Learning Sign-Constrained Support Vector Machines
arxiv(2020)
摘要
Domain knowledge is useful to improve the generalization performance of learning machines. Sign constraints are a handy representation to combine domain knowledge with learning machine. In this paper, we consider constraining the signs of the weight coefficients in learning the linear support vector machine, and develop two optimization algorithms for minimizing the empirical risk under the sign constraints. One of the two algorithms is based on the projected gradient method, in which each iteration of the projected gradient method takes O(nd) computational cost and the sublinear convergence of the objective error is guaranteed. The second algorithm is based on the Frank-Wolfe method that also converges sublinearly and possesses a clear termination criterion. We show that each iteration of the Frank-Wolfe also requires O(nd) cost. Furthermore, we derive the explicit expression for the minimal iteration number to ensure an 6-accurate solution by analyzing the curvature of the objective function. Finally, we empirically demonstrate that the sign constraints are a promising technique when similarities to the training examples compose the feature vector.
更多查看译文
关键词
learning machine,sign constraints,domain knowledge,linear support vector machine,optimization algorithms,sublinear convergence,Frank-Wolfe method,minimal iteration number,feature vector,generalization performance,sign-constrained support vector machines,projected gradient method,O(nd) computational cost
AI 理解论文
溯源树
样例
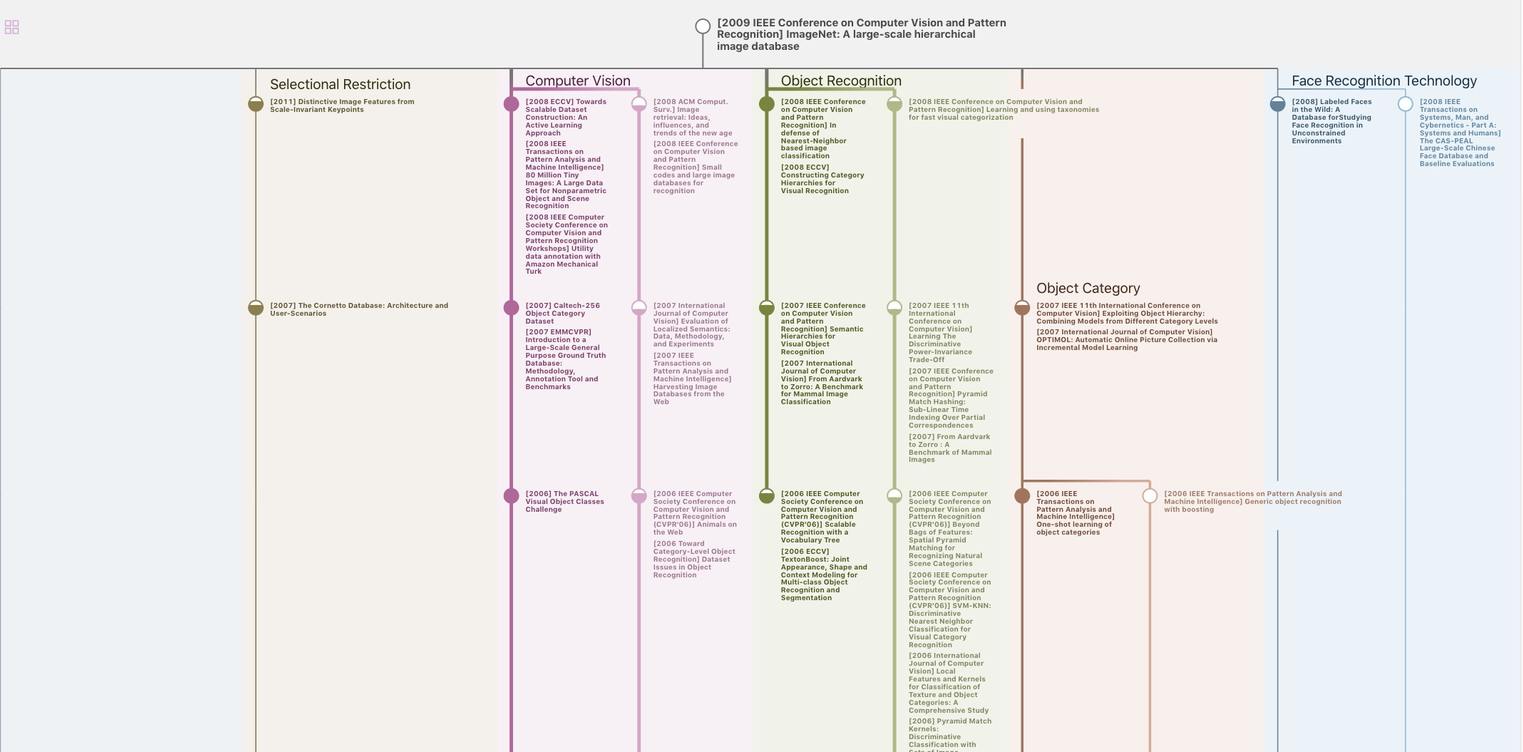
生成溯源树,研究论文发展脉络
Chat Paper
正在生成论文摘要