Exploring the Space Between Smoothed and Non-Smooth Total Variation for 3-D Iterative CT Reconstruction
Proceedings of SPIE(2019)
摘要
Because total variation (TV) is non-differentiable, iterative reconstruction using a TV penalty comes with technical difficulties. To avoid these, it is popular to use a smooth approximation of TV instead, which is defined using a single parameter, herein called delta, with the convention that the approximation is better when delta is smaller. To our knowledge, it is not known how well image reconstruction with this approximation can approach a converged non-smooth TV-regularized result. In this work, we study this particular question in the context of X-ray computed tomography (CT). Experimental results are reported with real CT data of a head phantom and supplemented with a theoretical analysis. To address our question, we make proficient use of a generalized iterative soft-thresholding algorithm that allows us to handle TV and its smooth approximation in the same framework. Our results support the following conclusions. First, images reconstructed using the smooth approximation of TV appears to smoothly converge towards the TV result as delta tends to zero. Second, the value of delta does not need to be overly small to obtain a result that is essentially equivalent to TV, implying that numerical instabilities can be avoided. Last, though it is smooth, the convergence with delta is not particularly fast, as the mean absolute pixel difference decreases only as root delta in our experiments. Altogether, we conclude that the approximation is a theoretically valid way to approximate the non-smooth TV penalty for CT, opening the door to safe utilization of a wide variety of optimization algorithms.
更多查看译文
关键词
Computed tomography,iterative reconstruction,total variation,Huber function,GISTA
AI 理解论文
溯源树
样例
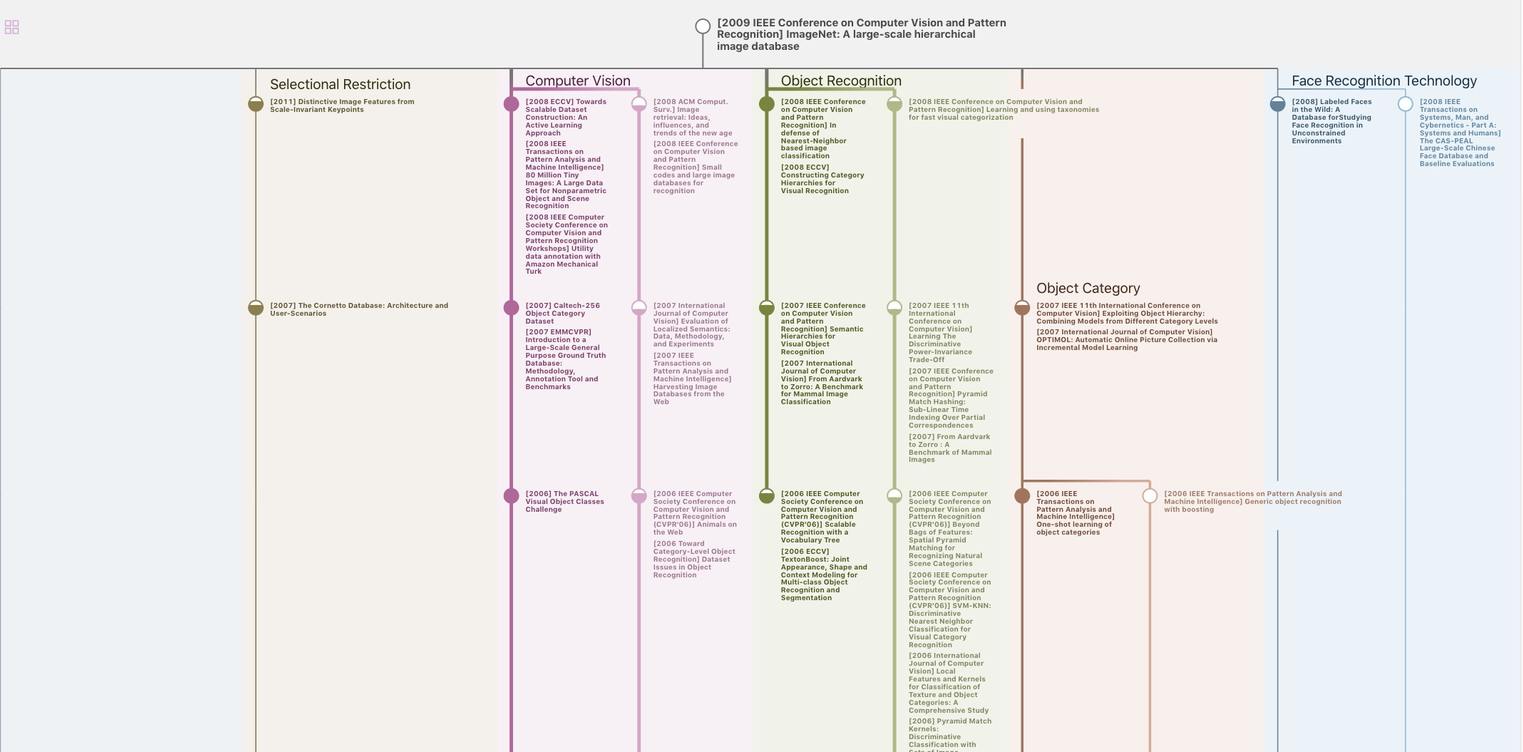
生成溯源树,研究论文发展脉络
Chat Paper
正在生成论文摘要