Optimizing Deeper Transformers on Small Datasets: An Application on Text-to-SQL Semantic Parsing
arxiv(2020)
摘要
Due to the common belief that training deep transformers from scratch requires large datasets, people usually only use shallow and simple additional layers on top of pre-trained models during fine-tuning on small datasets. We provide evidence that this does not always need to be the case: with proper initialization and training techniques, the benefits of very deep transformers are shown to carry over to hard structural prediction tasks, even using small datasets. In particular, we successfully train 48 layers of transformers for a semantic parsing task. These comprise 24 fine-tuned transformer layers from pre-trained RoBERTa and 24 relation-aware transformer layers trained from scratch. With fewer training steps and no task-specific pre-training, we obtain the state of the art performance on the challenging cross-domain Text-to-SQL semantic parsing benchmark Spider. We achieve this by deriving a novel Data dependent Transformer Fixed-update initialization scheme (DT-Fixup), inspired by the prior T-Fixup work. Further error analysis demonstrates that increasing the depth of the transformer model can help improve generalization on the cases requiring reasoning and structural understanding.
更多查看译文
关键词
small datasets,deeper transformers,text-to-sql
AI 理解论文
溯源树
样例
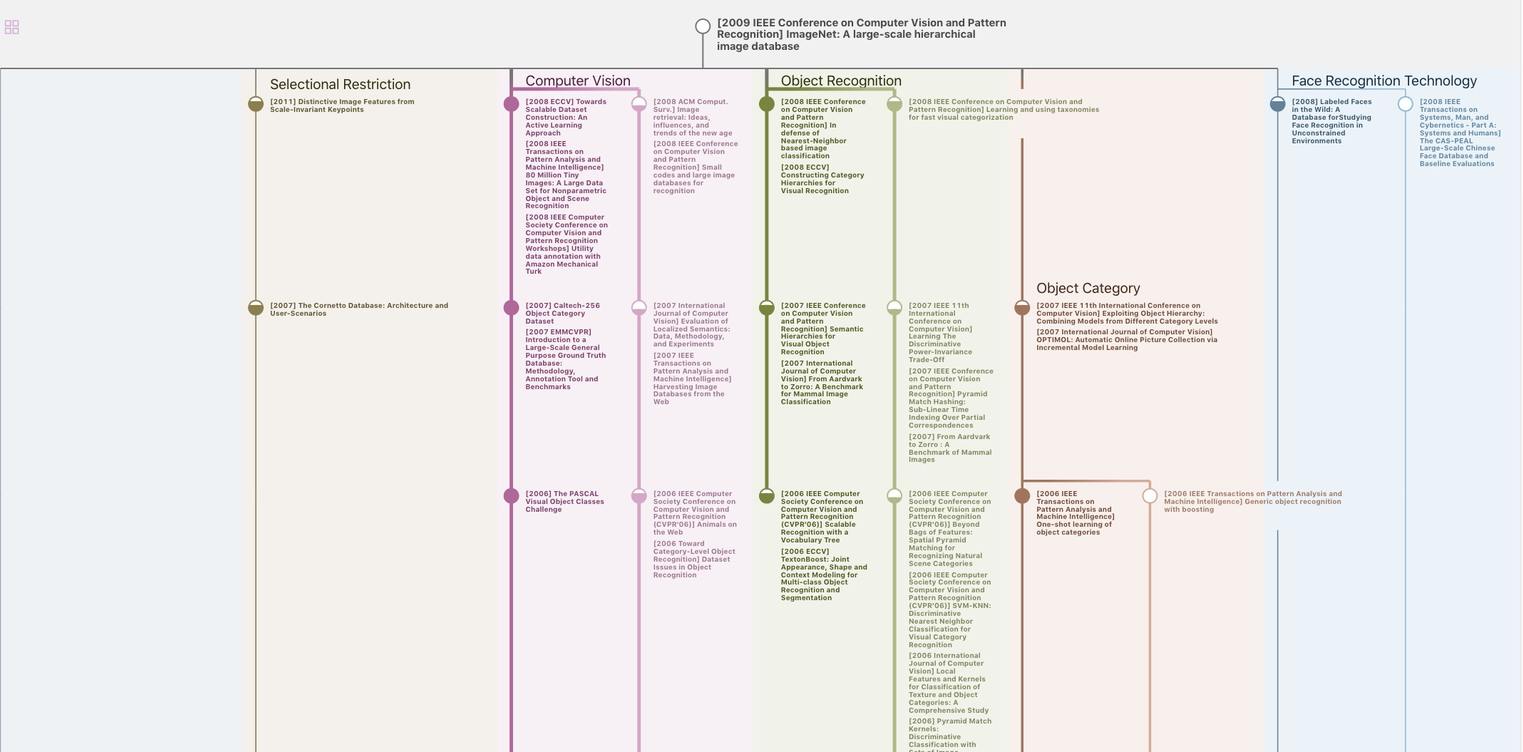
生成溯源树,研究论文发展脉络
Chat Paper
正在生成论文摘要