Using Dynamic Bayesian Networks for Incorporating Nontraditional Data Sources in Public Health Surveillance
Lecture Notes in Social Networks(2017)
摘要
The estimation of disease prevalence based on public health surveillance data requires the accurate identification of cases from limited information (e.g., diagnostic codes). These data sources typically consist of routinely collected records of population healthcare utilization, such as administrative and clinical data, that specifies diagnostic codes or terms for each encounter. These data sources include, for example, emergency department visits, pharmaceutical (drug) dispensations, and laboratory test orders. The case definitions depend on the data source and are typically based on the presence of diagnostic codes or key words in a prespecified time frame. Each data source will result in a certain degree of misclassification bias when estimating prevalence. Inaccuracies can occur at each stage from the time the disease process is initiated to the stage at which diagnostic codes are entered into the database. Indeed, when relying on these data sources, asymptomatic cases will be missed, as well as those not seeking health care. Even patients that seek care may be inaccurately diagnosed or the diagnostic code that is entered in the system may not represent the diagnosis or may not be a code or key word used in the definition. In addition to misclassification bias, these data sources are not usually available in a timely manner. Timeliness is an important factor for prevalence estimation in certain contexts such as the prevalence of infectious diseases during an epidemic. For instance, in an influenza pandemic, such estimates must be obtained within days. In recent years, several nonclinical and nontraditional data sources have been introduced to public health surveillance with the potential to provide more timely signals of changing prevalence trends. Ideally, combining the new and traditional data sources, there is greater potential to overcome bias and provide more timely signals. However, building a construct capable of incorporating data from these various sources in a coherent manner is not trivial. In this research, we consider the case of the 2009-2010 H1N1 pandemic as the context of interest and we use media reports of deaths from H1N1 on the web as a nontraditional data source. We propose to use dynamic Bayesian networks from the class of probabilistic graphical models in order to combine this new data source with traditional ones through exploration of the possible probabilistic relationships between these data streams. This is an initial step toward building a framework that can potentially support aggregation of heterogeneous data for a real-time estimation of disease prevalence. Our preliminary results show that the proposed model can be used in accurate prediction of short-term future counts of the data sources. This is particularly useful in timely prediction of epidemic changes over a defined population.
更多查看译文
关键词
Public health,Surveillance systems,Probabilistic models,Nontraditional data sources,Dynamic Bayesian networks
AI 理解论文
溯源树
样例
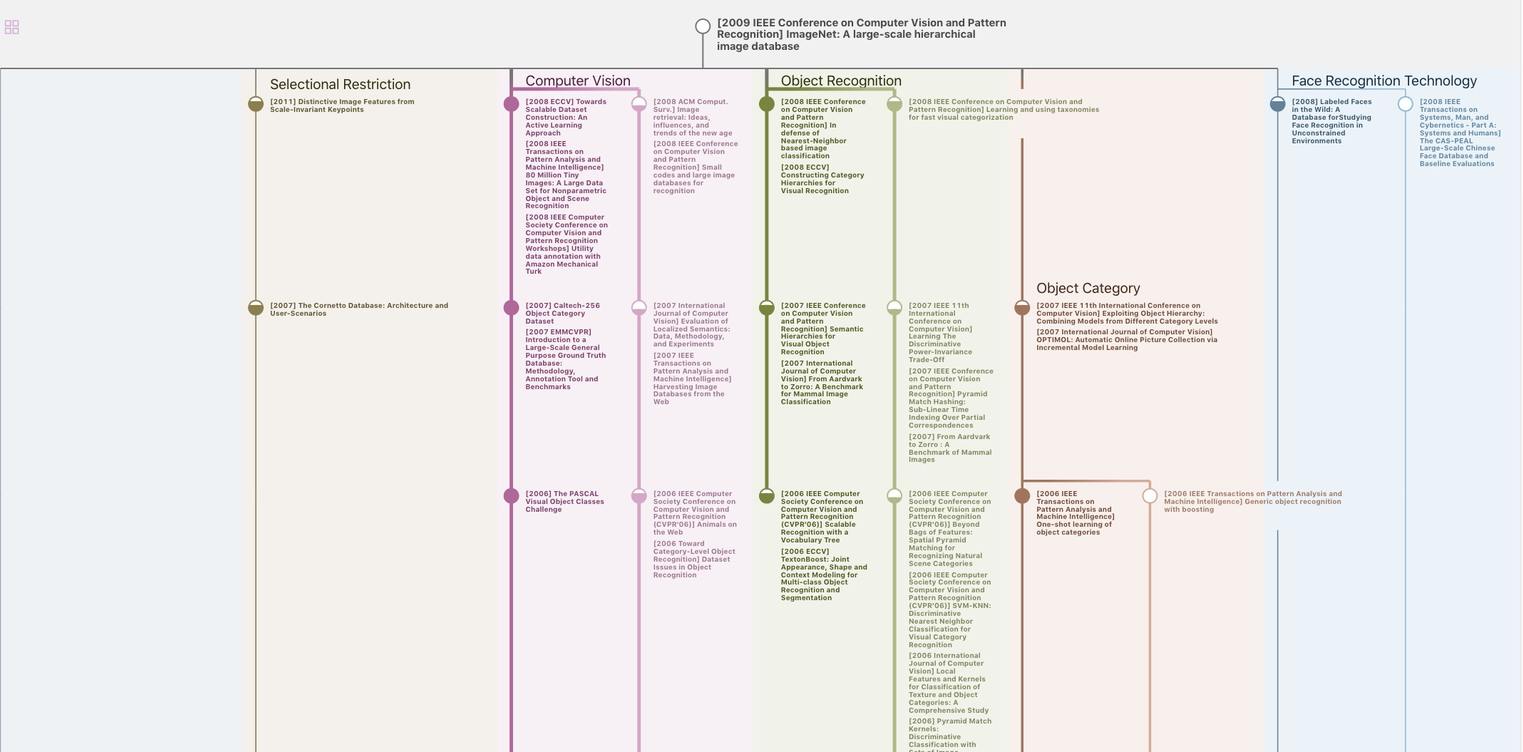
生成溯源树,研究论文发展脉络
Chat Paper
正在生成论文摘要