Stochastic Action Prediction for Imitation Learning
arXiv (Cornell University)(2020)
Abstract
Imitation learning is a data-driven approach to acquiring skills that relies on expert demonstrations to learn a policy that maps observations to actions. When performing demonstrations, experts are not always consistent and might accomplish the same task in slightly different ways. In this paper, we demonstrate inherent stochasticity in demonstrations collected for tasks including line following with a remote-controlled car and manipulation tasks including reaching, pushing, and picking and placing an object. We model stochasticity in the data distribution using autoregressive action generation, generative adversarial nets, and variational prediction and compare the performance of these approaches. We find that accounting for stochasticity in the expert data leads to substantial improvement in the success rate of task completion.
MoreTranslated text
Key words
imitation learning,prediction,stochastic
AI Read Science
Must-Reading Tree
Example
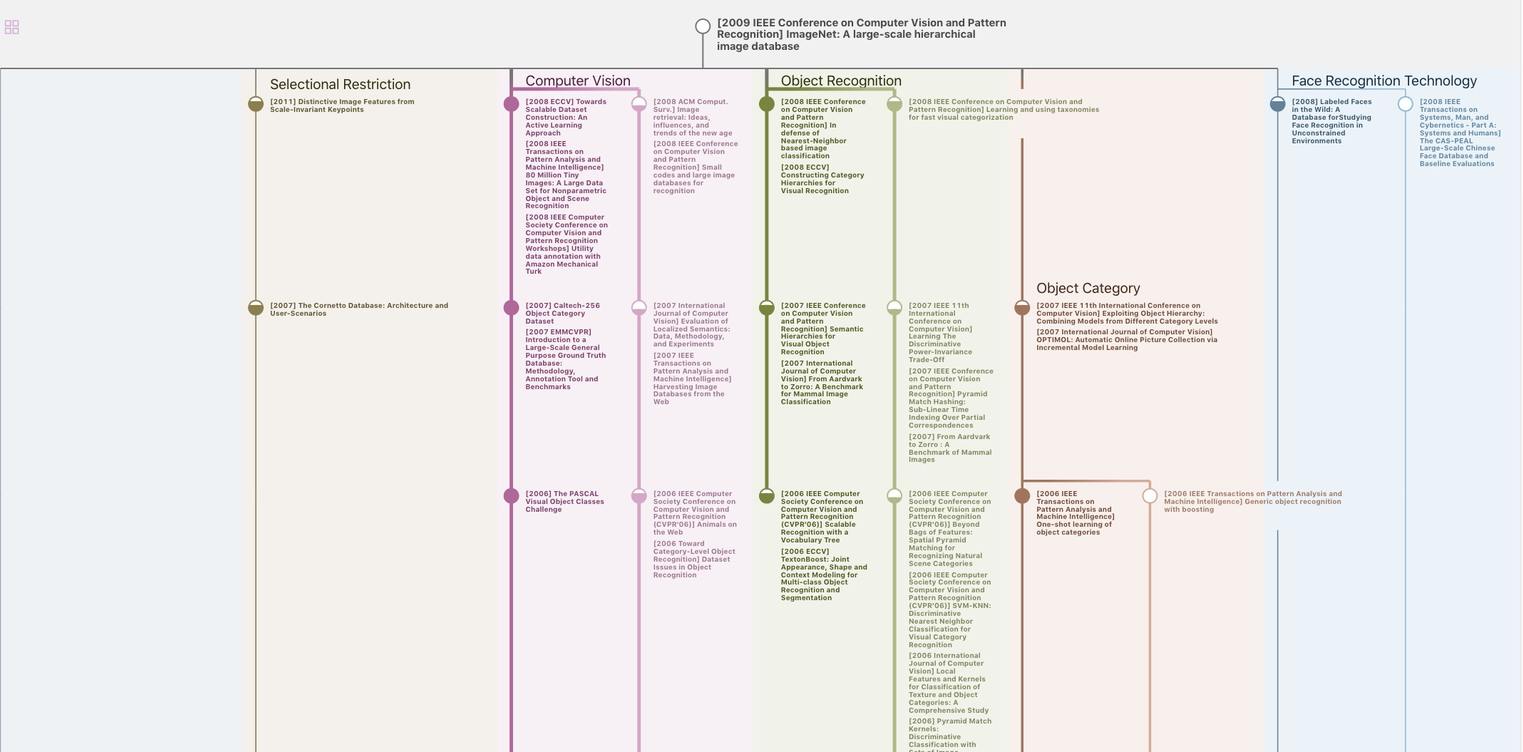
Generate MRT to find the research sequence of this paper
Chat Paper
Summary is being generated by the instructions you defined