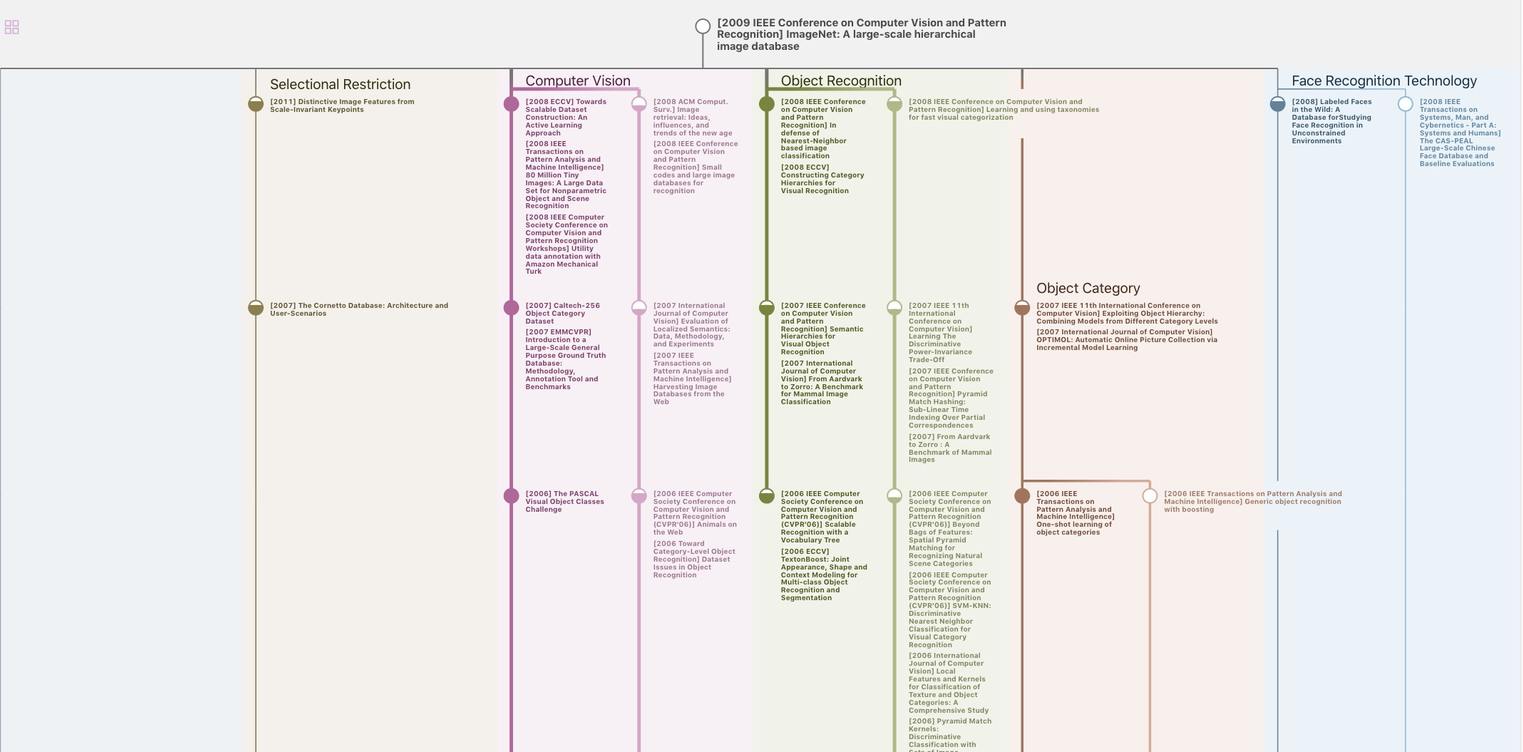
生成溯源树,研究论文发展脉络
Chat Paper
正在生成论文摘要